SMART FACTORY, SUPPLY CHAIN, SUSTAINABILITY
Building accurate decision-support systems with Causal AI
Parabole AI-powered Causal inference technology enables data scientists to build accurate decision-support systems that estimate the effect of interventions under real-world data constraints, and imperfect causal knowledge.
Why Causal AI
“Correlation does not imply causation”
Decisions made at the enterprise level can have critical downstream consequences, therefor the need exists to not only understand why a system makes a decision, but also to understand the impacts of that decision, and how decision-making can be optimized to achieve more desirable outcomes.
The right question to ask is “Does Supervised Machine Learning and other traditional approaches help discover the cause and effect of a decision. Plainly, the answer is NO. The challenge therefore in the traditional approach is that interventions change the statistical distribution of the variable in observed data and invalidate the assumptions on which the training models were built. The resultant prediction is therefore unreliable. Furthermore, answering counterfactual questions can be even more difficult than answering interventional questions using traditional approaches. Furthermore, answering counterfactual questions can be even more difficult than answering interventional questions.
How Causality can help address these challenges Supervised ML approaches cannot answer Causal questions through a singular dependence on trained data sets and traditional ML algorithms. Here, Causal Inferencing helps bridge the gap between predictions and decision-making and allows AI practitioners to simulate an intervention and infer causality from available data sets.
Benefits of Causal AI
Causal AI has obvious benefits when compared to statistical and traditional supervised learning approaches
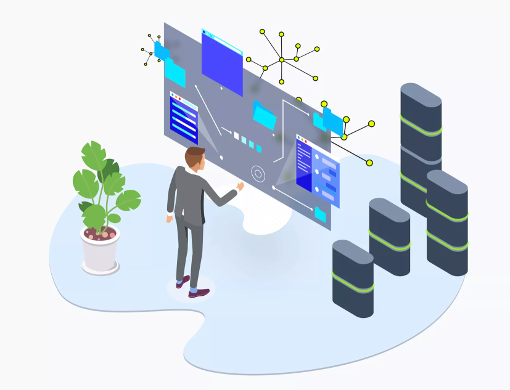
Simulated experiments and causal inference
Experiments predict how models will behave under intervention, and quantify how much a cause (e.g. new raw material introduction) affects an effect (e.g. product quality) without carrying out the intervention, all in a fraction of time and cost.
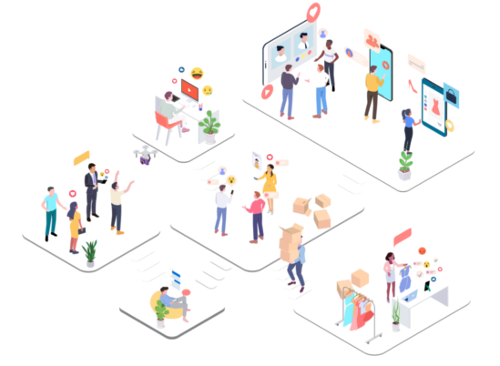
Decisions, Interventions and Counterfactuals
Bridges the gap between prediction and decision-making and allows scientists to simulate an intervention and infer causality. Causal experiments further enable scientists to predict how the models will behave under intervention, without needing to carry out the intervention in the physical world. Additionally. Causal models allow operators to respond to previously unseen situations and enable the solution to plan for any unforeseen counterfactual situations.
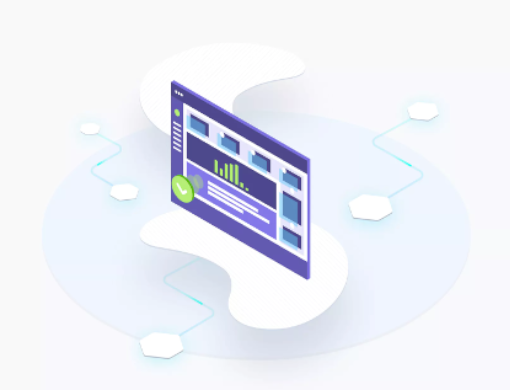
ML made more robust, explainable, and valuable
ML models lack robustness due to overfitting. Causal modeling enhances robustness by representing invariant causal relationships between predictors and the predicted outcome. For example, if collecting data on ice cream sales and sunglasses sales during a calendar year, a statistical correlation mechanism might suggest ice cream sales and sunglass sales as originating from a shared cause, summer heat; and thus, not spurious at all. However, causation brings out that sales of ice cream are high during warm temperature months whereas sunglass sales are higher during high UV months which goes beyond summer months capturing the causal invariance.
How can Parabole.ai help you
Our platform-centric approach helps data scientists to build use case-specific causal models for solving complex challenges and apply them to build bespoke solutions
Featured Solutions
Software for a broad range of industries
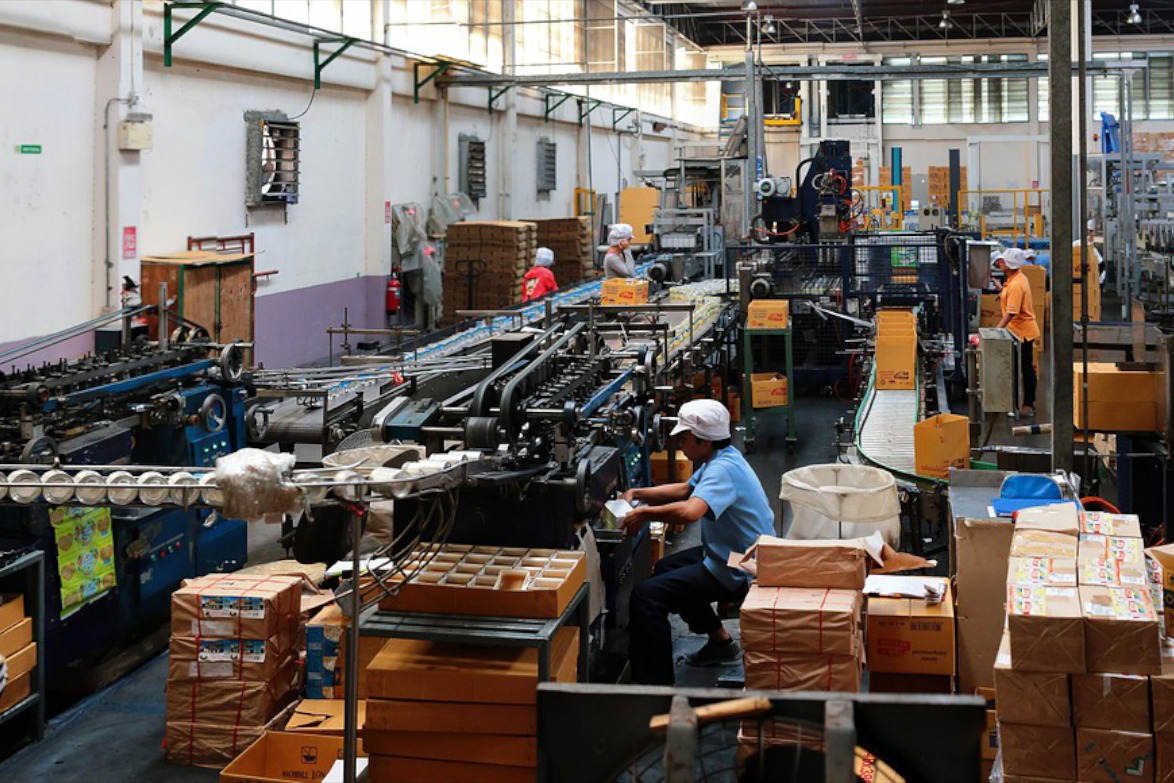
An Engineering major uses Parabole to smartly design and assemble products
Learn how Causal AI helped product designers to contextually search product information faster to aid new product design.
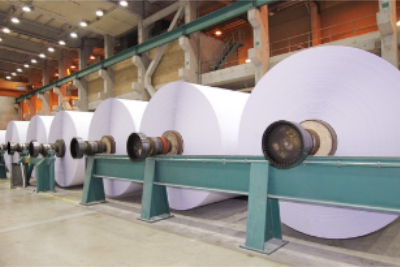
Paper manufacturer uses Parabole to identify the alternate raw material sources and product mix to achieve consistent quality metrics
Learn how Causal AI helped procurement managers source alternate raw materials from new suppliers in the changing supply chain environment
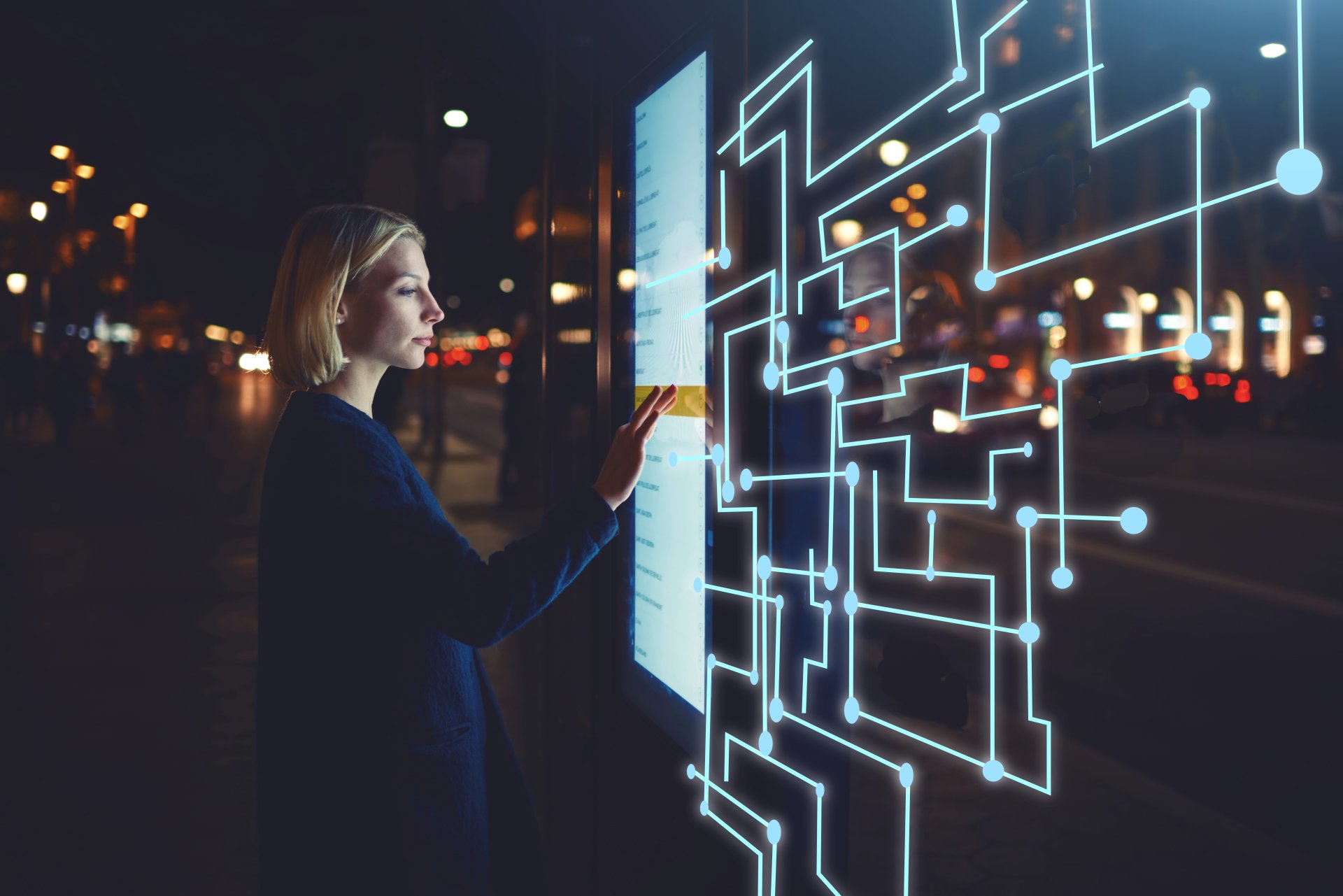
Consumer product company uses Parabole to automate touchless order processing
Learn how Causal AI helped build an intelligent order fulfillment and optimization solution for enhancing overall order fulfillment and customer experience.
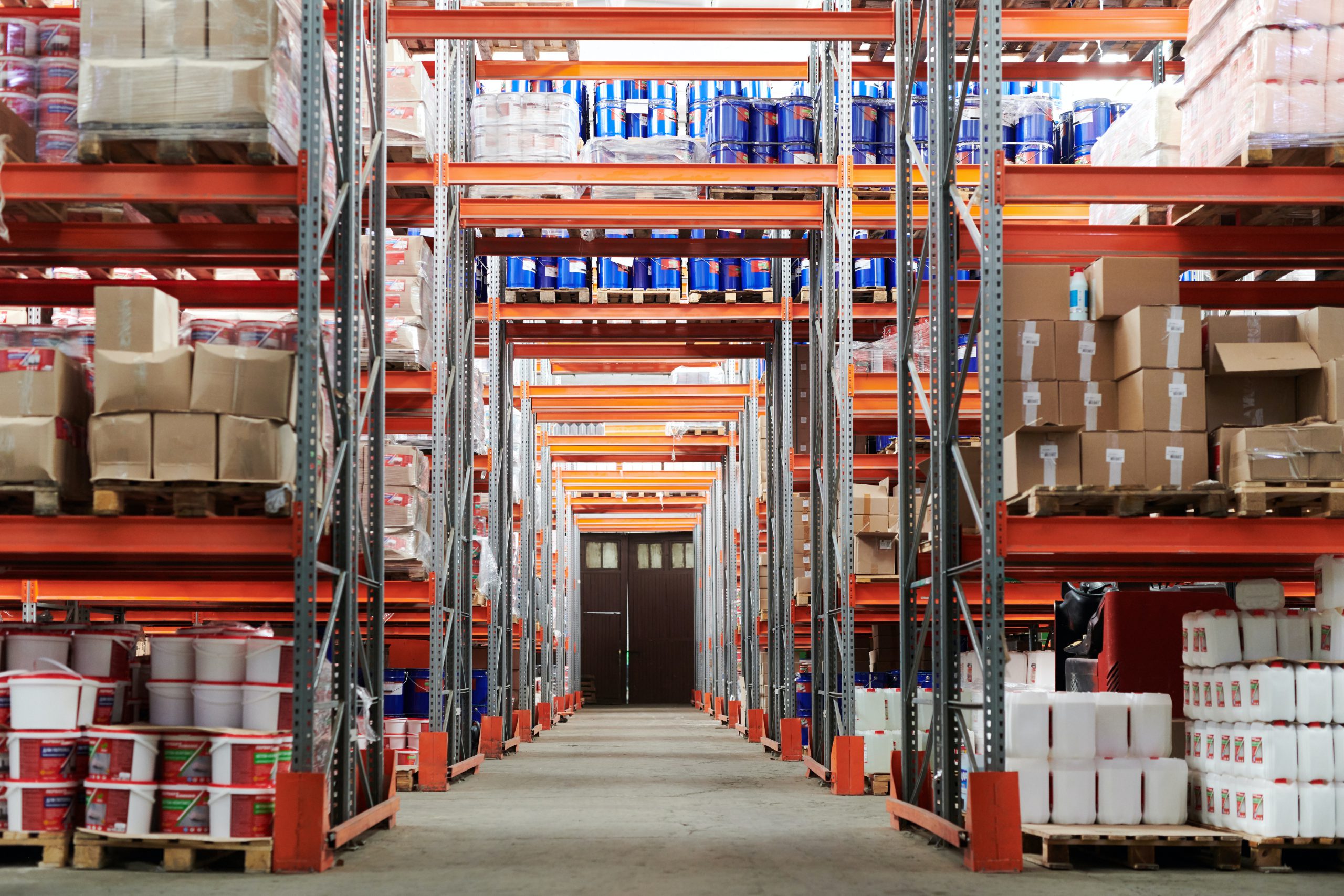
An asset management company uses Parabole to generate alpha and monitor ESG-related risks in real time.
Learn how Causal AI helped build AI models trained on SASB to detect ESG signals from company disclosures and news reports.
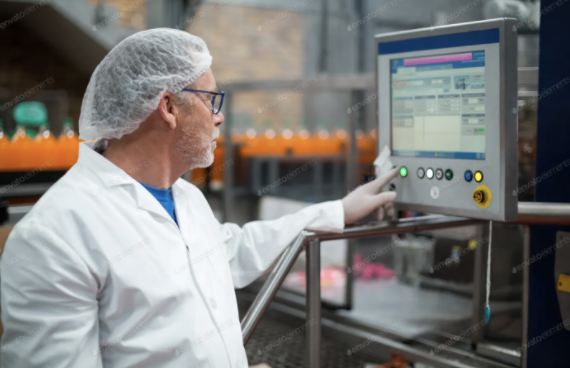
Consumer product company uses Parabole to discover metadata from unstructured information and an build enterprise business glossary.
Learn how Causal AI helped build a semantically connected data catalog.