TRAIN

A platform, uniquely designed to automate AI modeling from complex industrial data
How it works
TRAIN provides fully automated modeling and teaching capabilities, designed to thrive in an imperfect data
world without requiring any manual tagging, labeling or ontology efforts










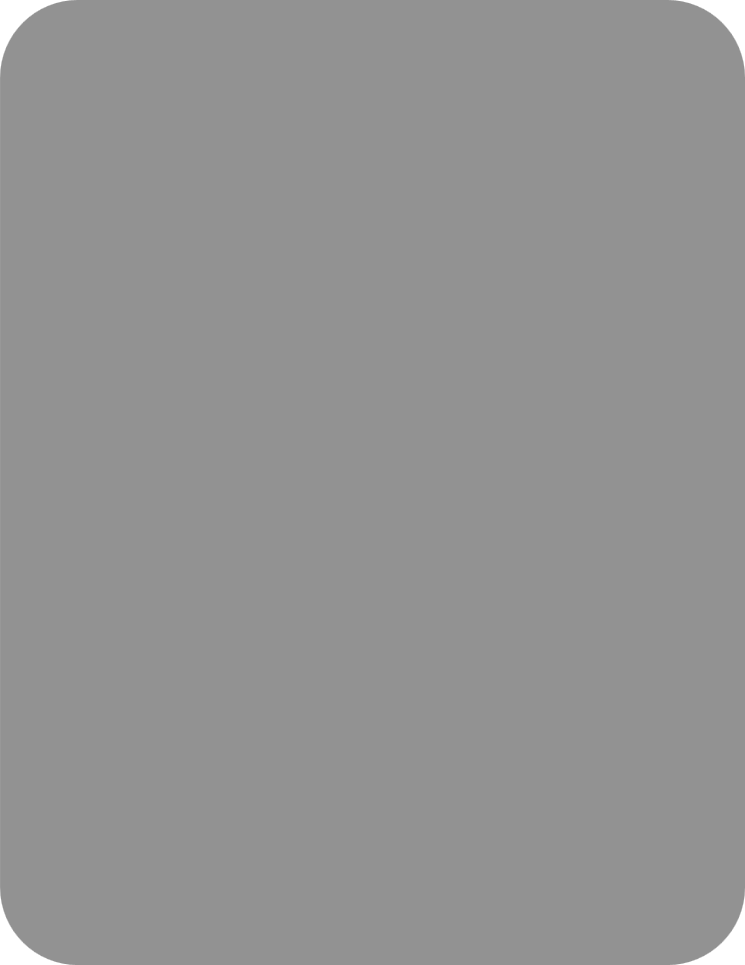
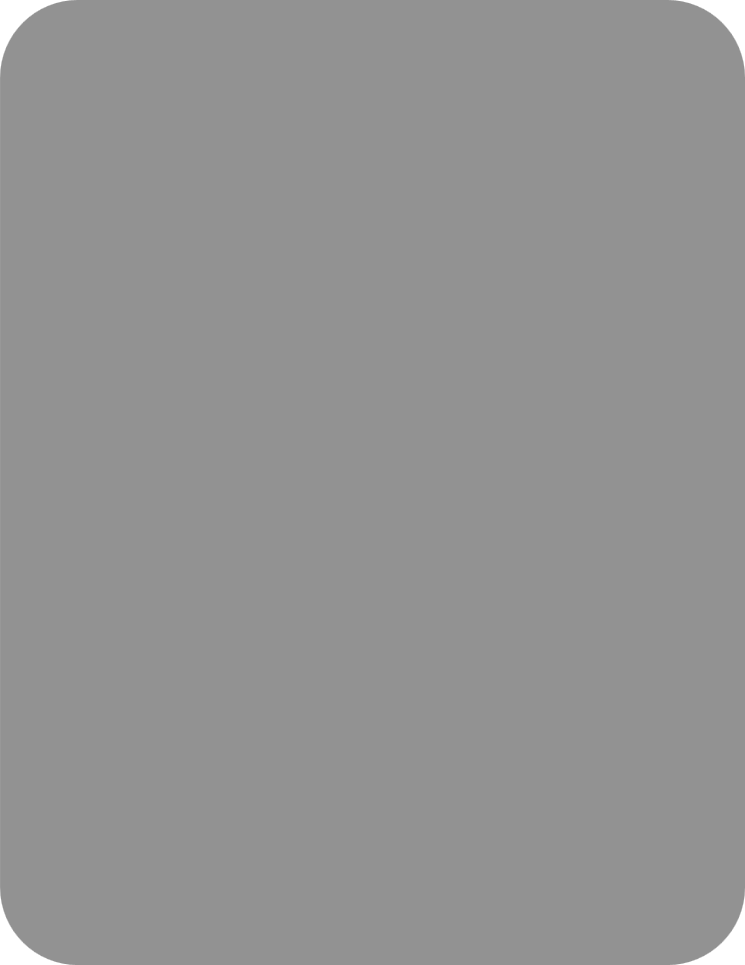
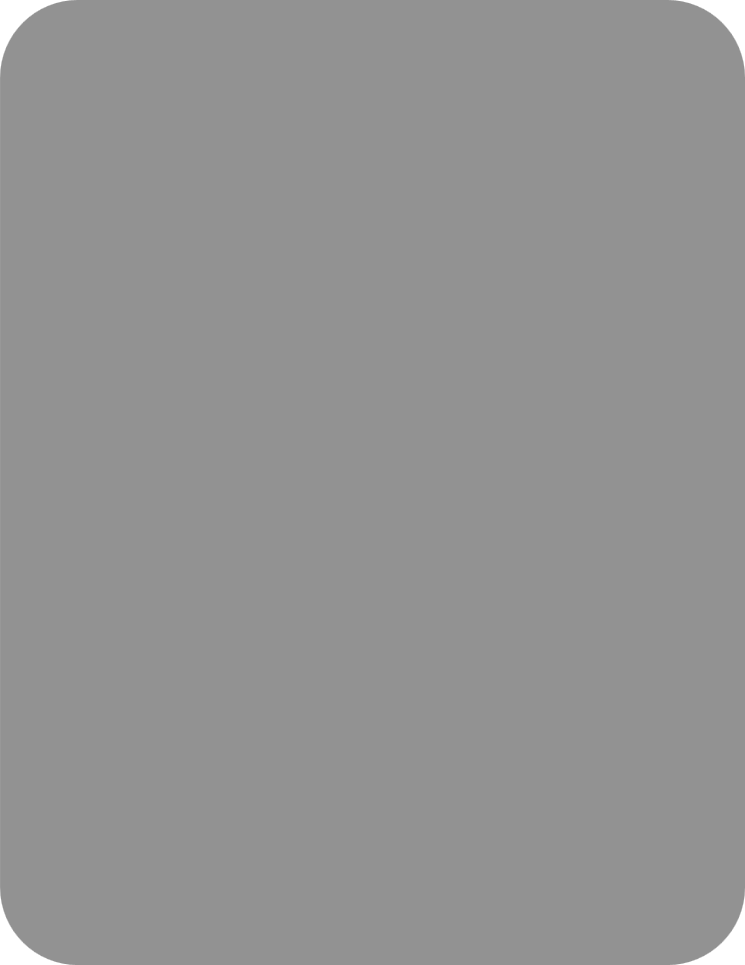
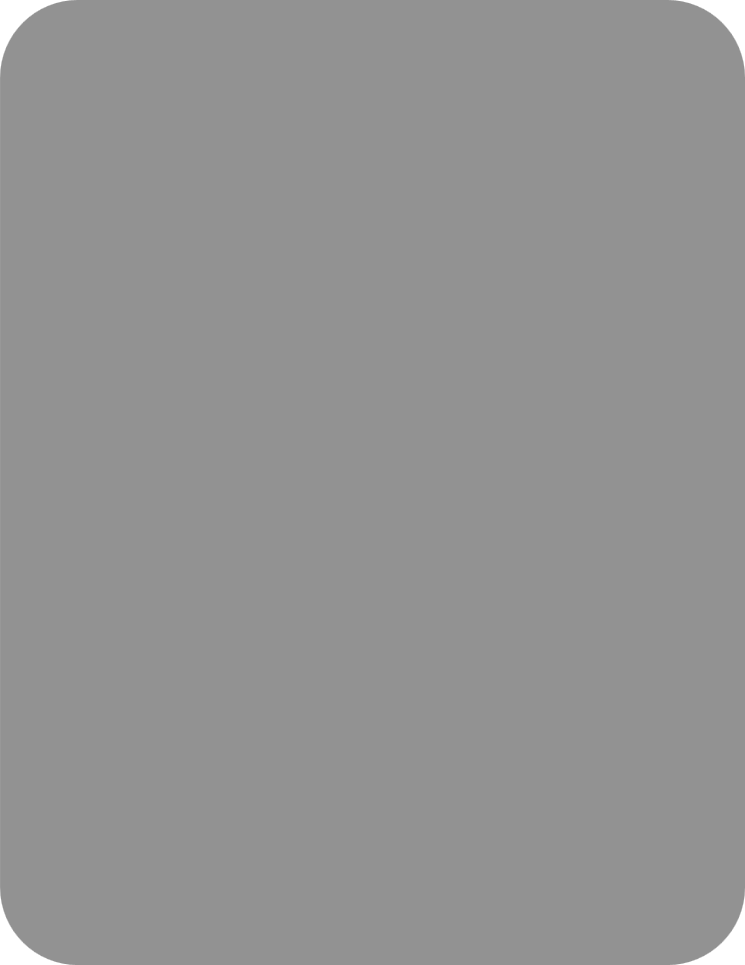
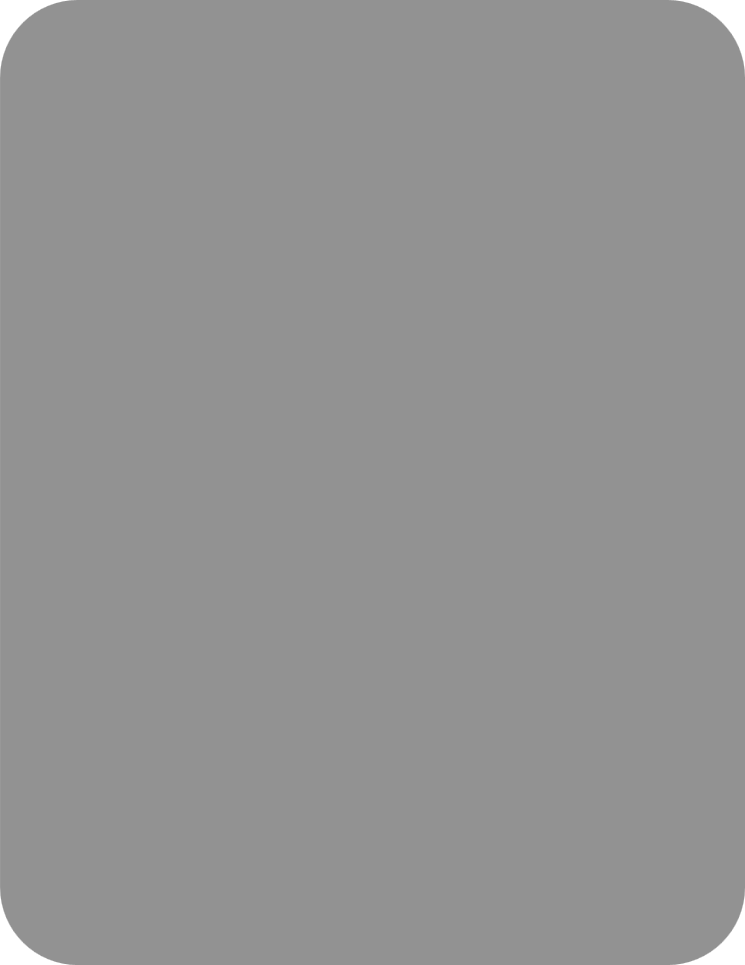
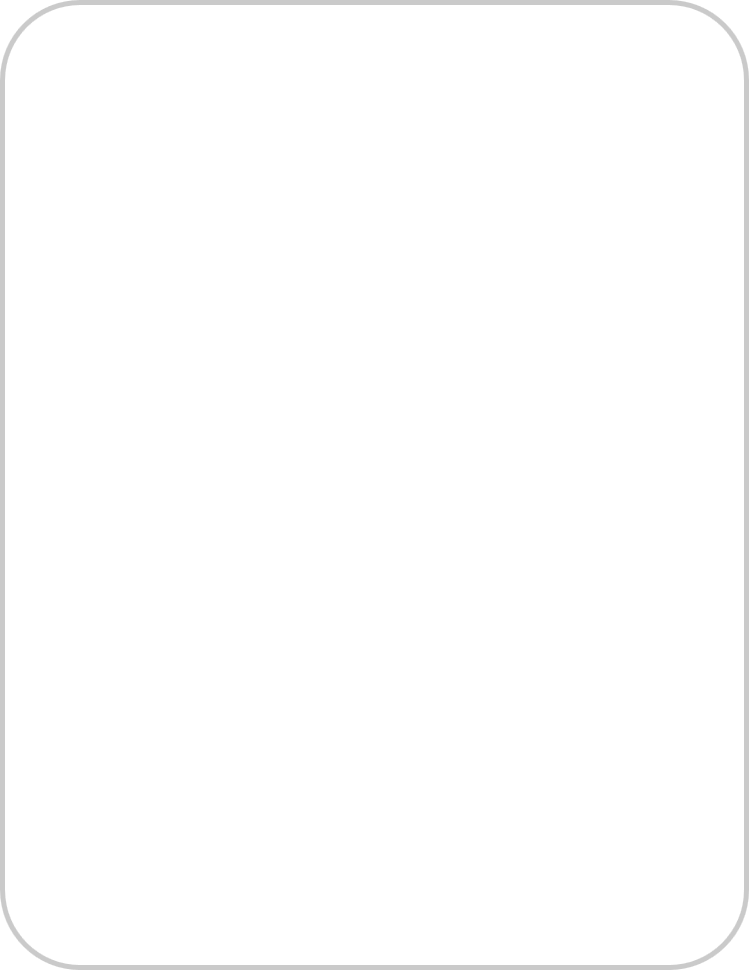
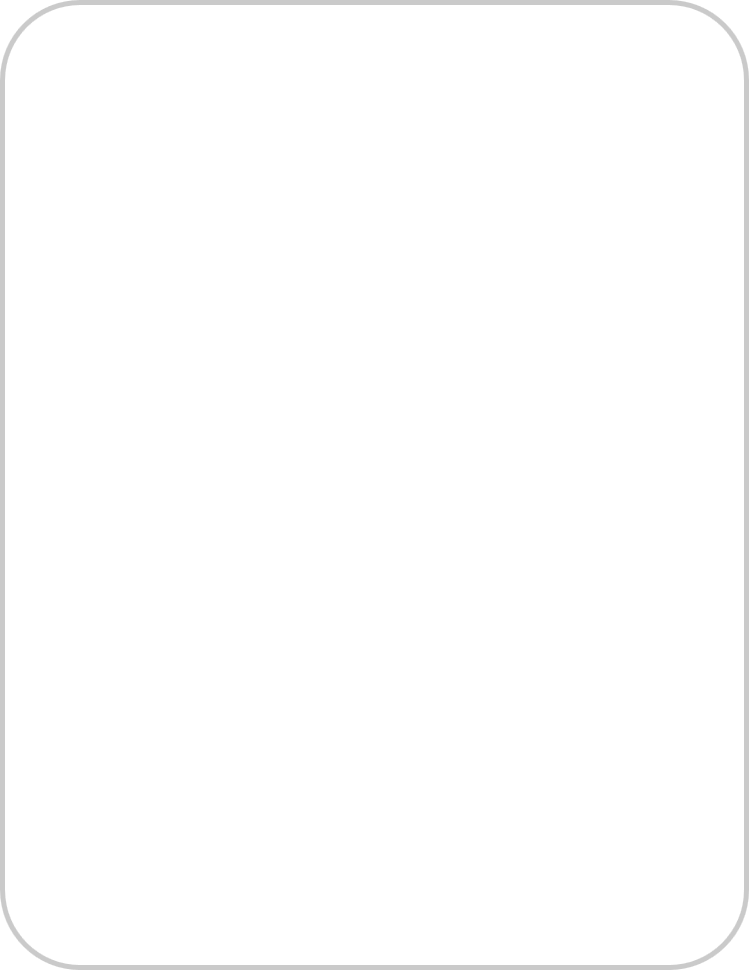
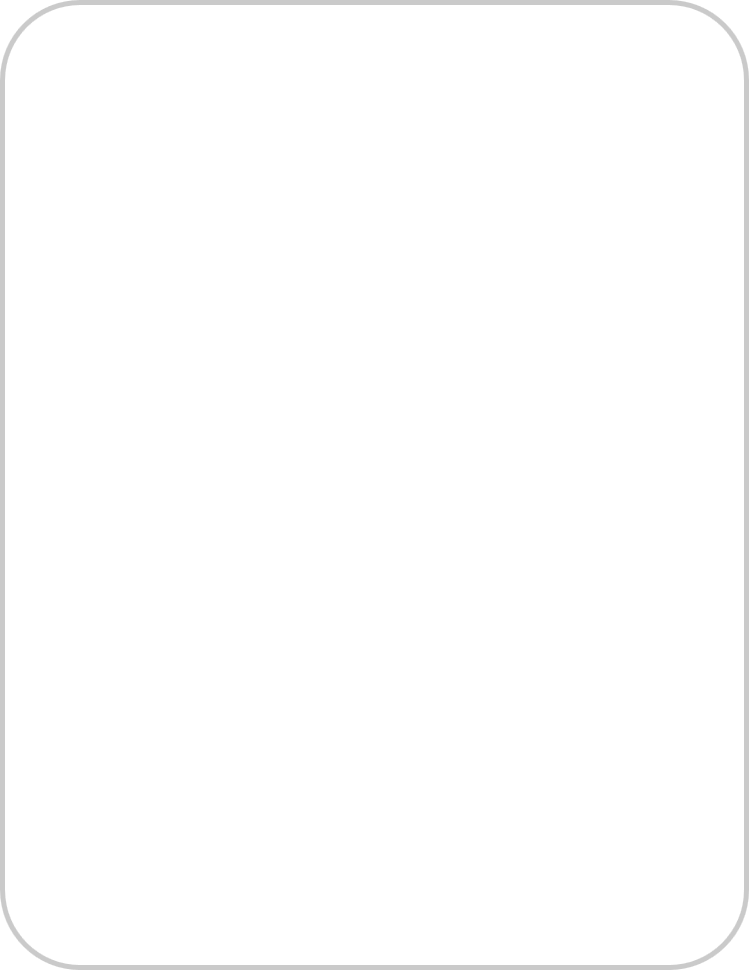
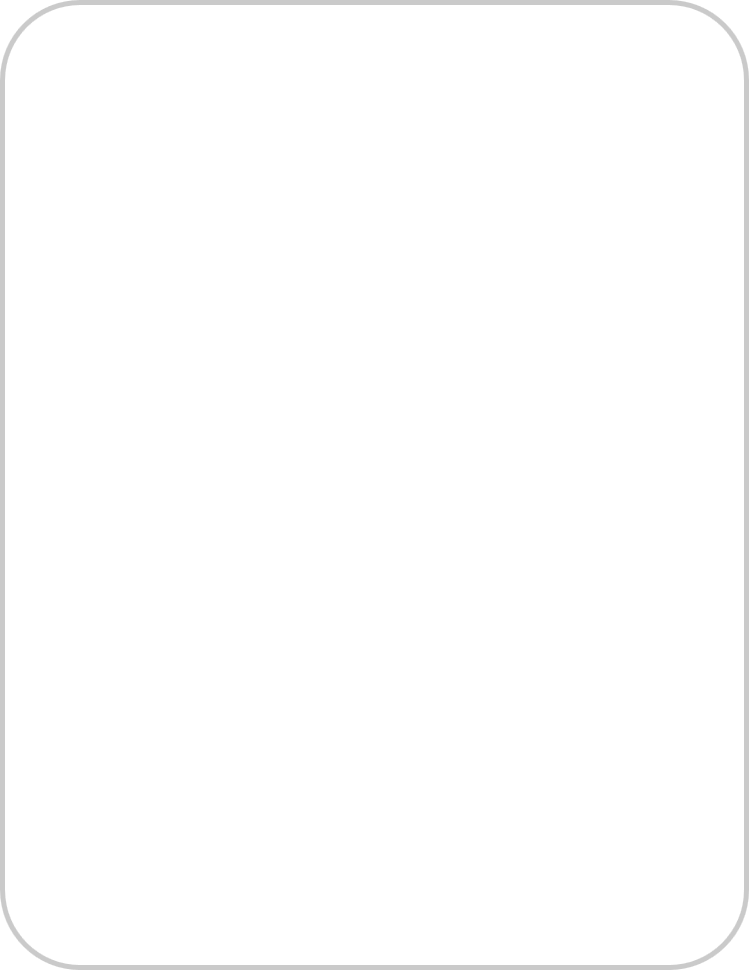
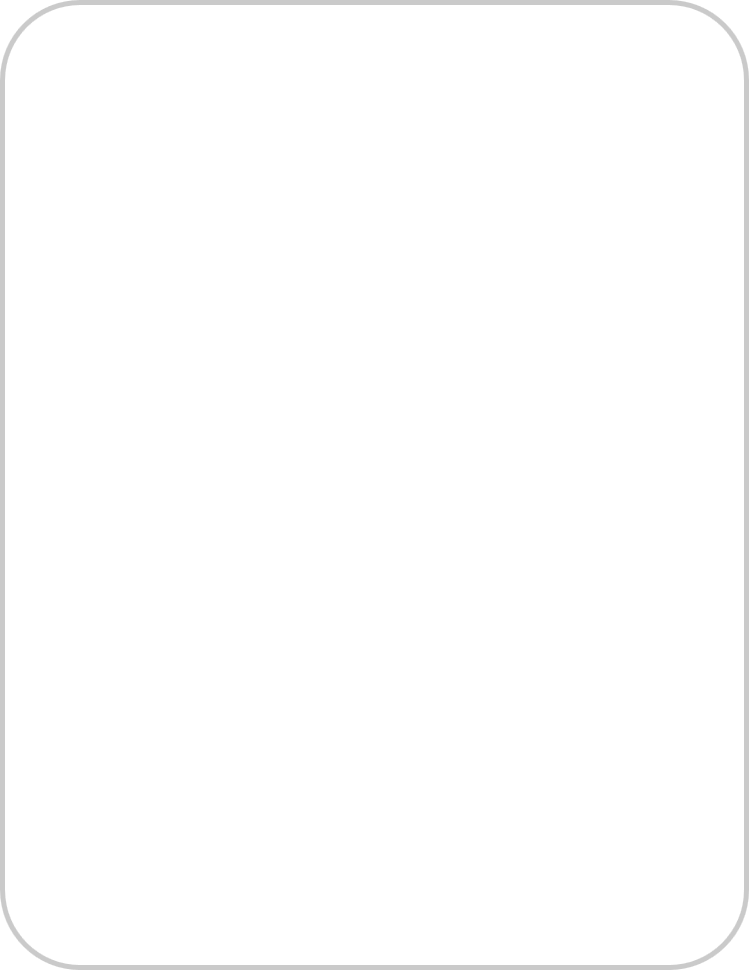





Causal Hypotheses
The workflow starts with ingesting relevant knowledge (internal documents). Guided by the use case specific KPIs, the user navigates the session to generate causal hypotheses that explain how the KPIs may be influenced, incorporating both first principles and enterprise-specific practices.
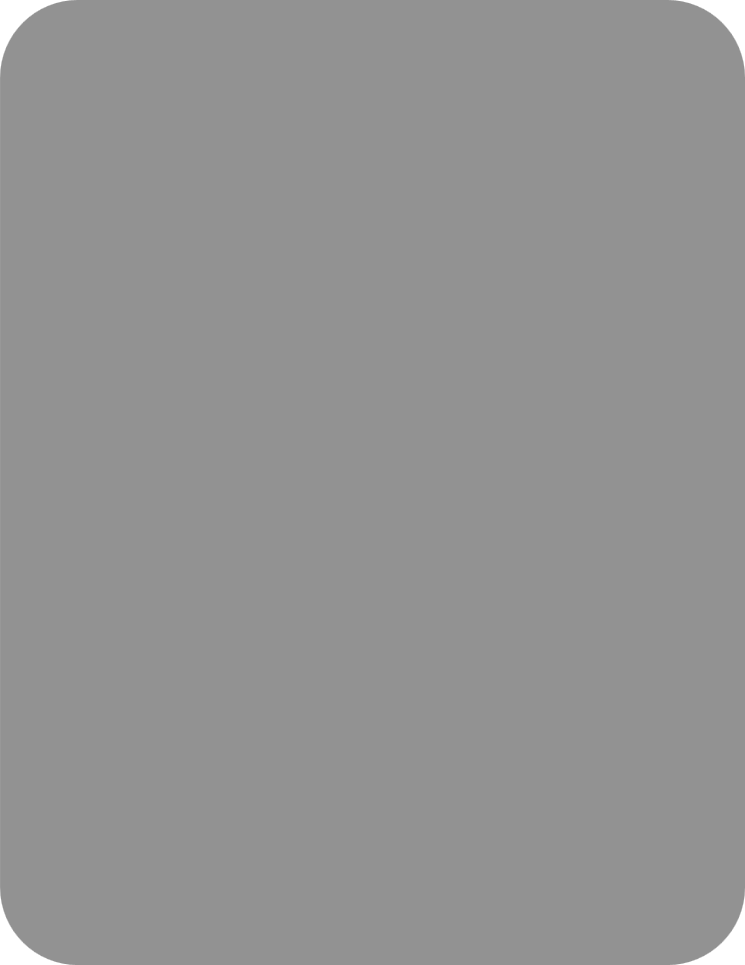
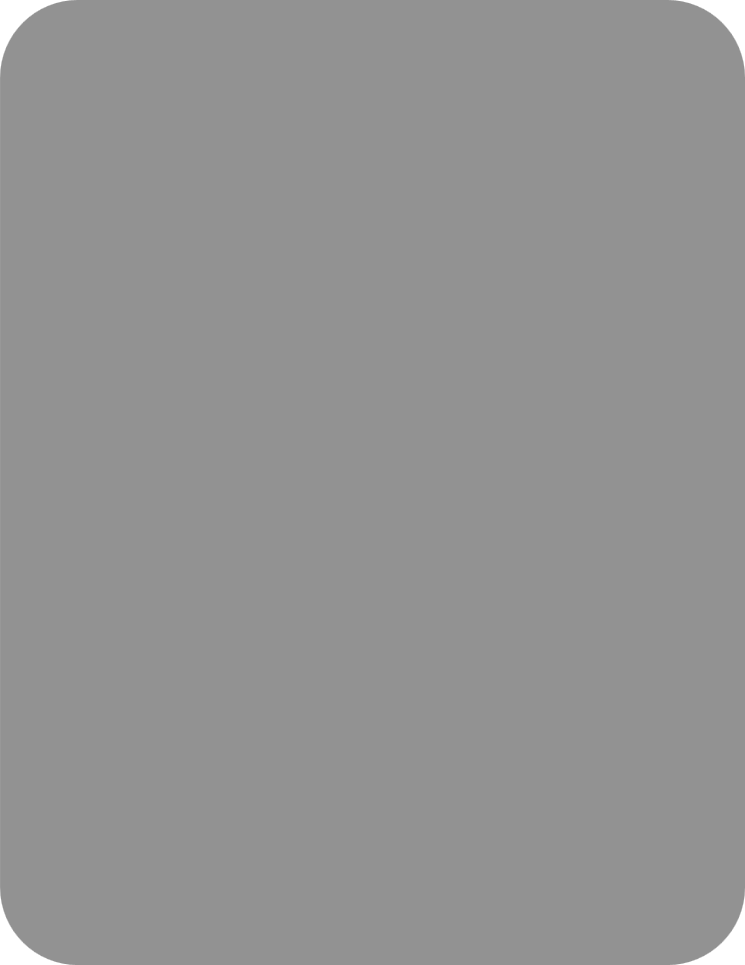
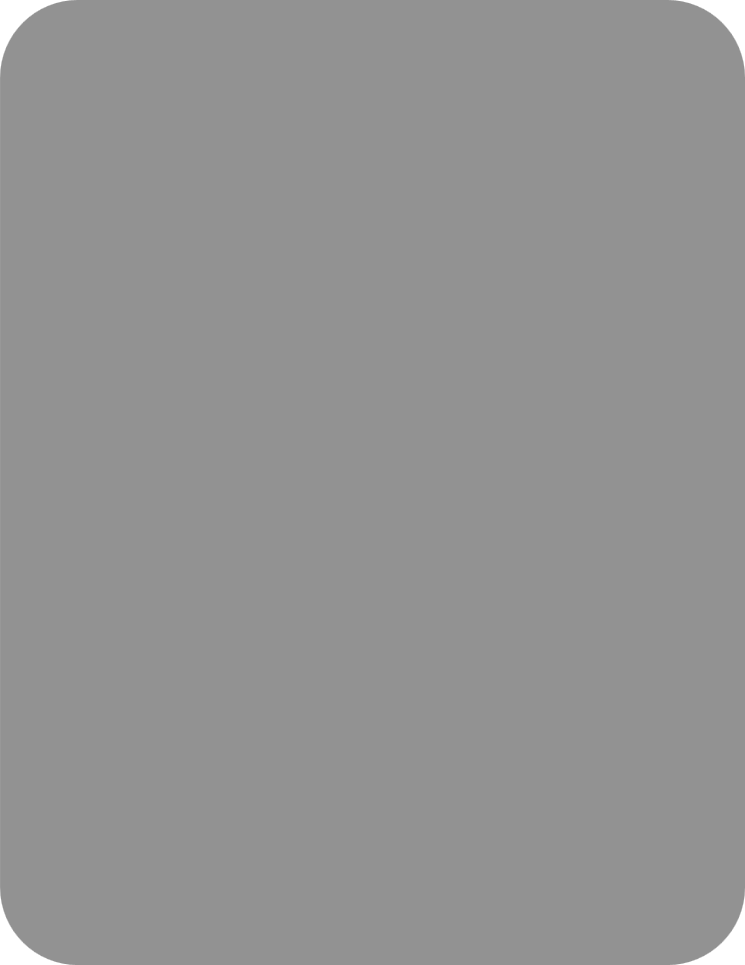
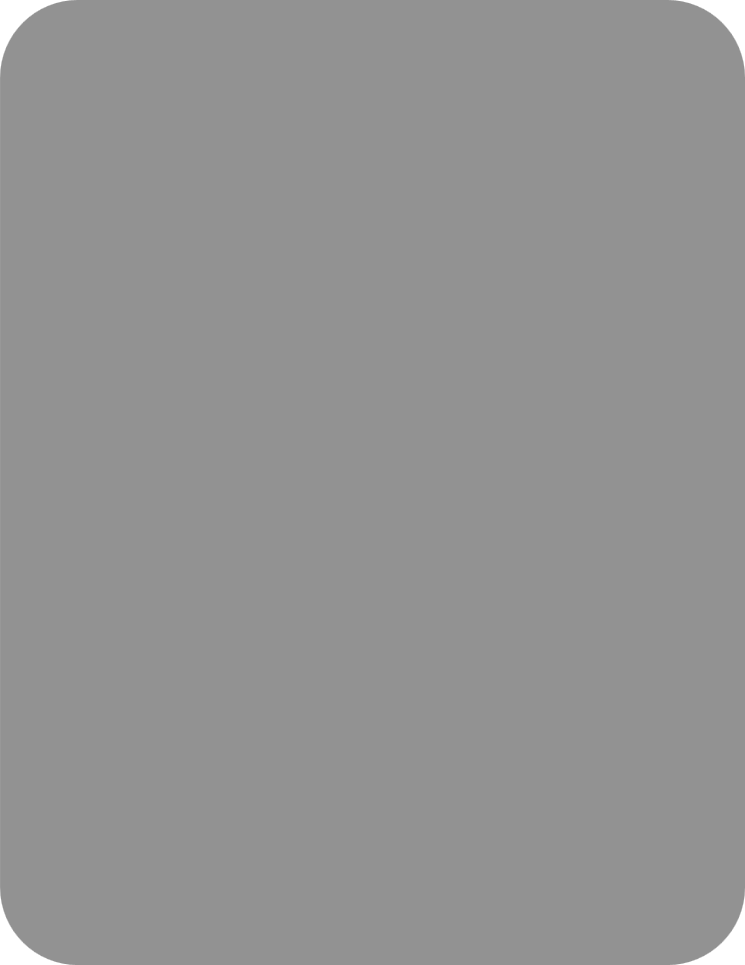
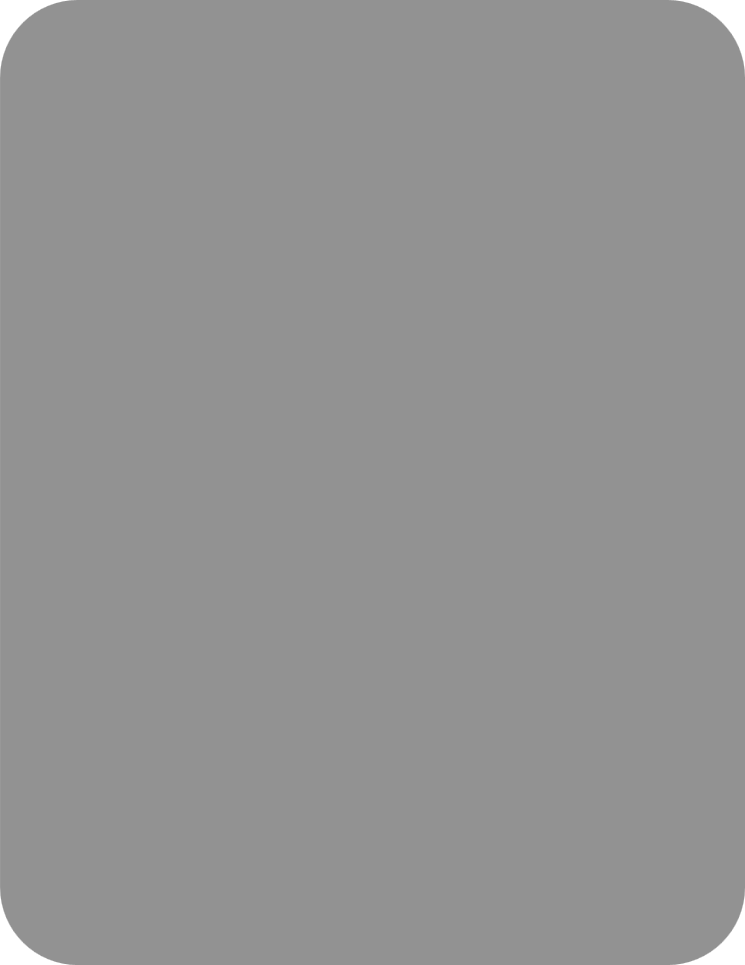
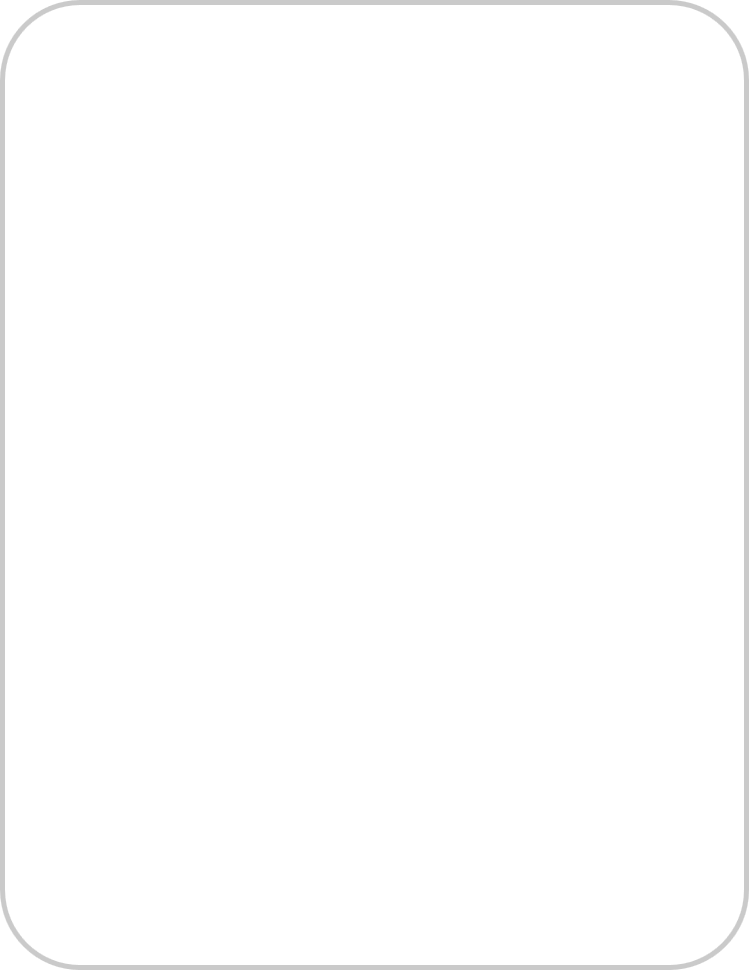
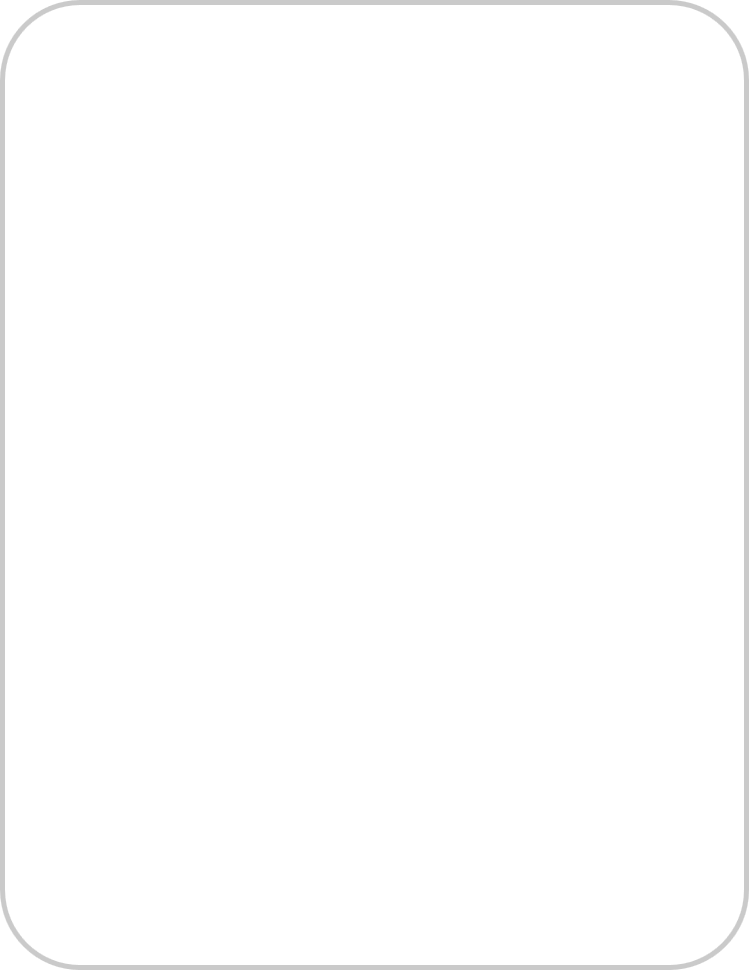
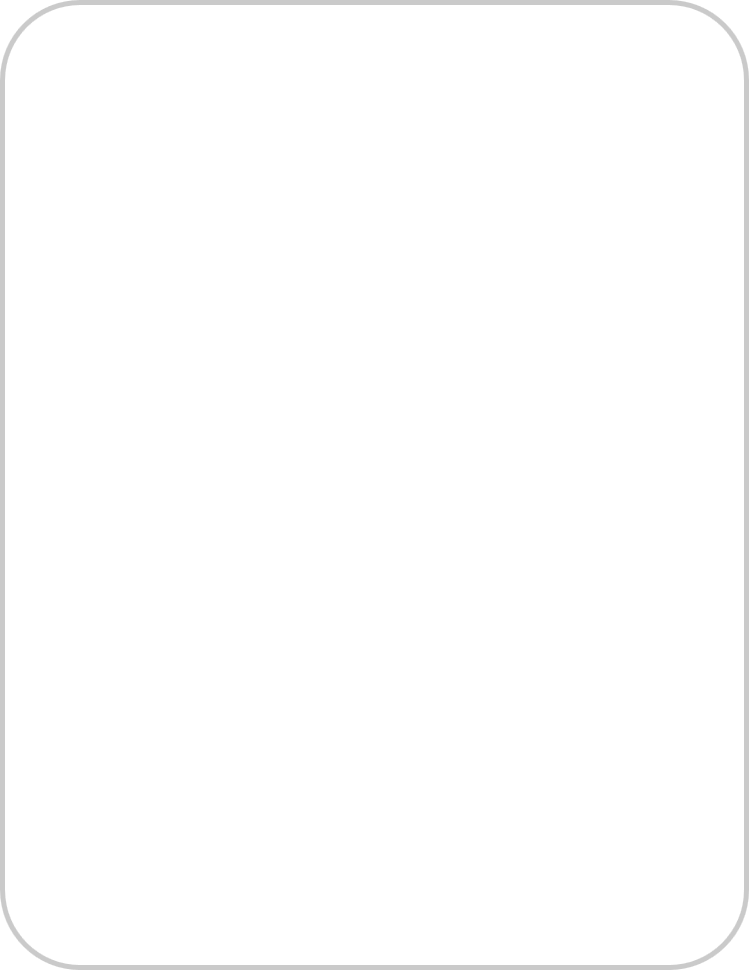
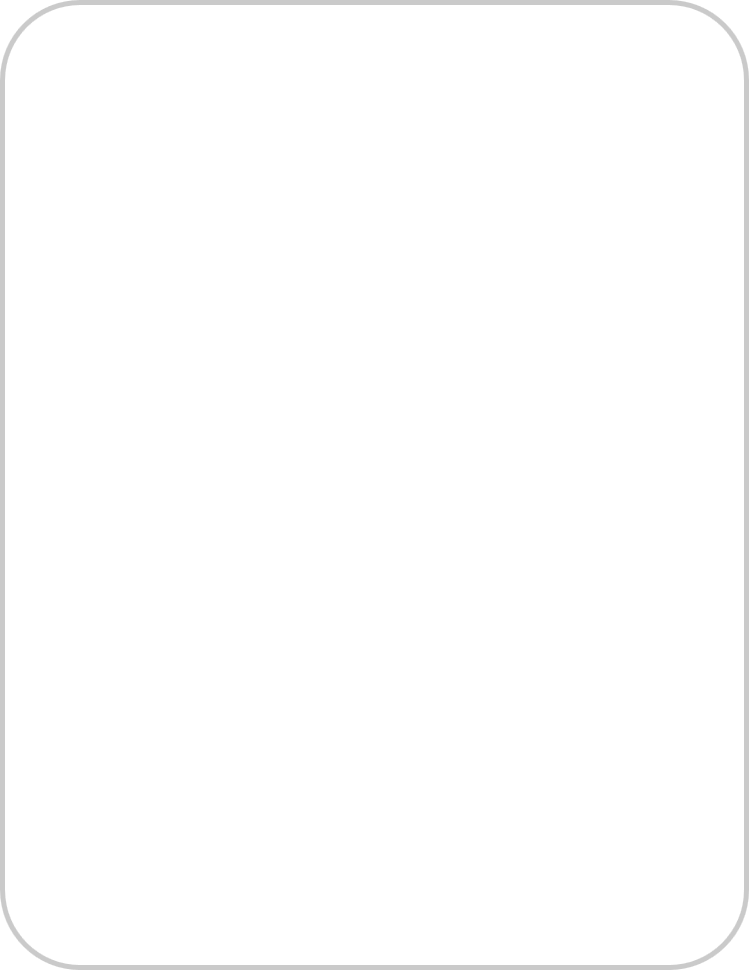
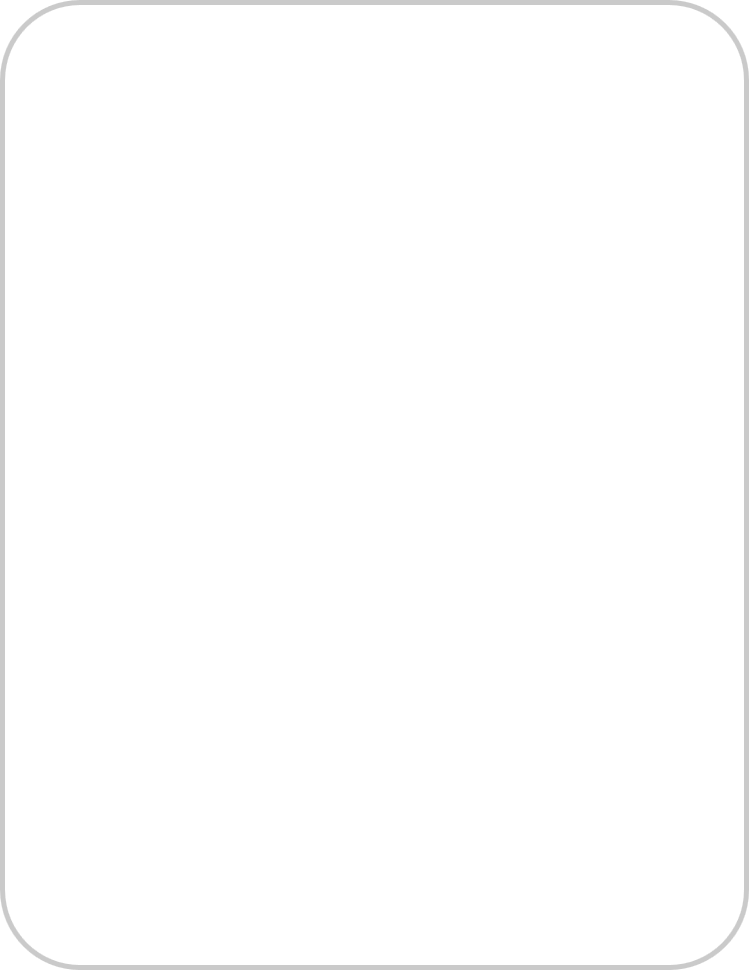





Data Context
The causal hypotheses outline potential causes for each KPI, with only a subset relevant to a specific scenario. These hypotheses guides what data to collect. The gathered data contextualizes a scenario by focusing on the specific hypotheses subset that impacts the analysis.
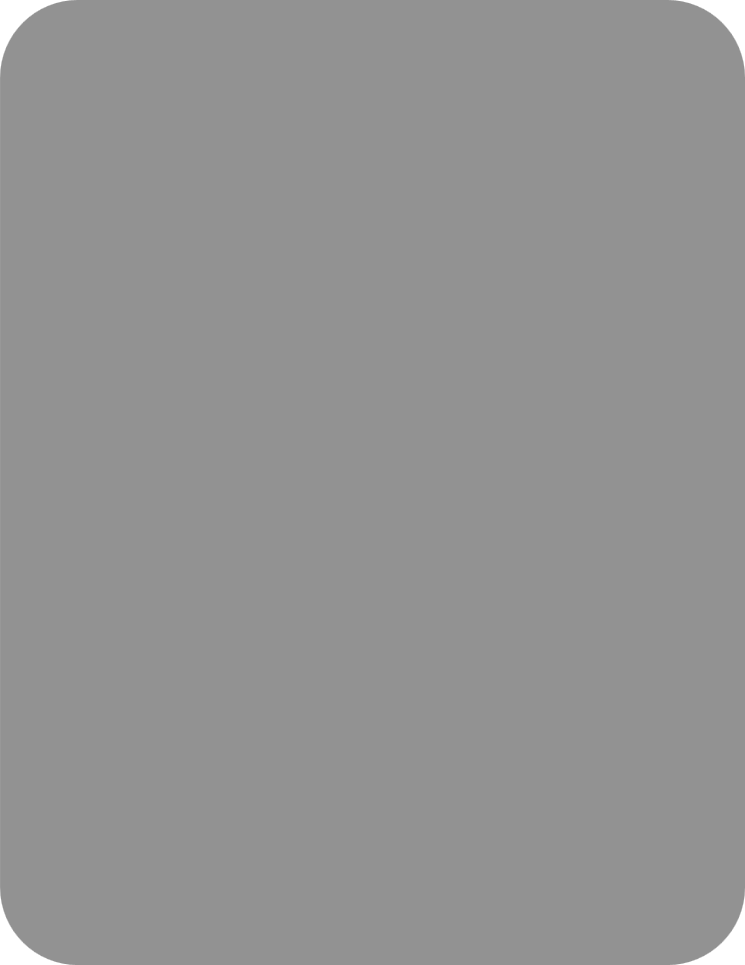
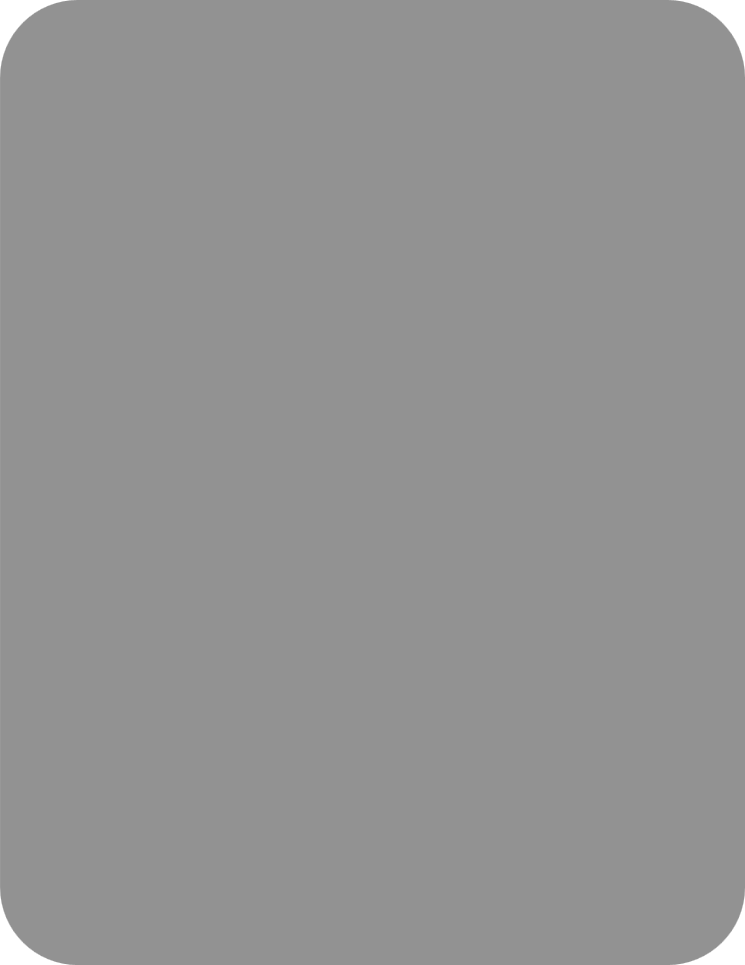
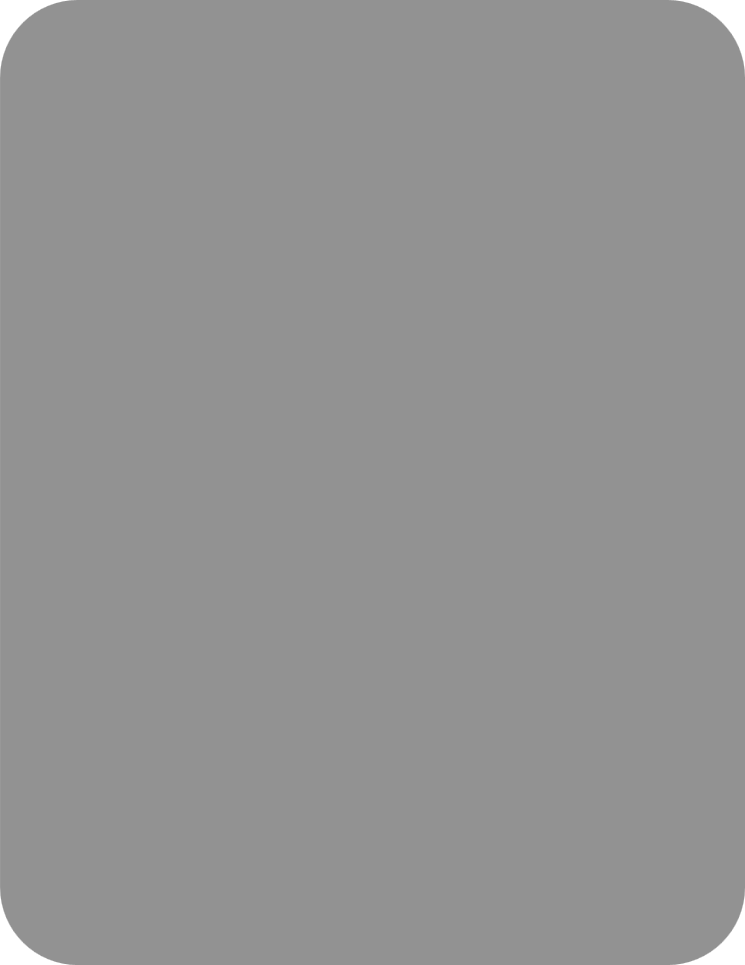
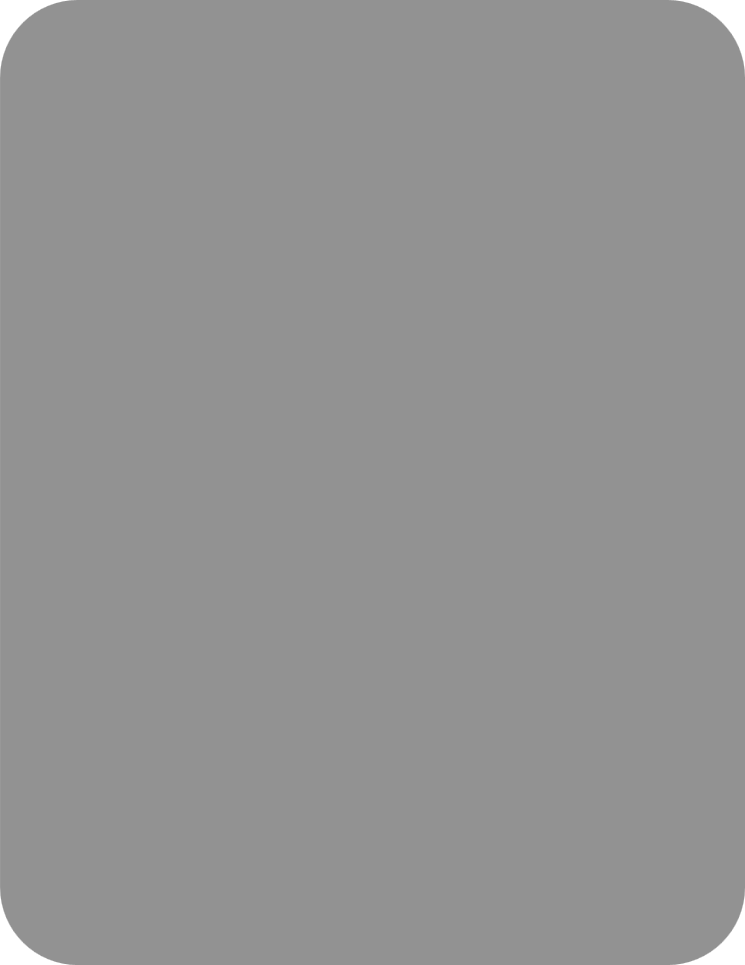
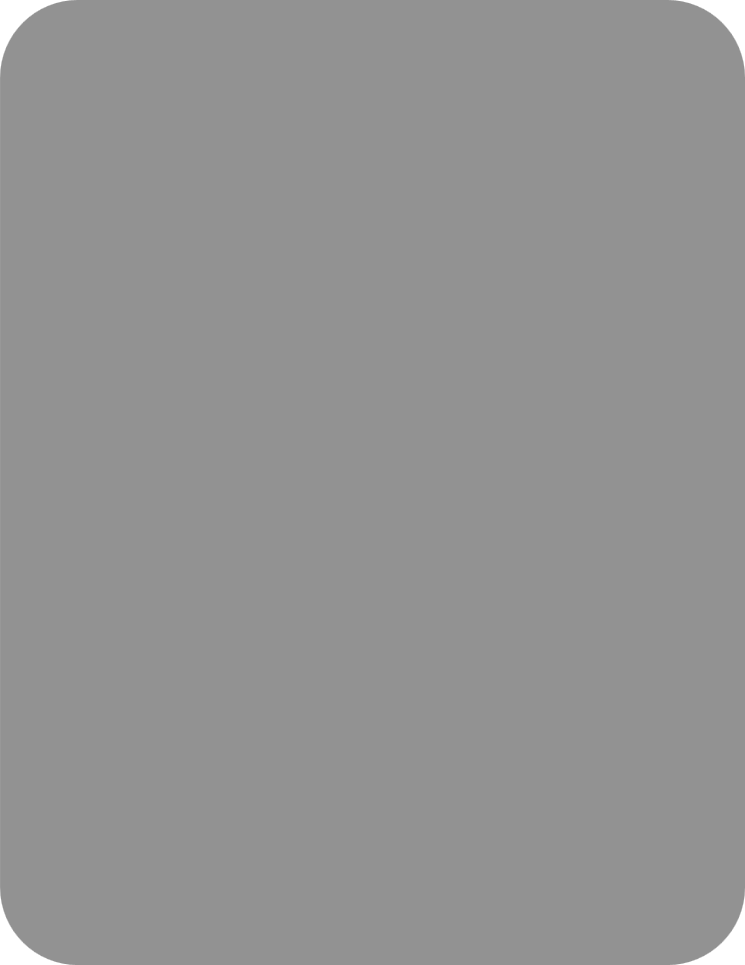
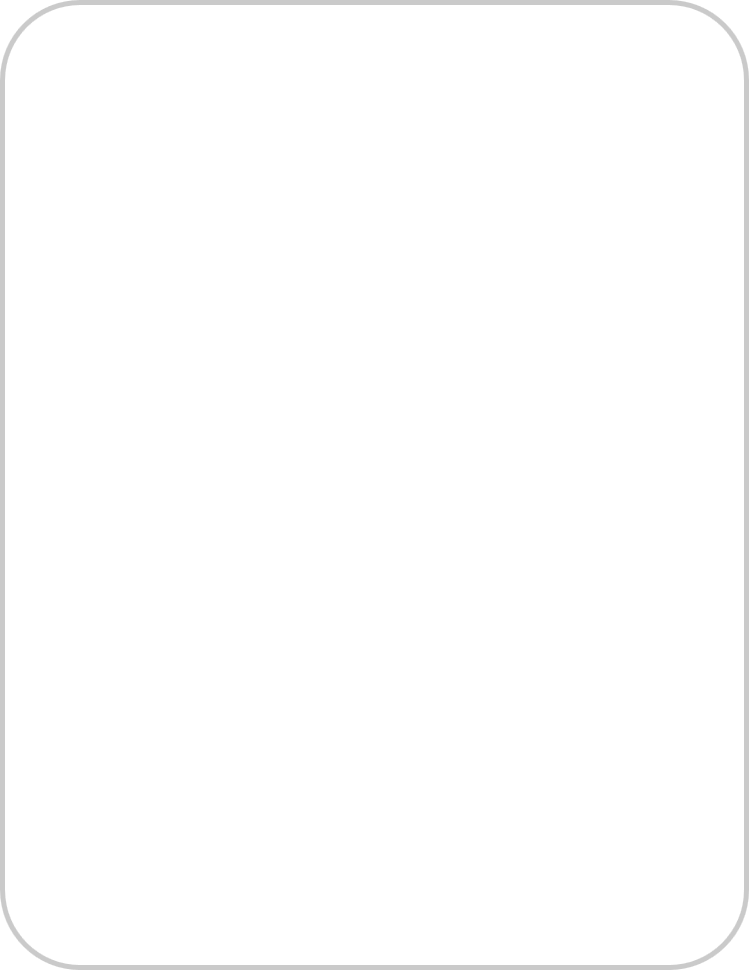
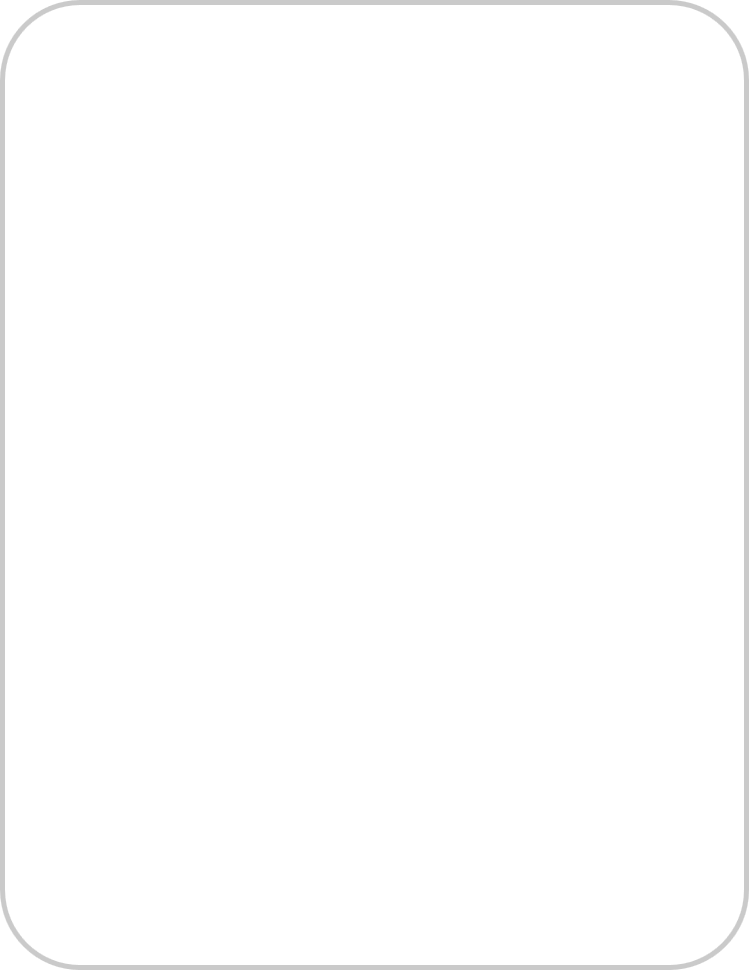
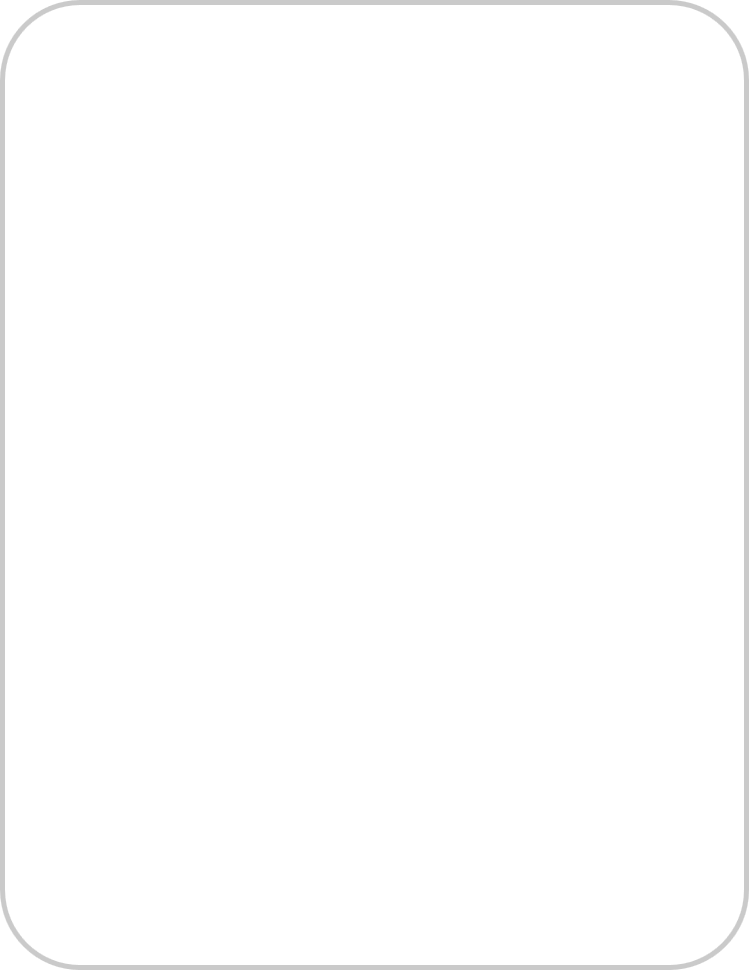
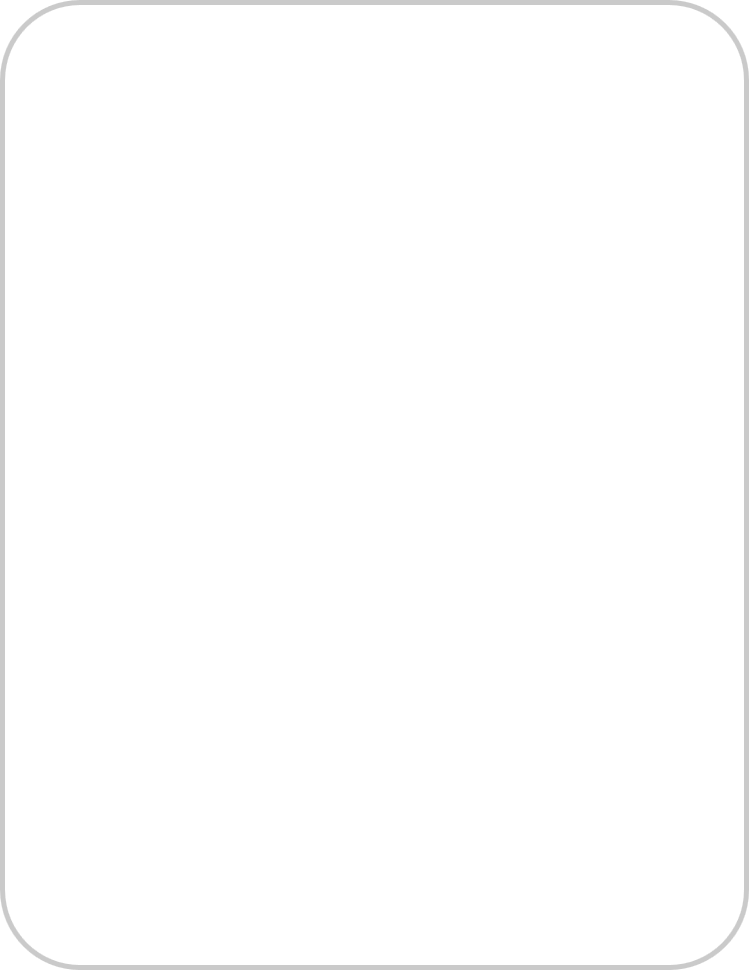
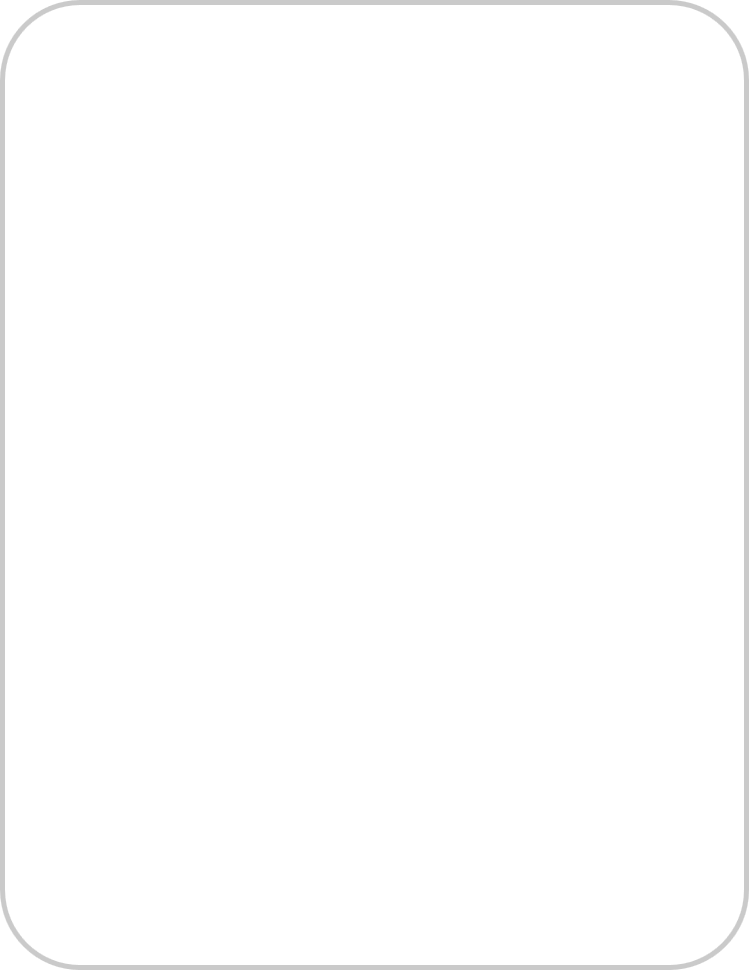





Causal Analysis
The contextualized hypotheses and causal model triggers several downstream causal analysis on IT and OT data. The causal analysis includes intervention for root cause, counterfactual for what-if, and optimization for multi-objective business decisions.
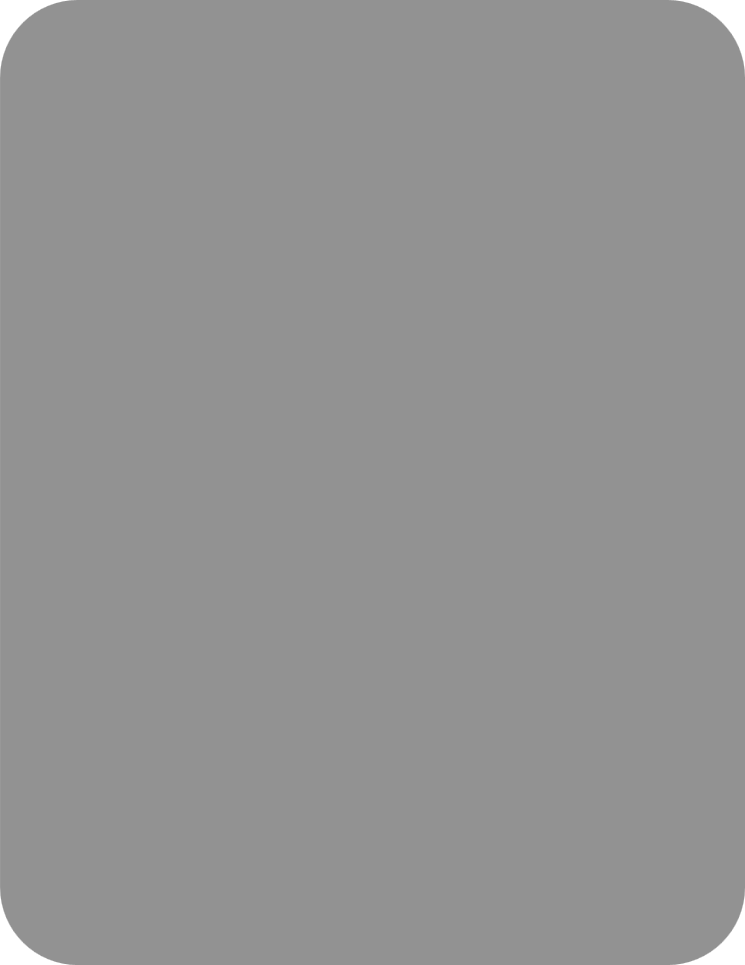
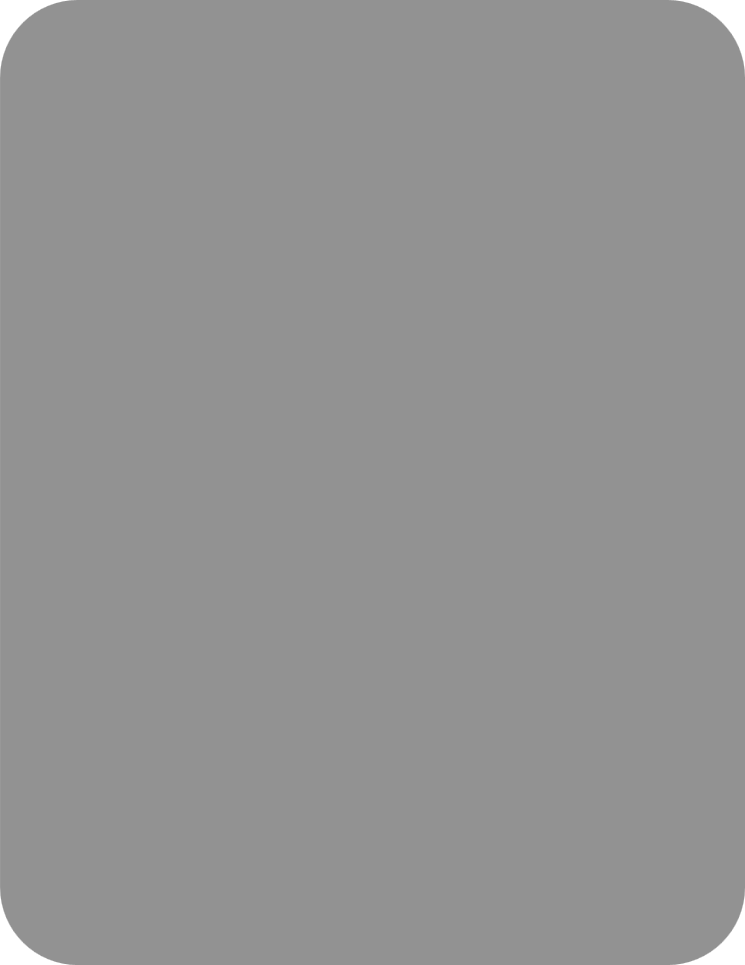
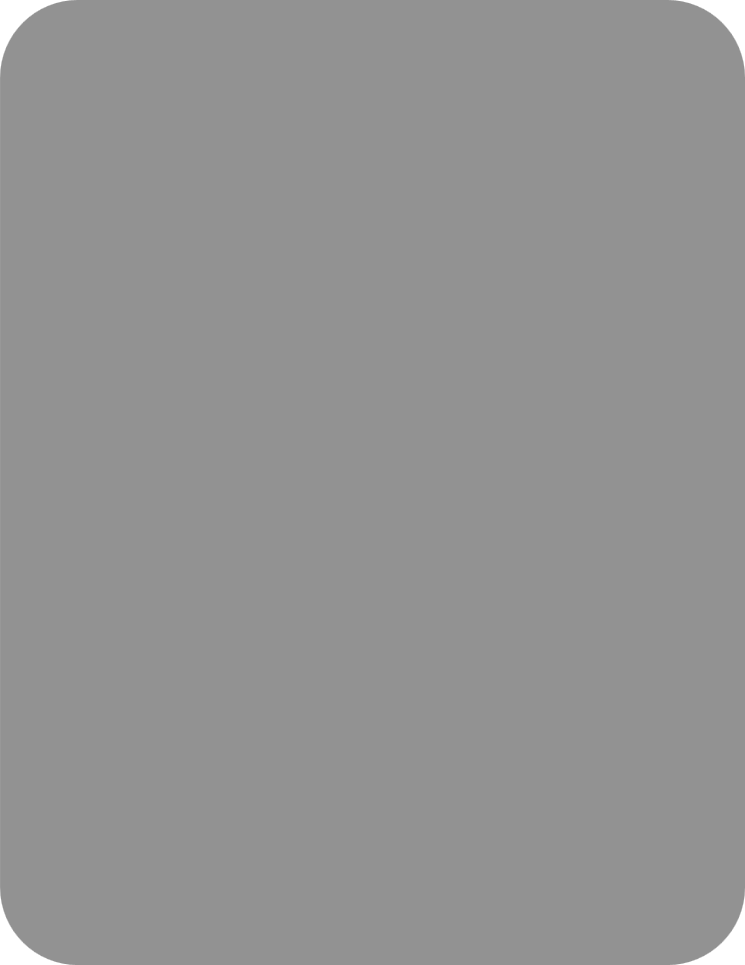
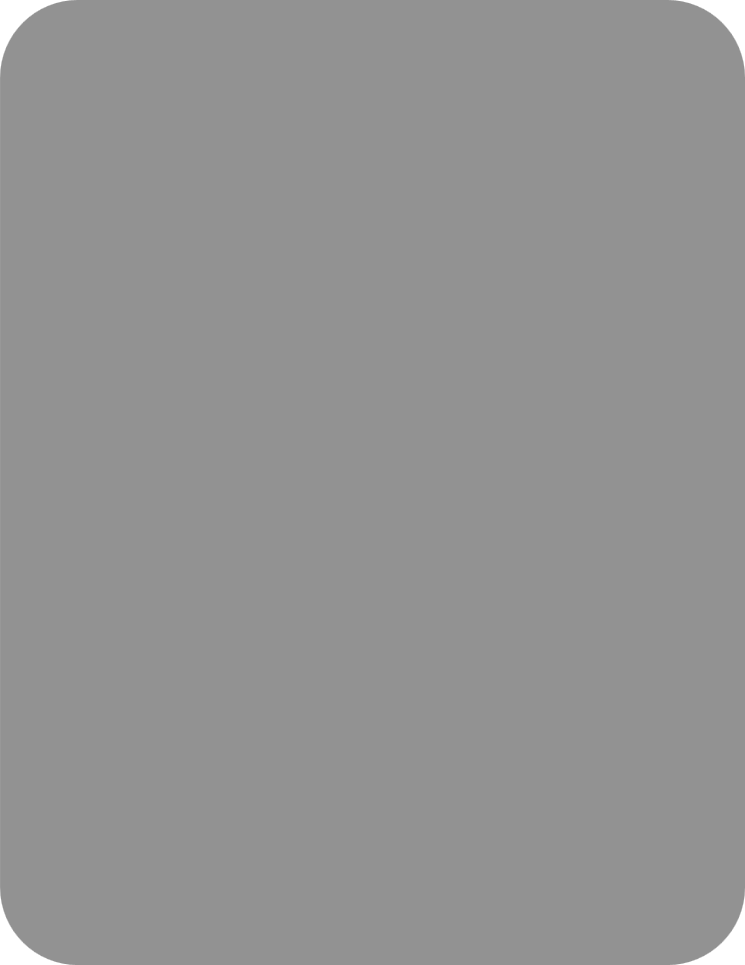
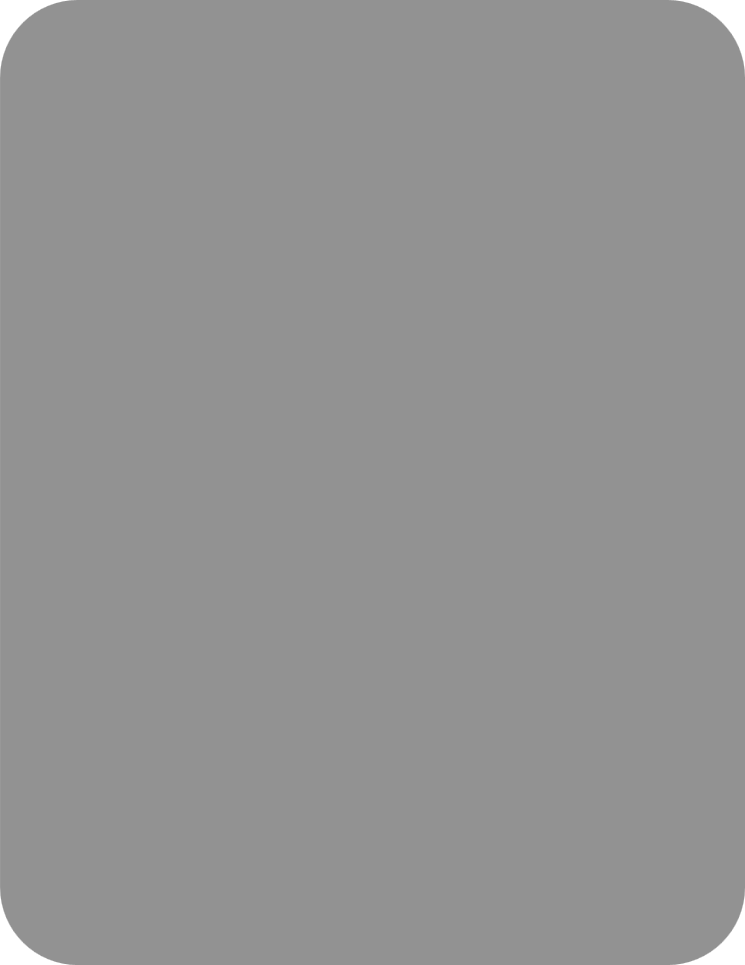
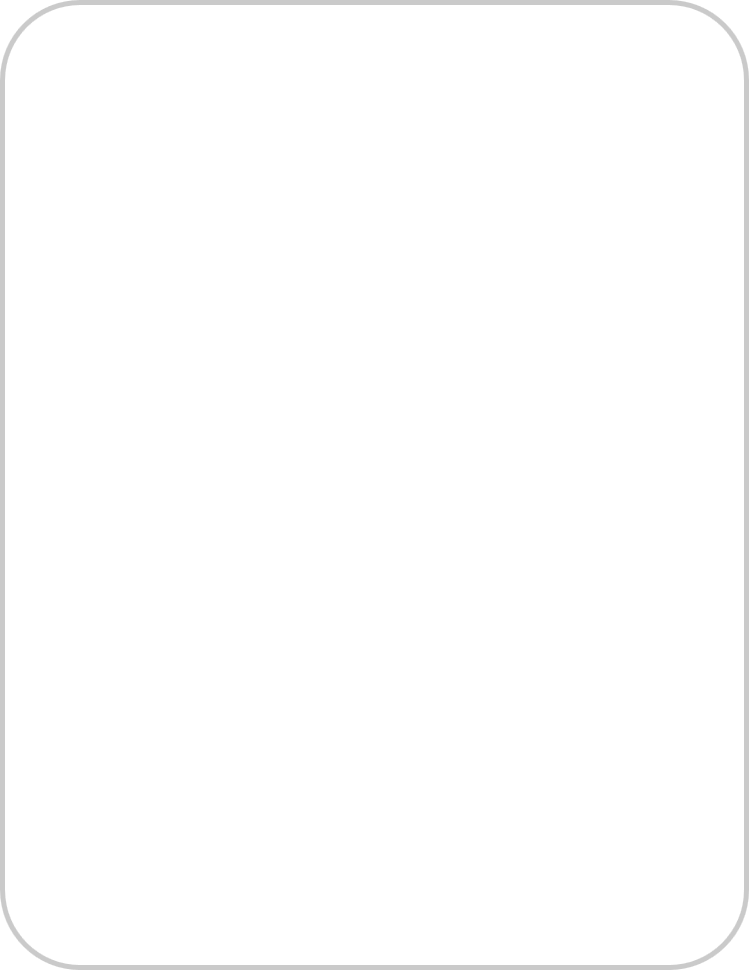
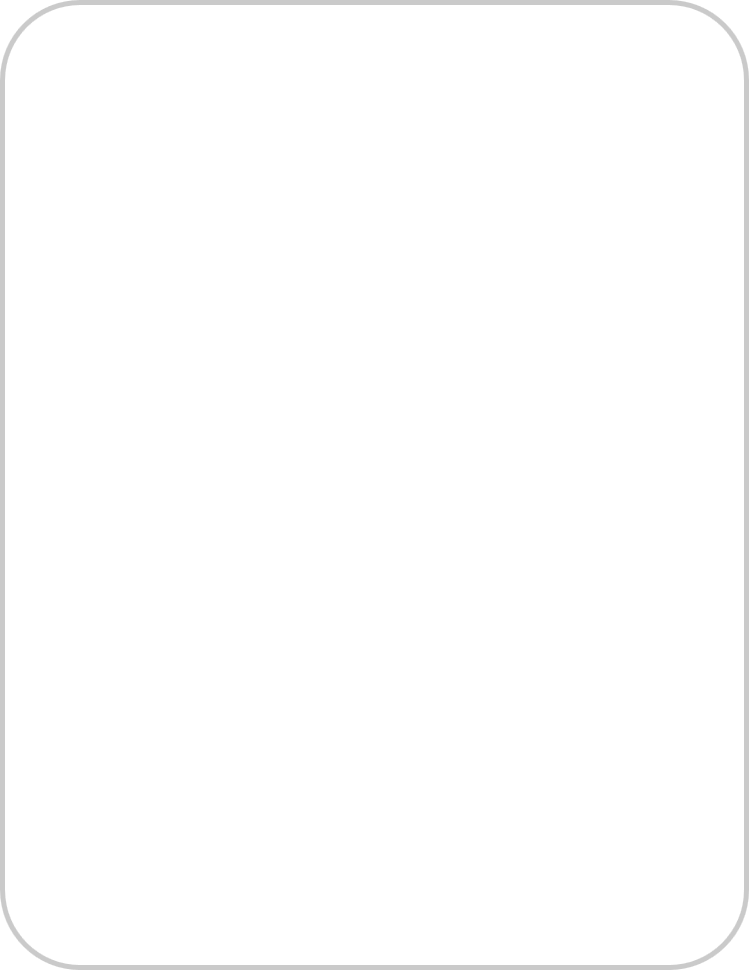
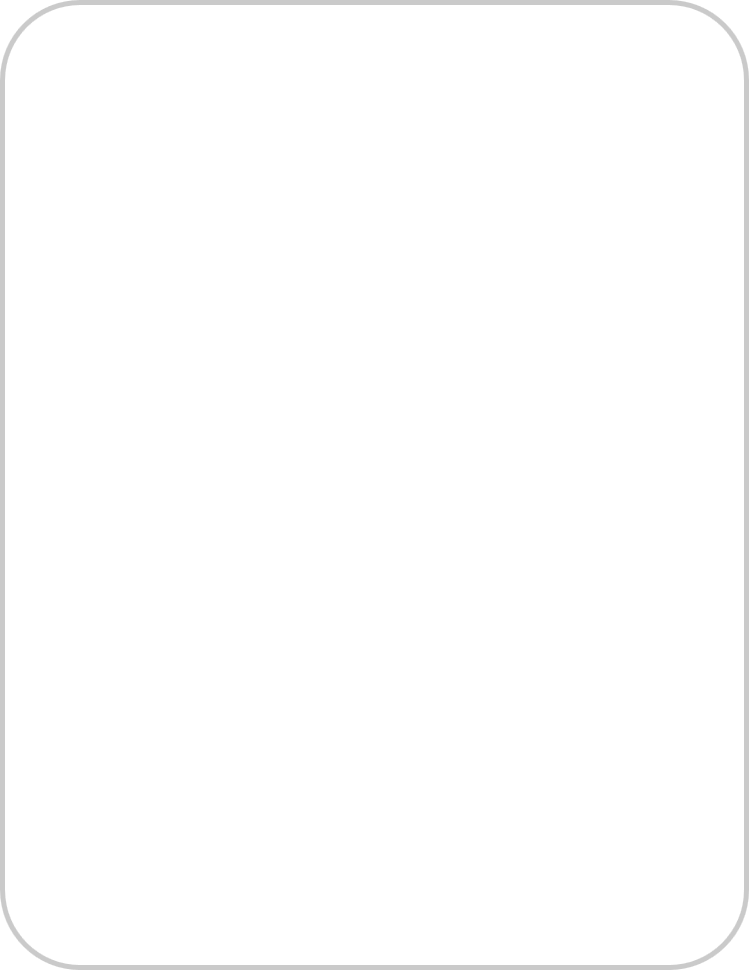
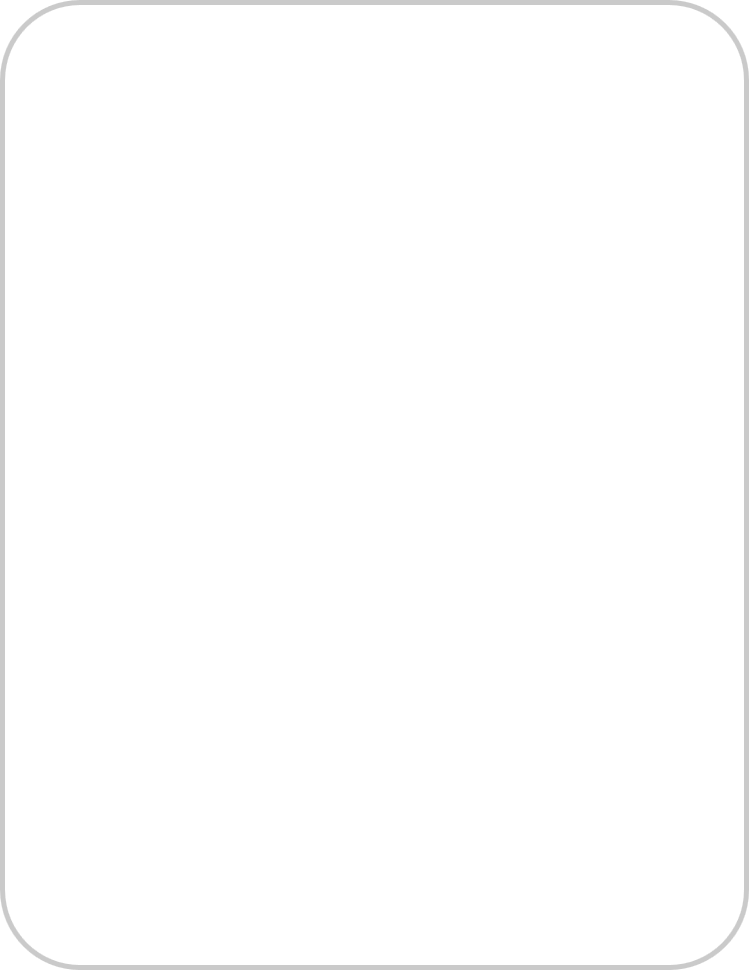
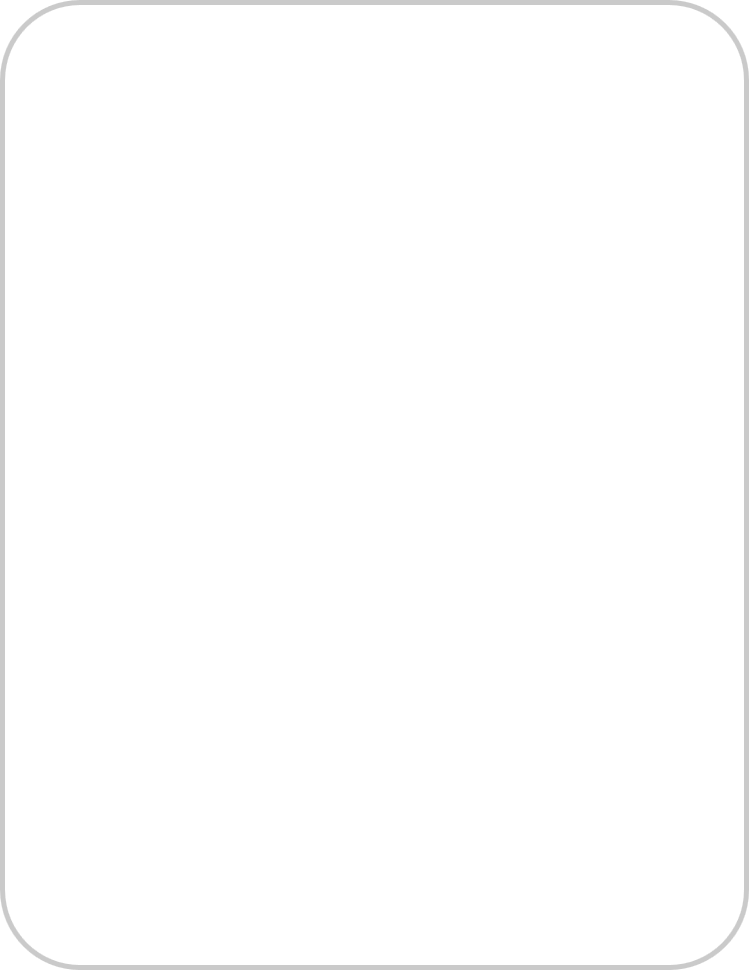





Causal AI Agents
The AI agents deliver the learned causal model and causal analysis to users IT and OT applications, offering a templated development method for swift value realization and deployment.


Causal Hypotheses
TRAIN automatically generates causal models by harnessing both enterprise knowledge—encompassing subject matter expertise, rules, theories and data—time series , statistical analysis and pattern recognition
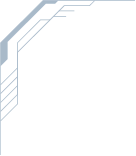
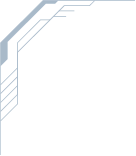
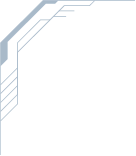
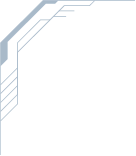
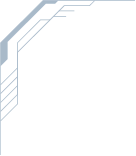
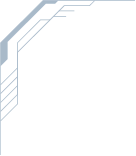
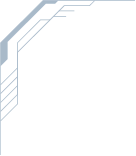
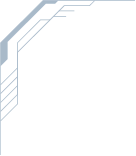
Building Causal Hypotheses
TRAIN provides a structured teaching workflow that enables users to onboard case-specific knowledge and KPIs for analysis. It then generates broader structural and functional relationships, building causal links that capture first principles in a Principle Causal Model (PCM) and enterprise practices in a Rational Causal Model (RCM). These causal models produce hypotheses that identify key drivers and form the basis for deeper analysis.








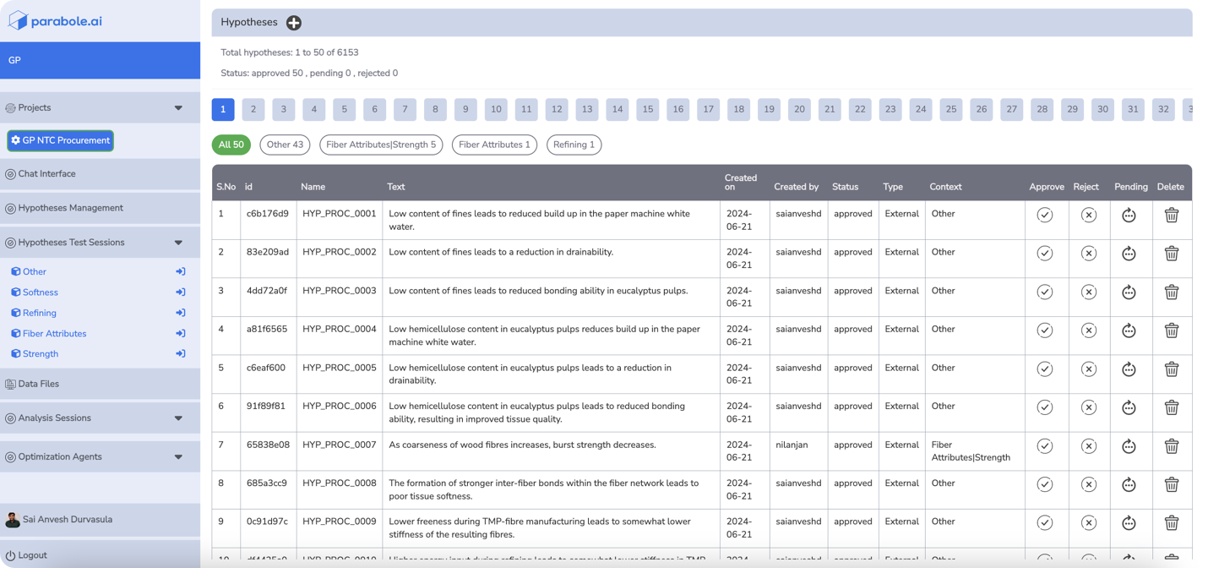
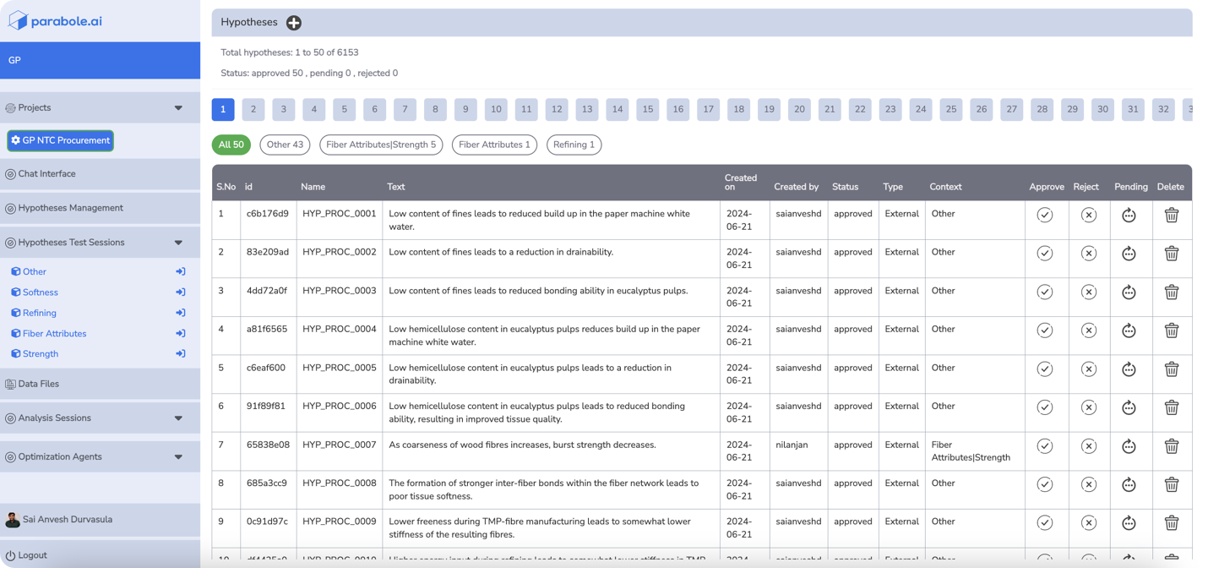
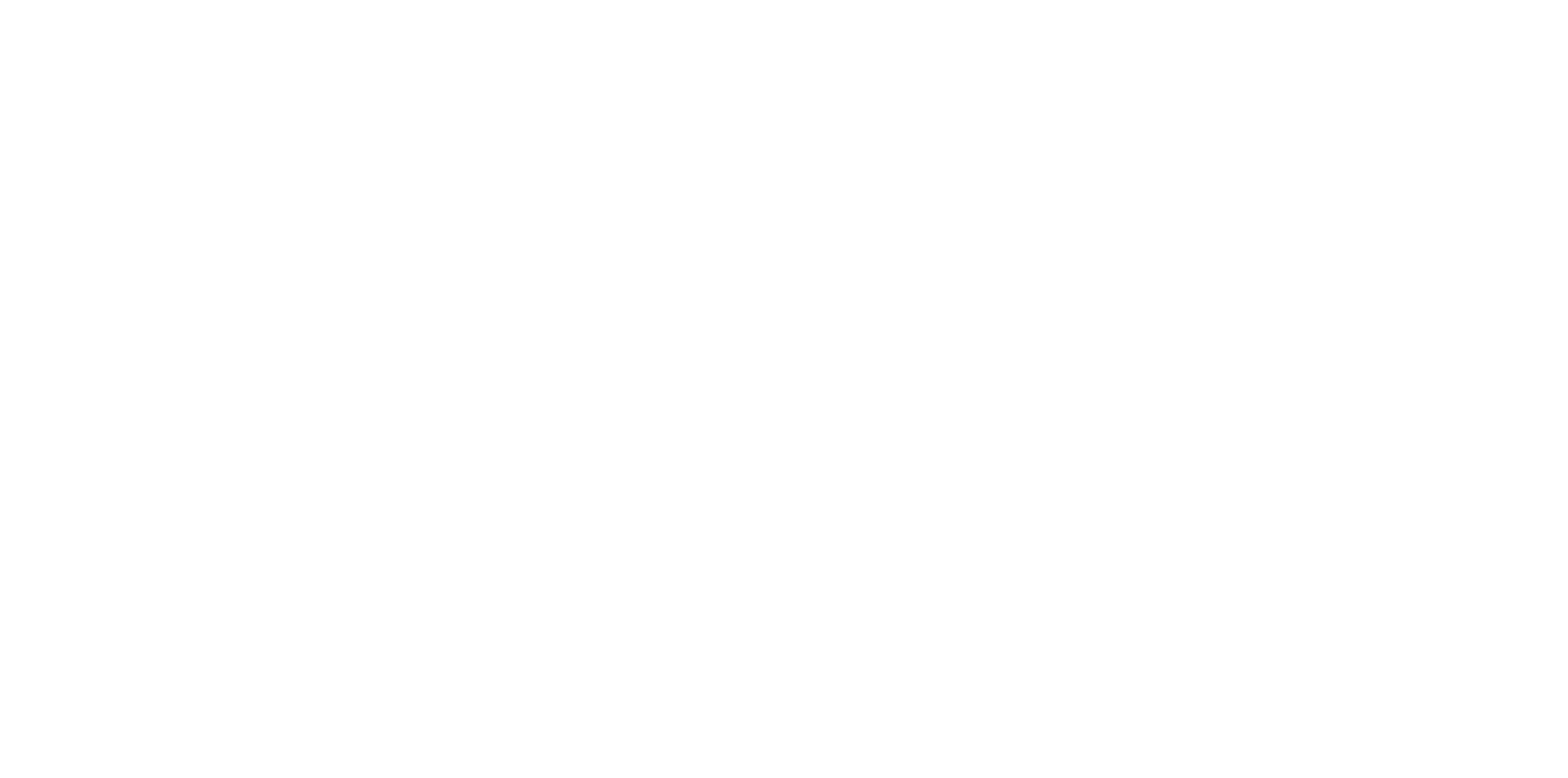
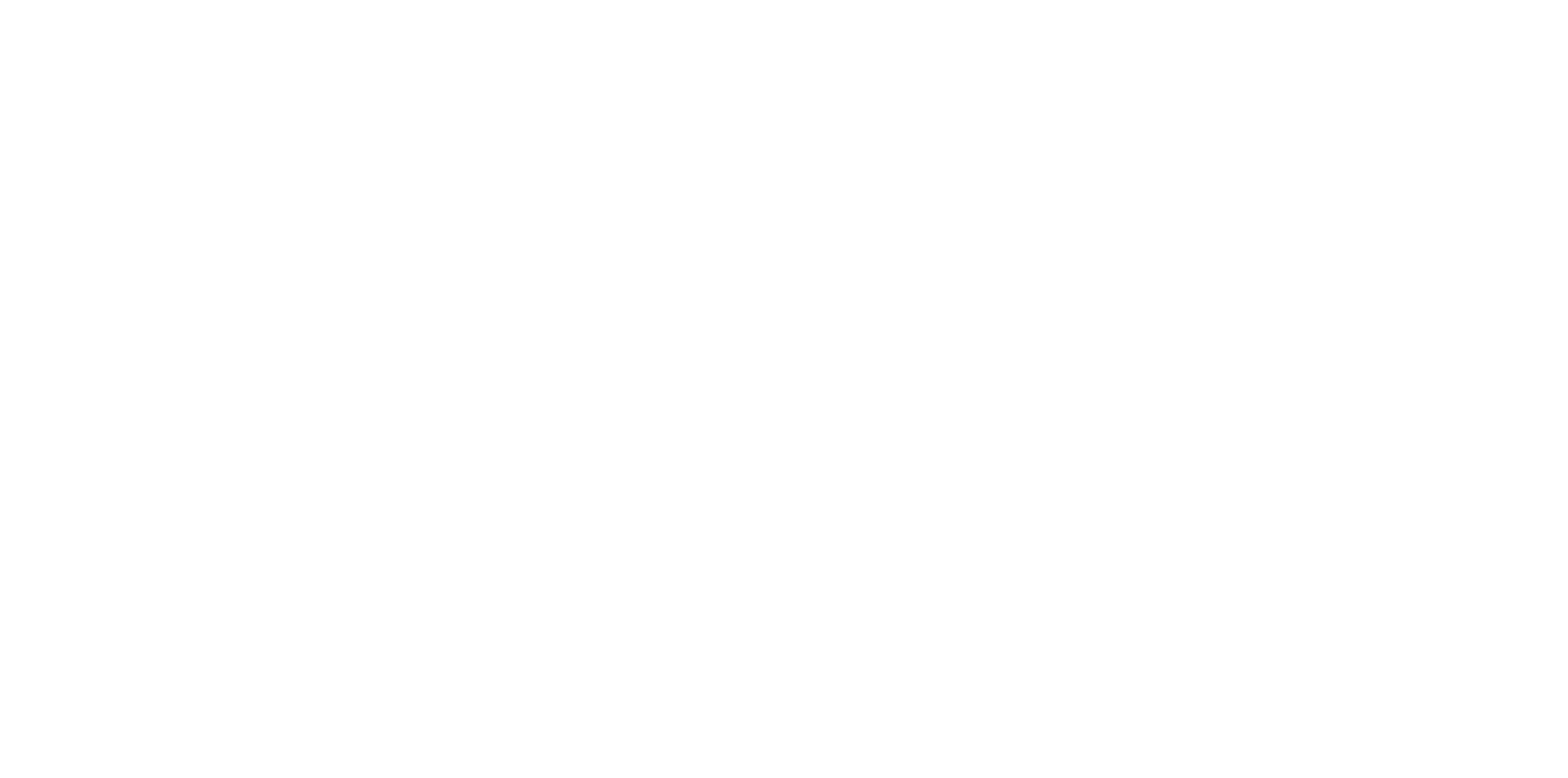
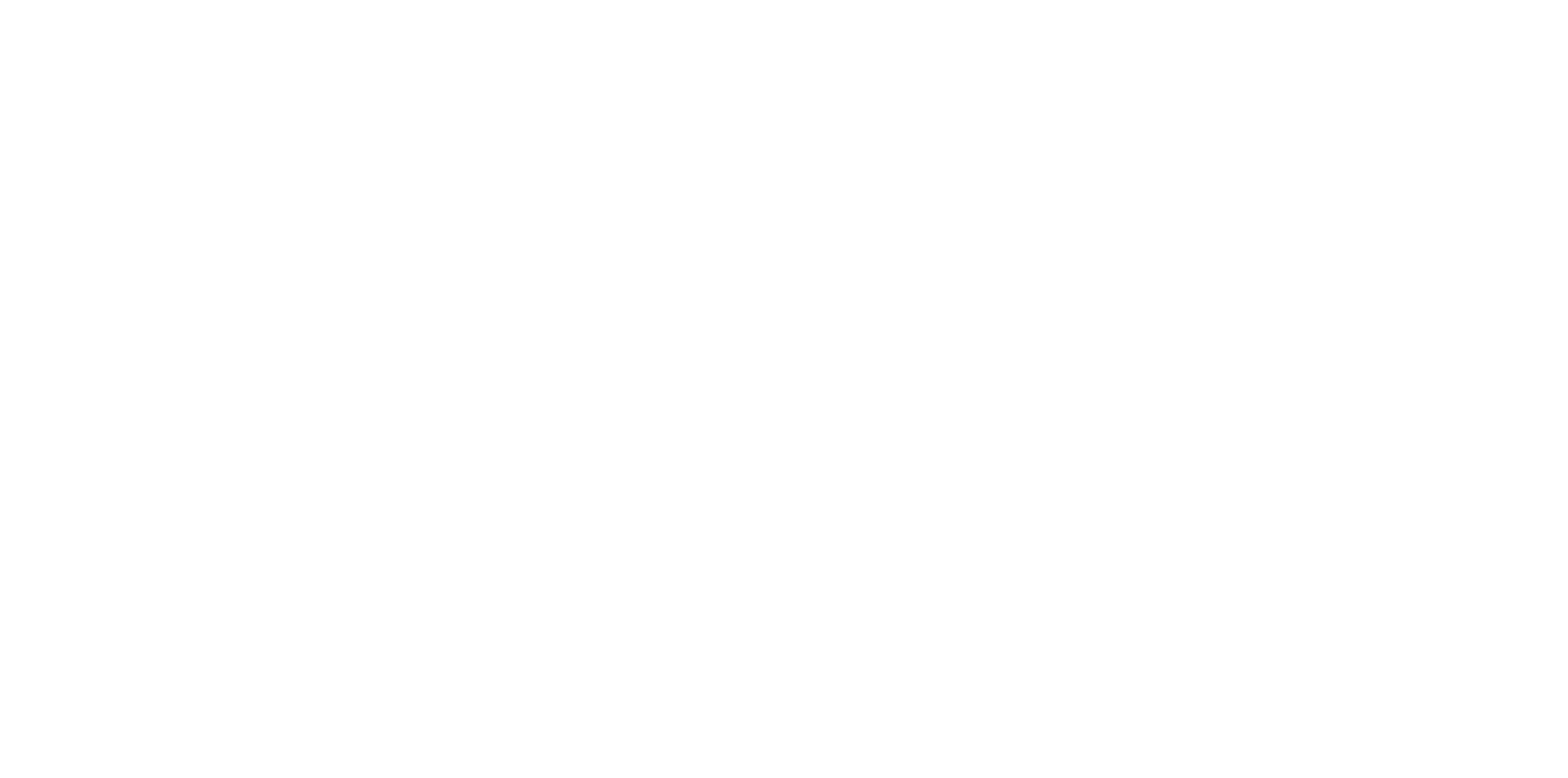
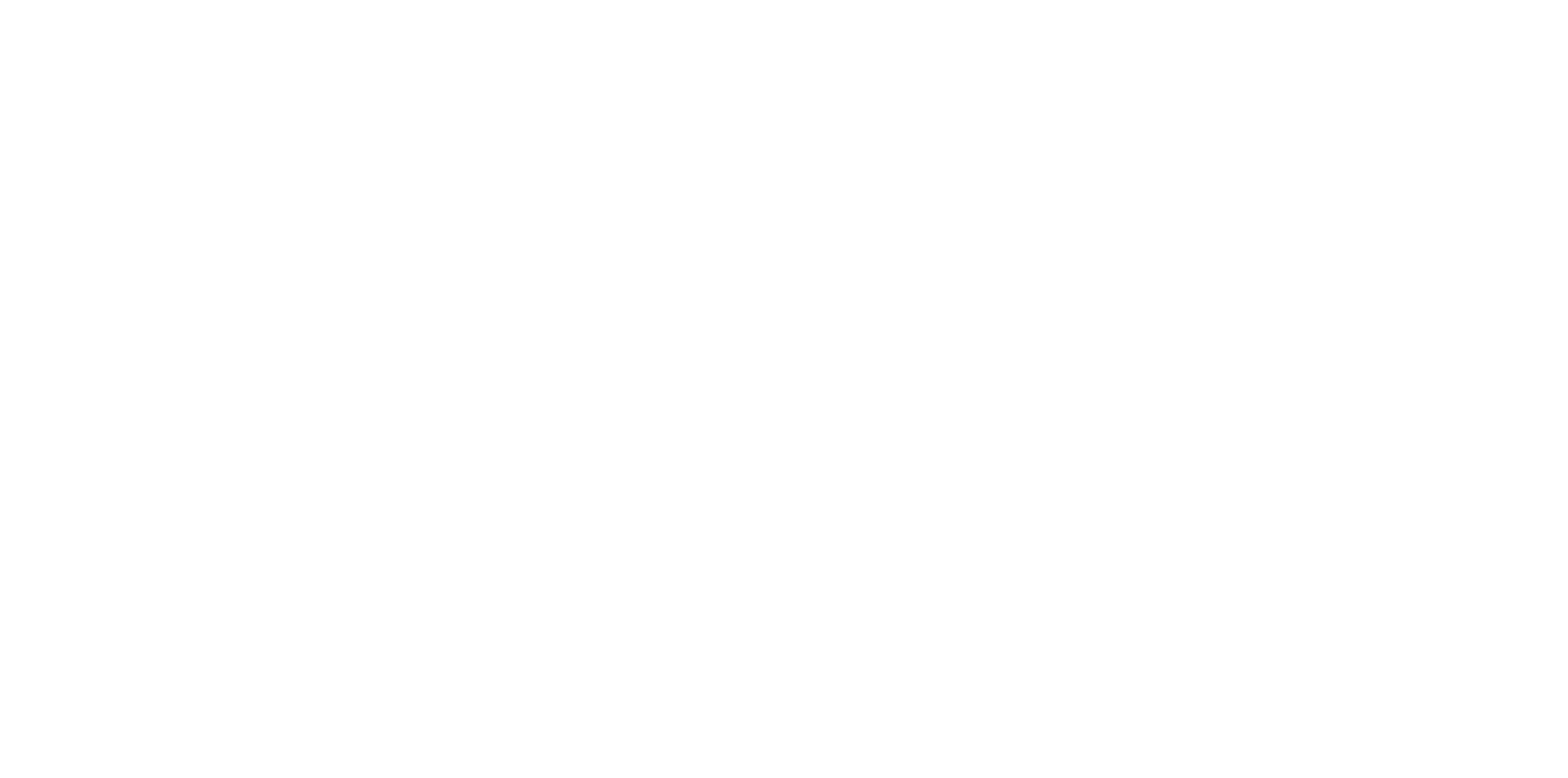
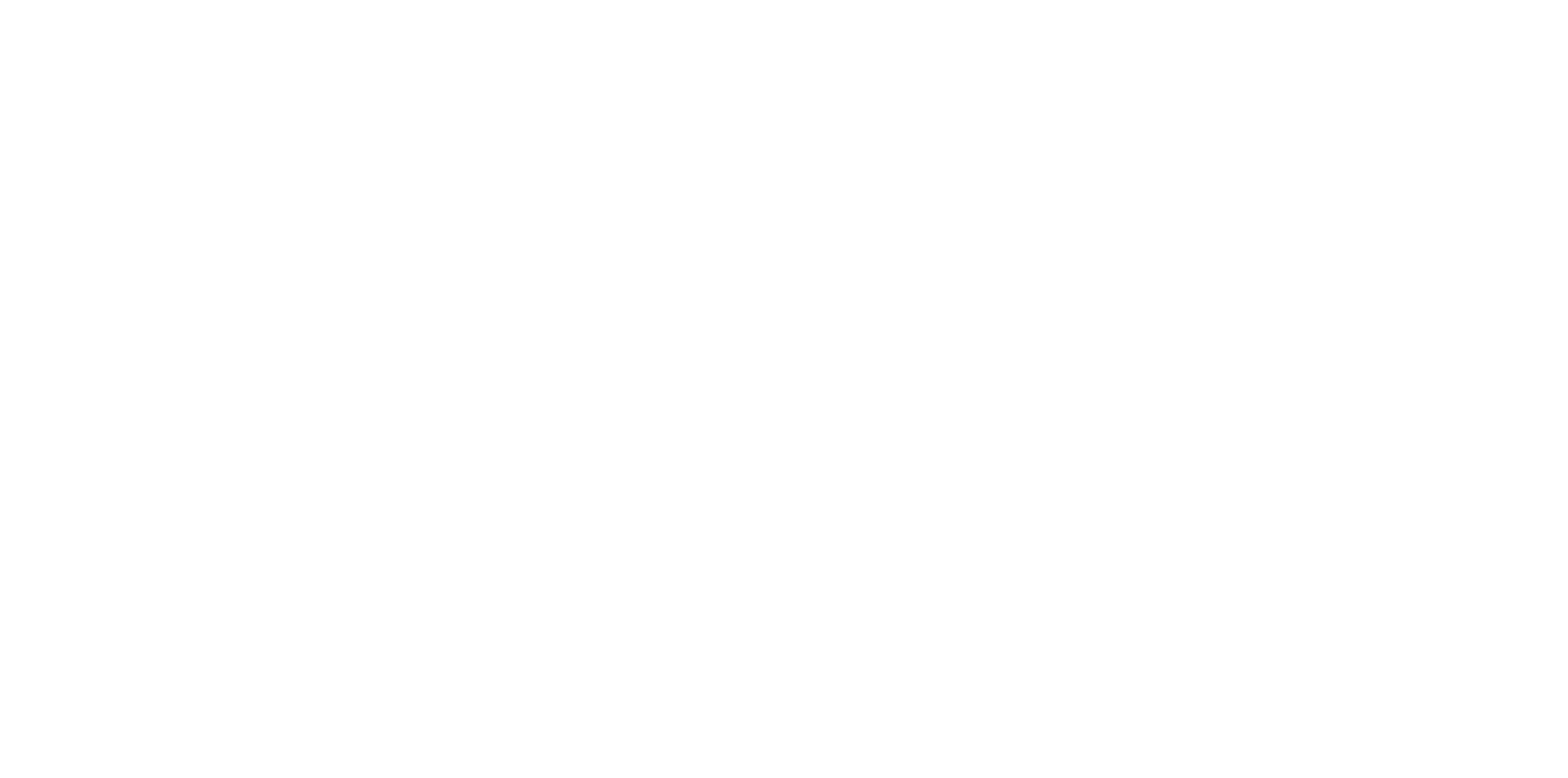
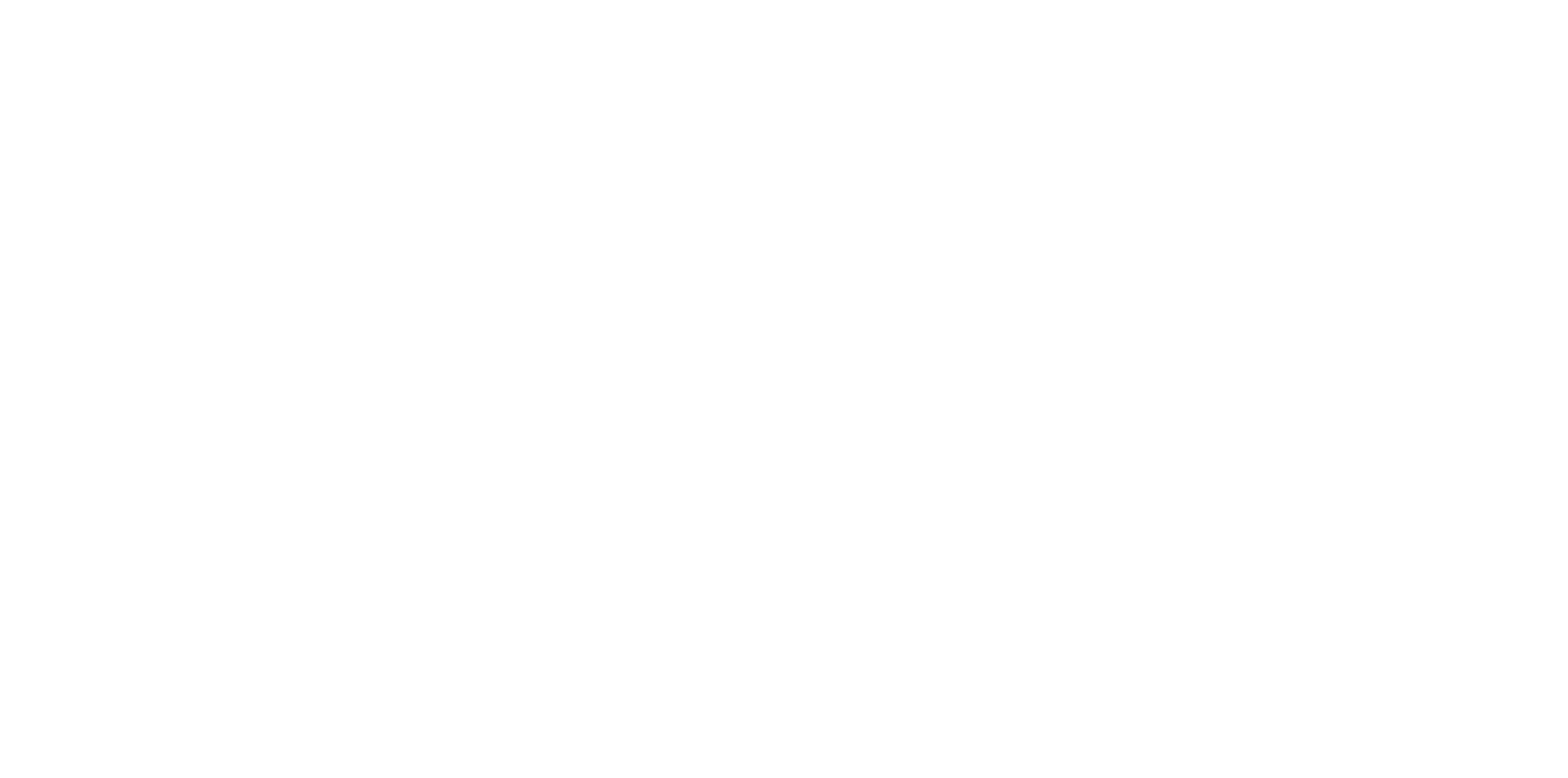
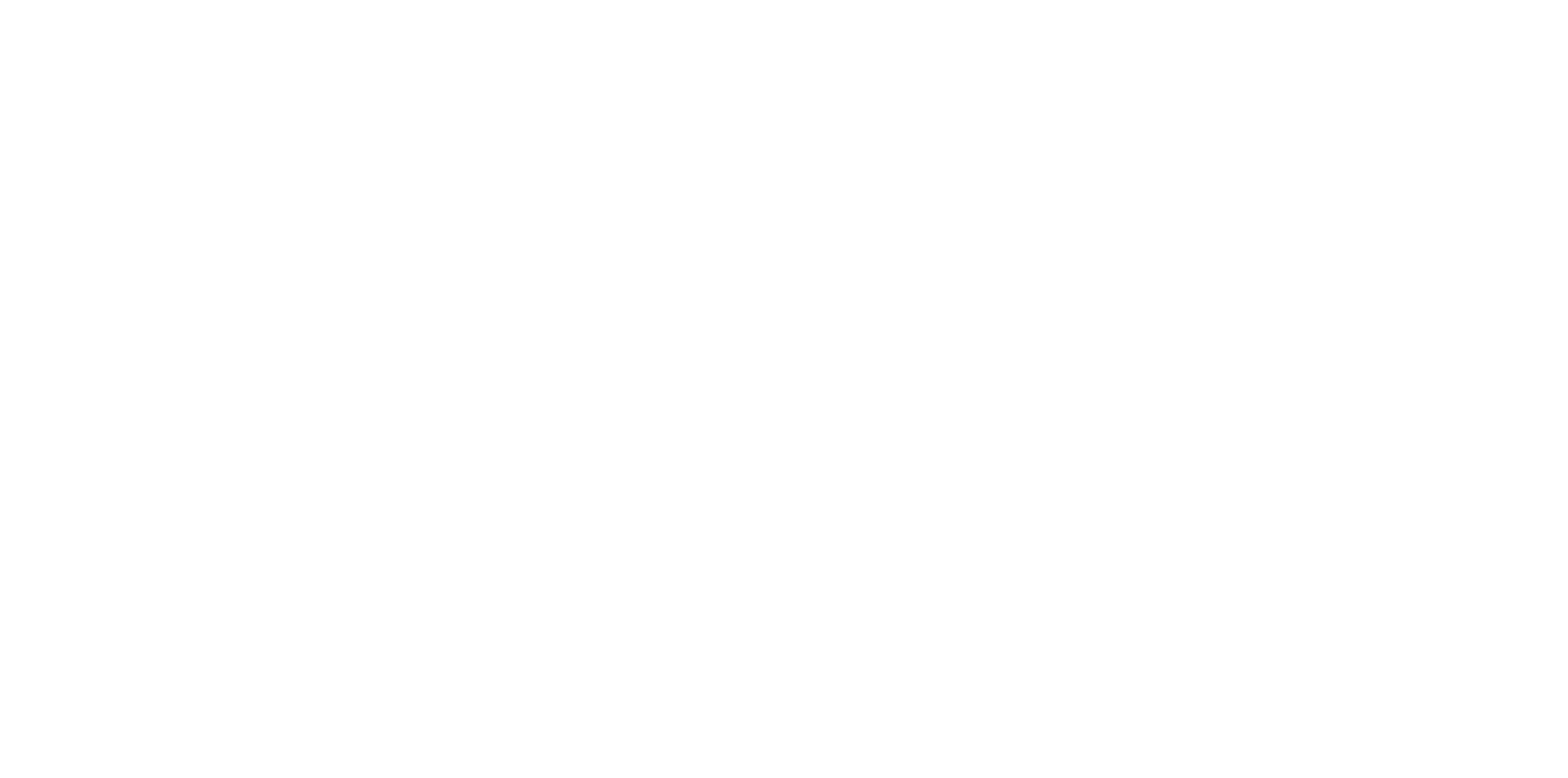
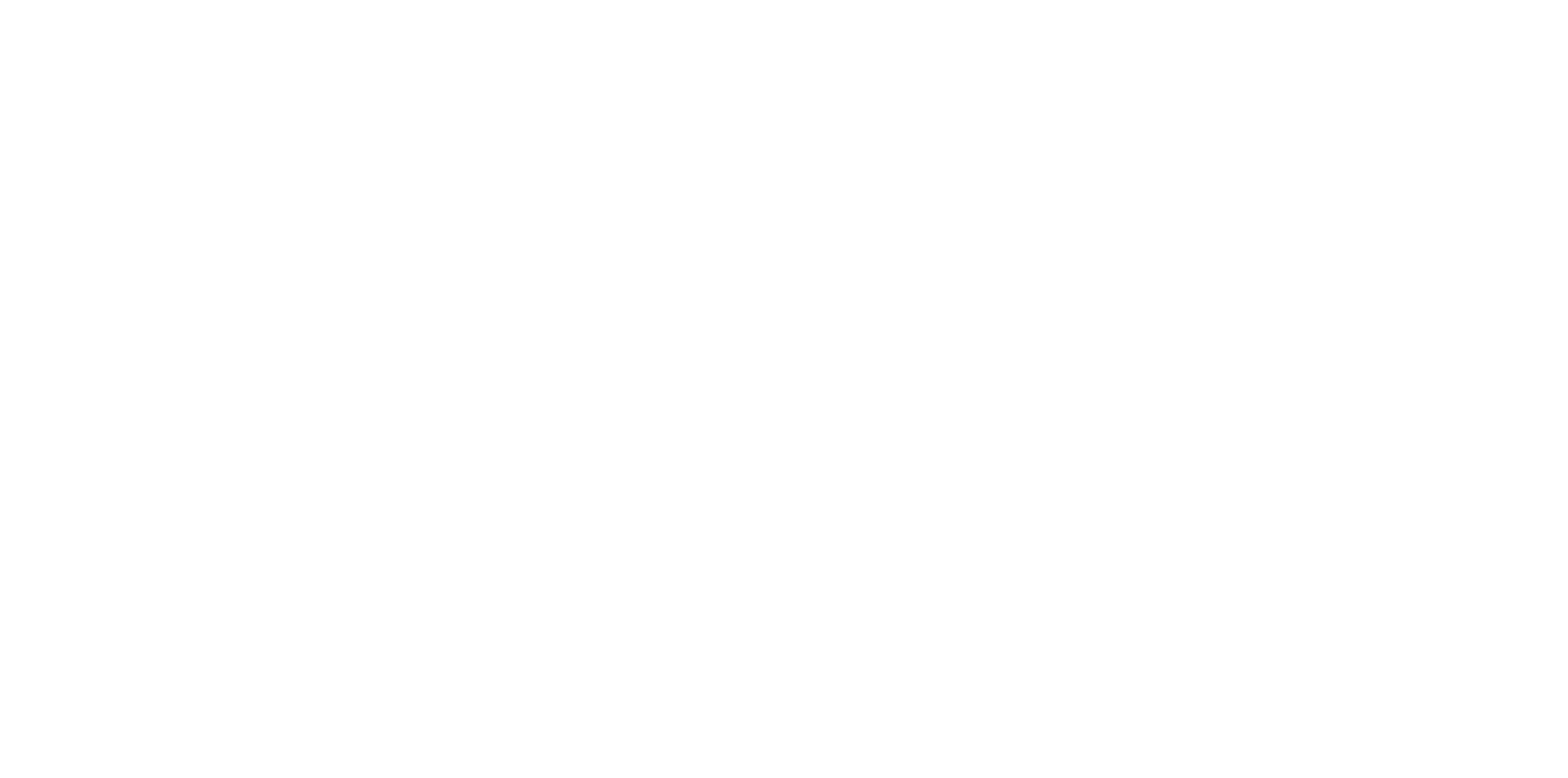
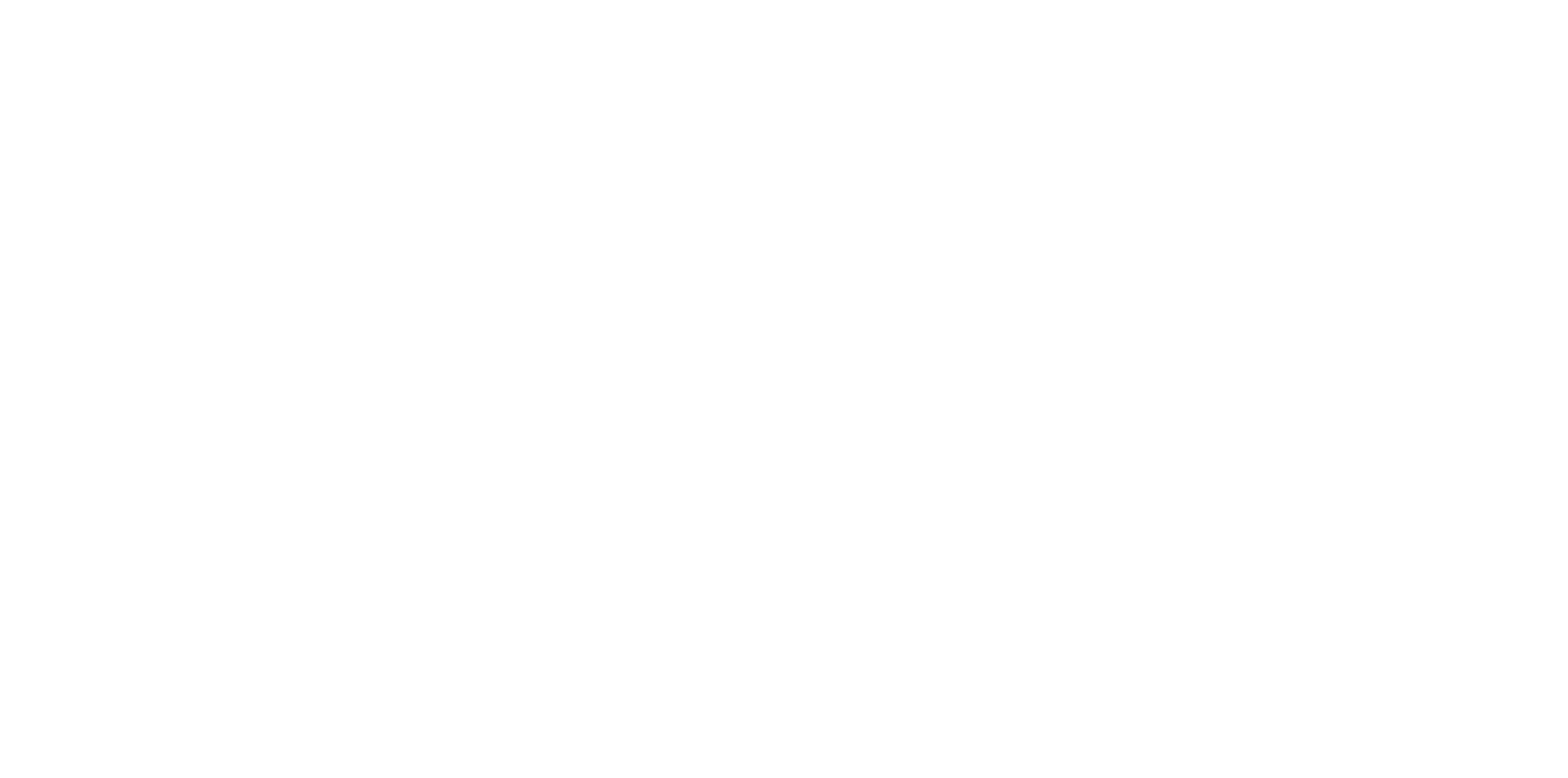
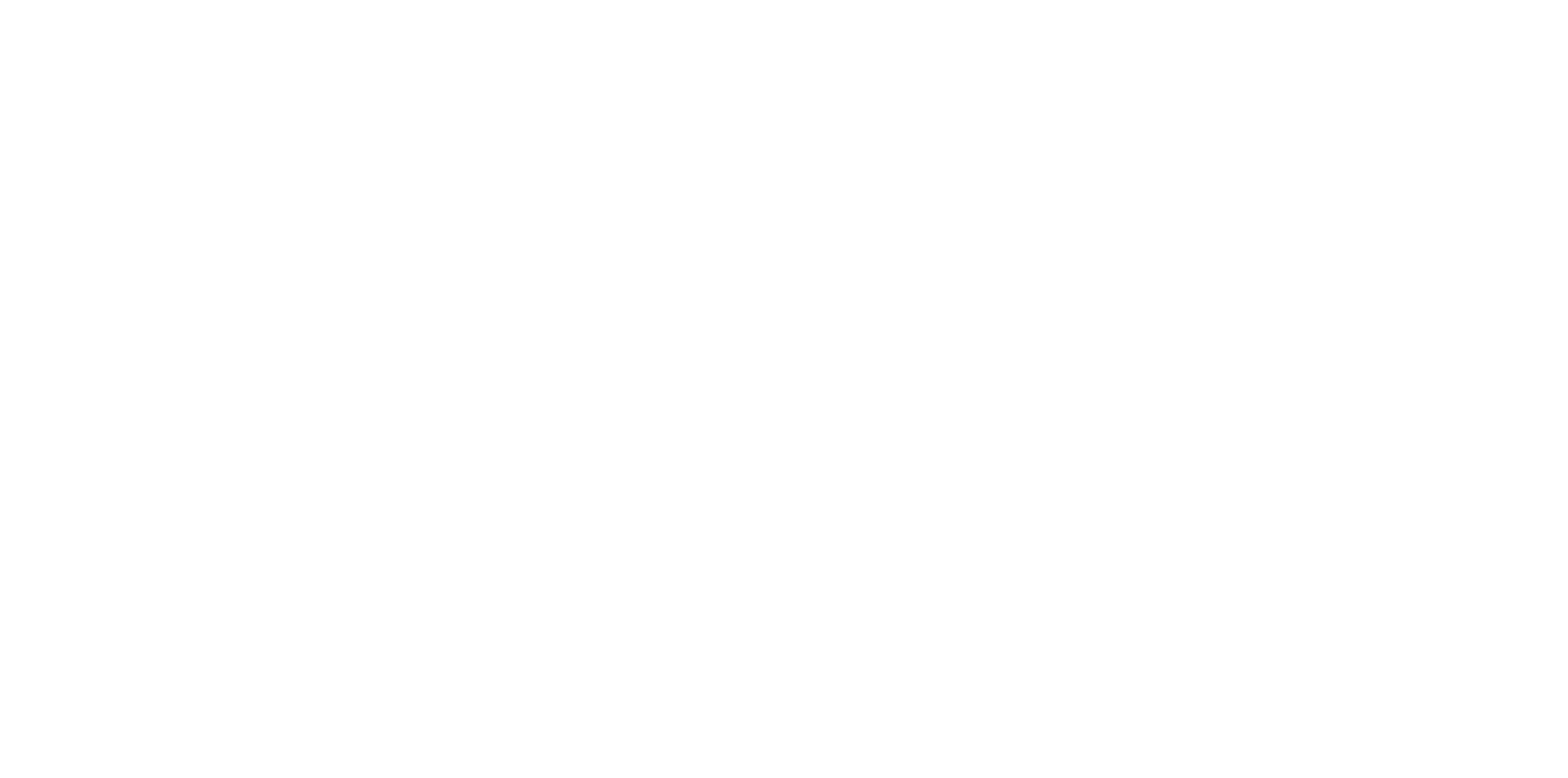








Data Context
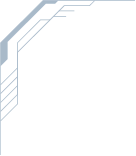
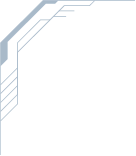
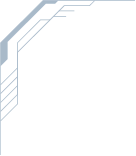
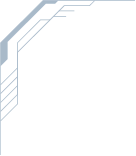
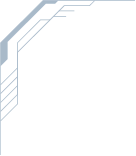
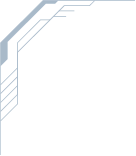
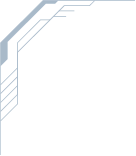
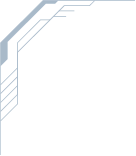
Integrate Data Context
TRAIN derives causal relationships from IT and OT data, structuring them into a Structural Causal Model (SCM). The SCM provides context for the broader causal models (PCM and RCM). TRAIN then merges these models into a Combined Causal Model (CCM) that focuses on the specific causal hypotheses relevant to the use case.










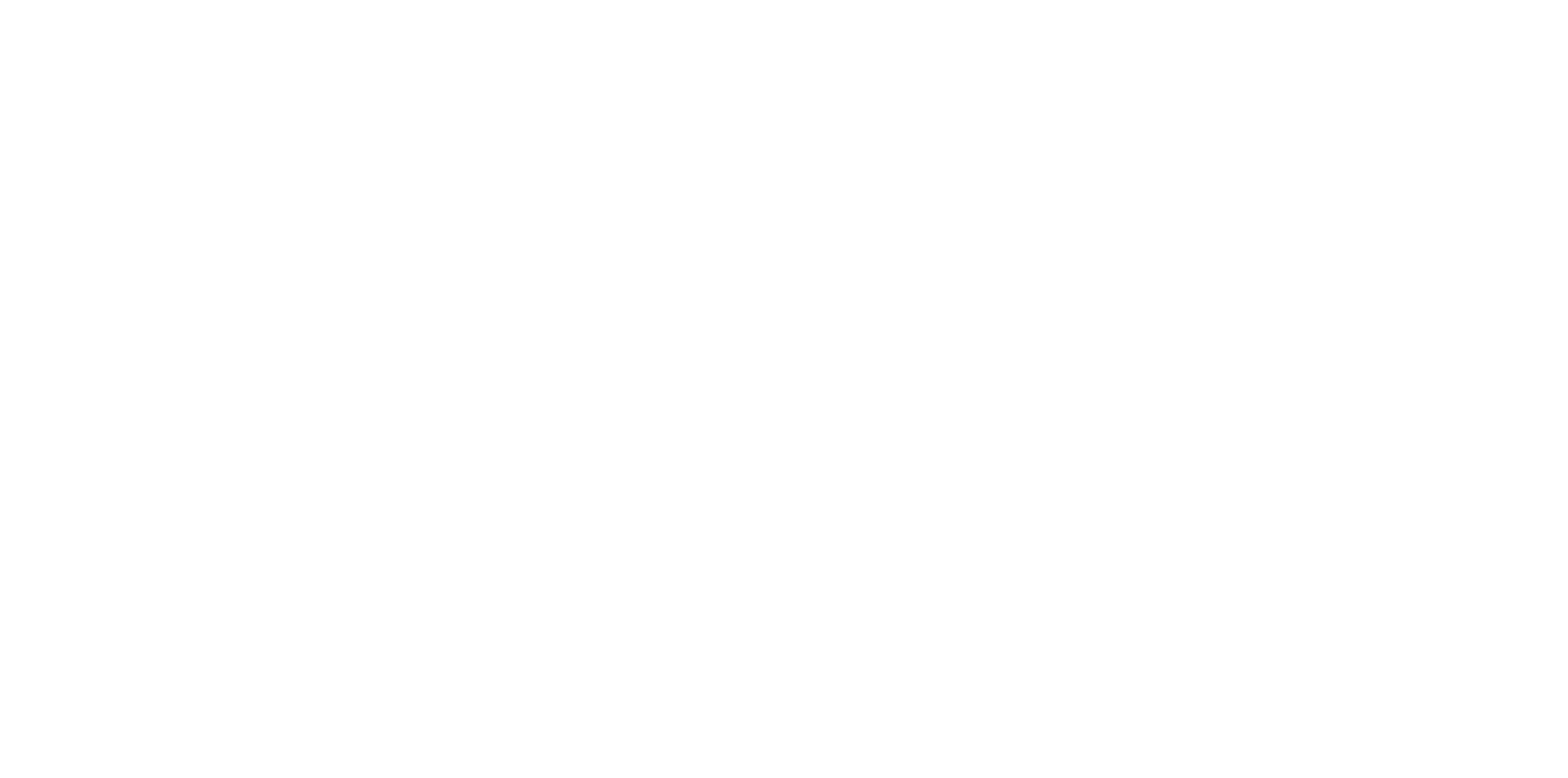
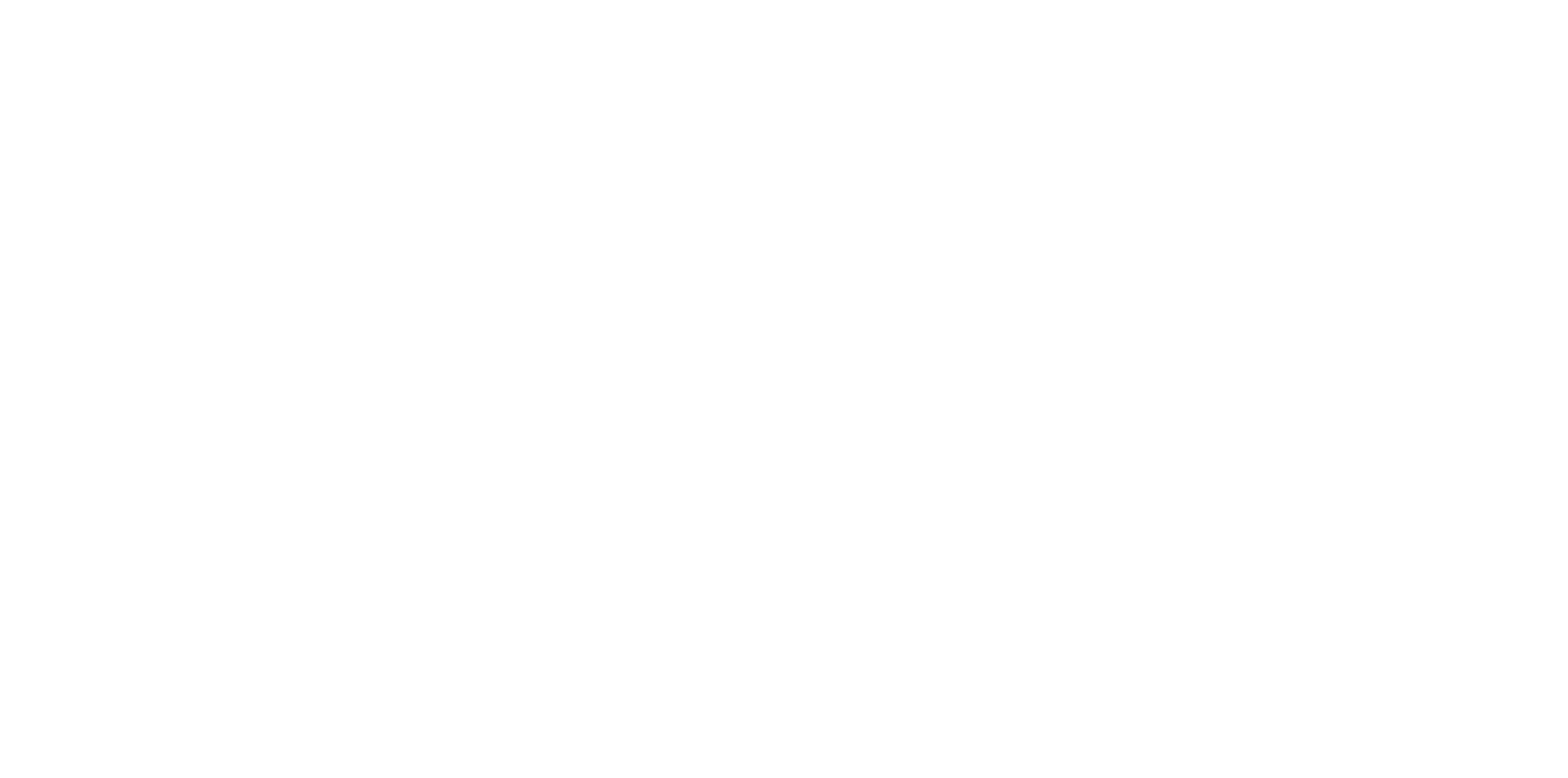
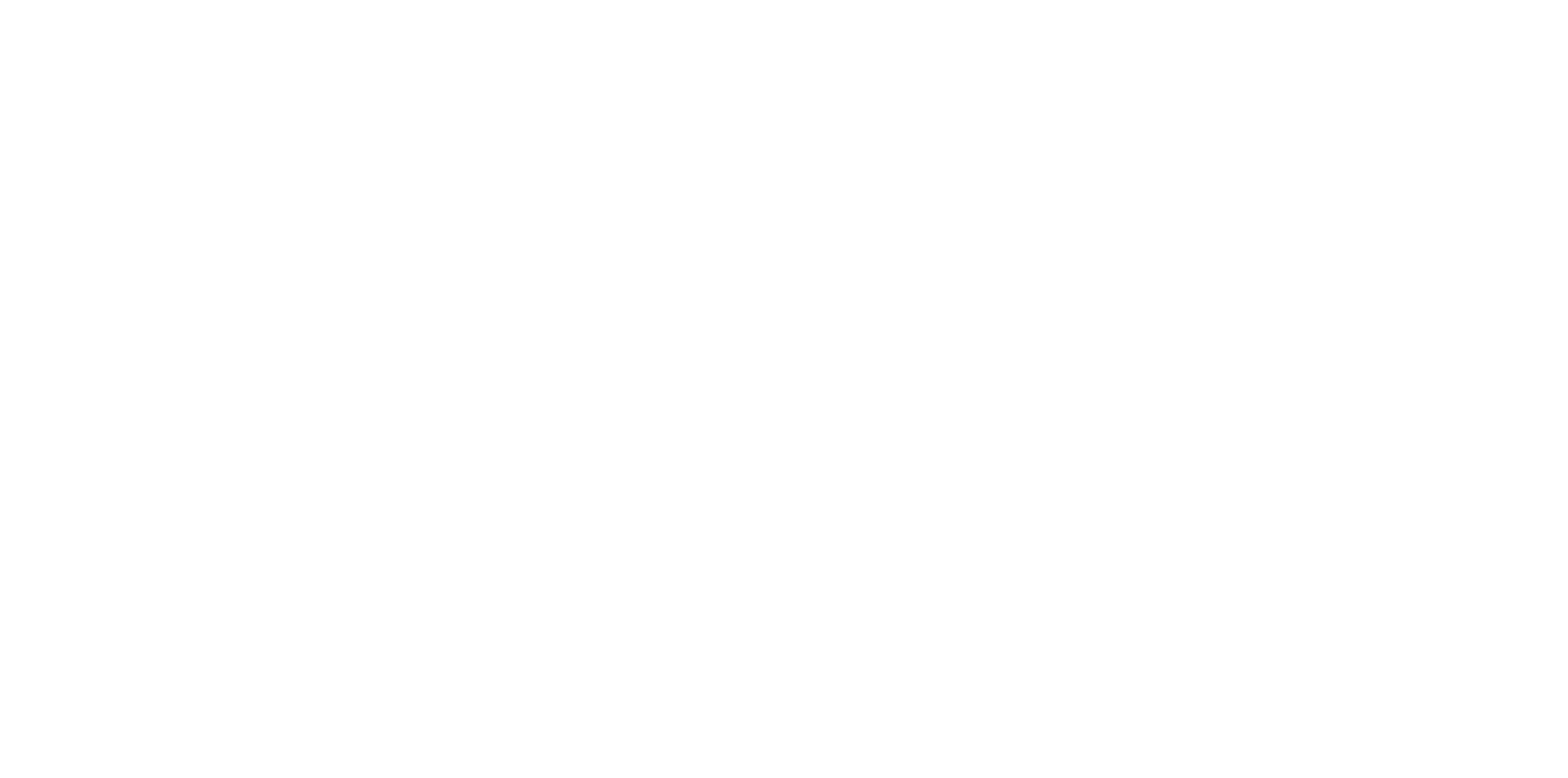
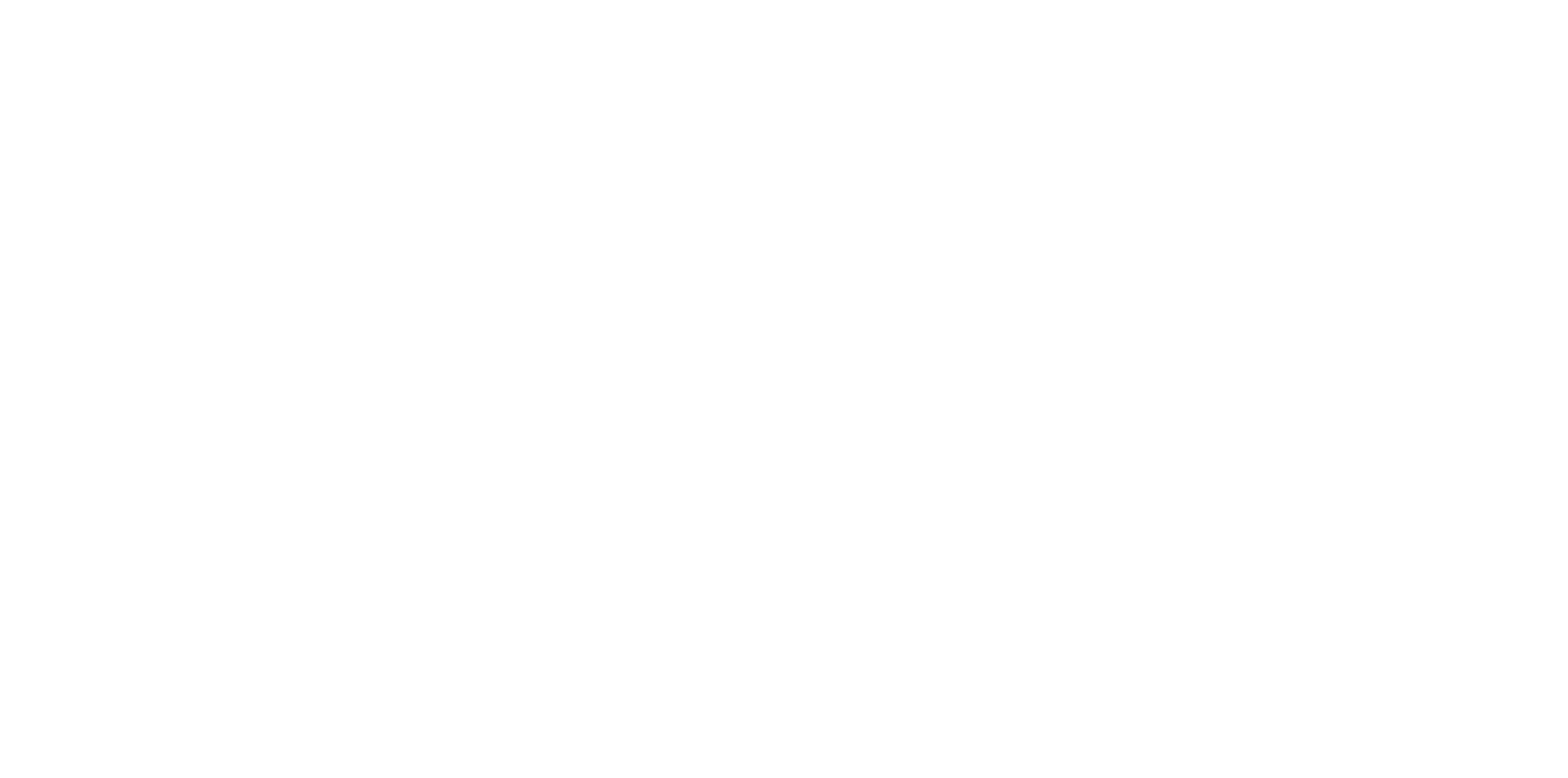
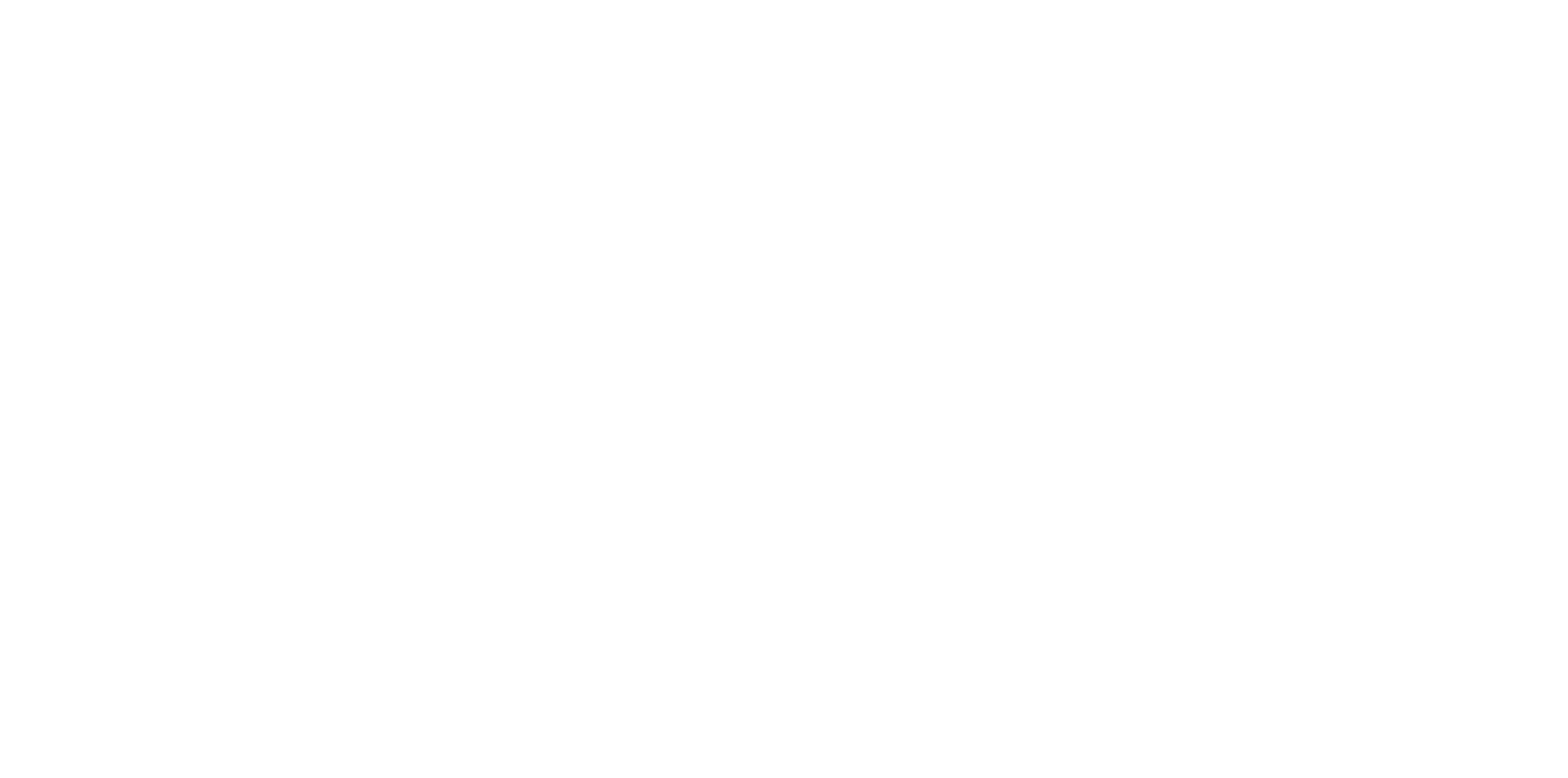
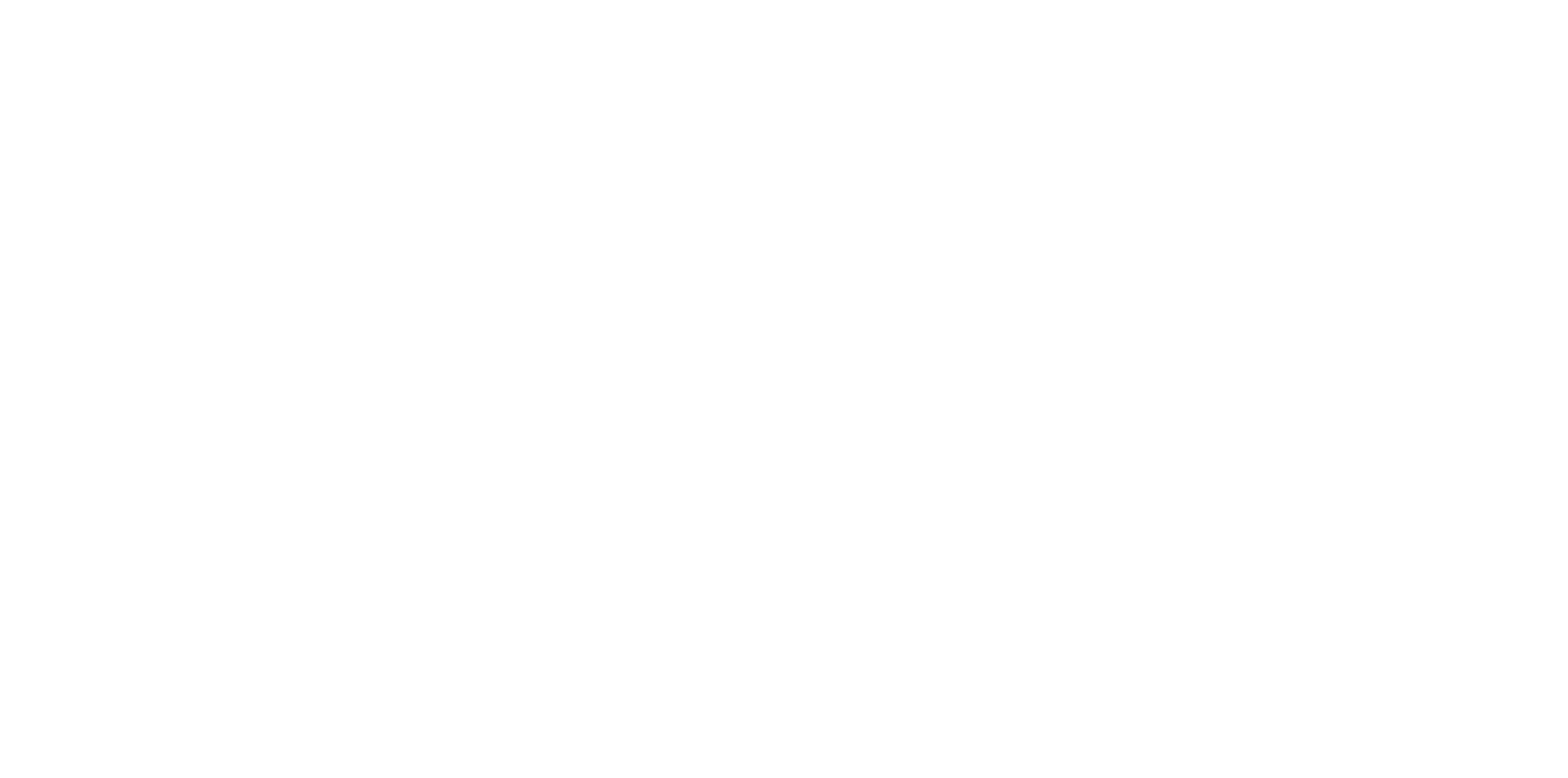
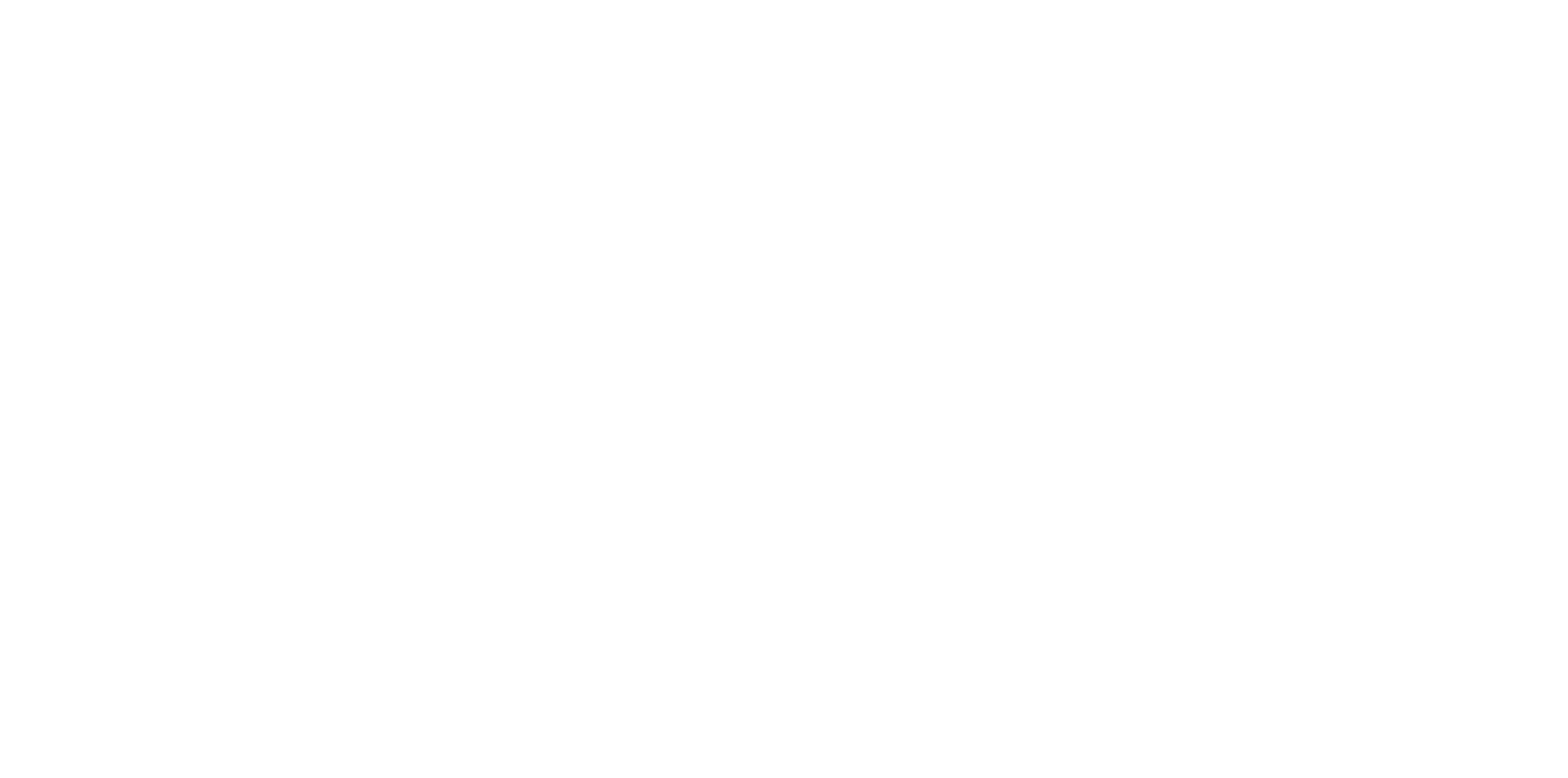
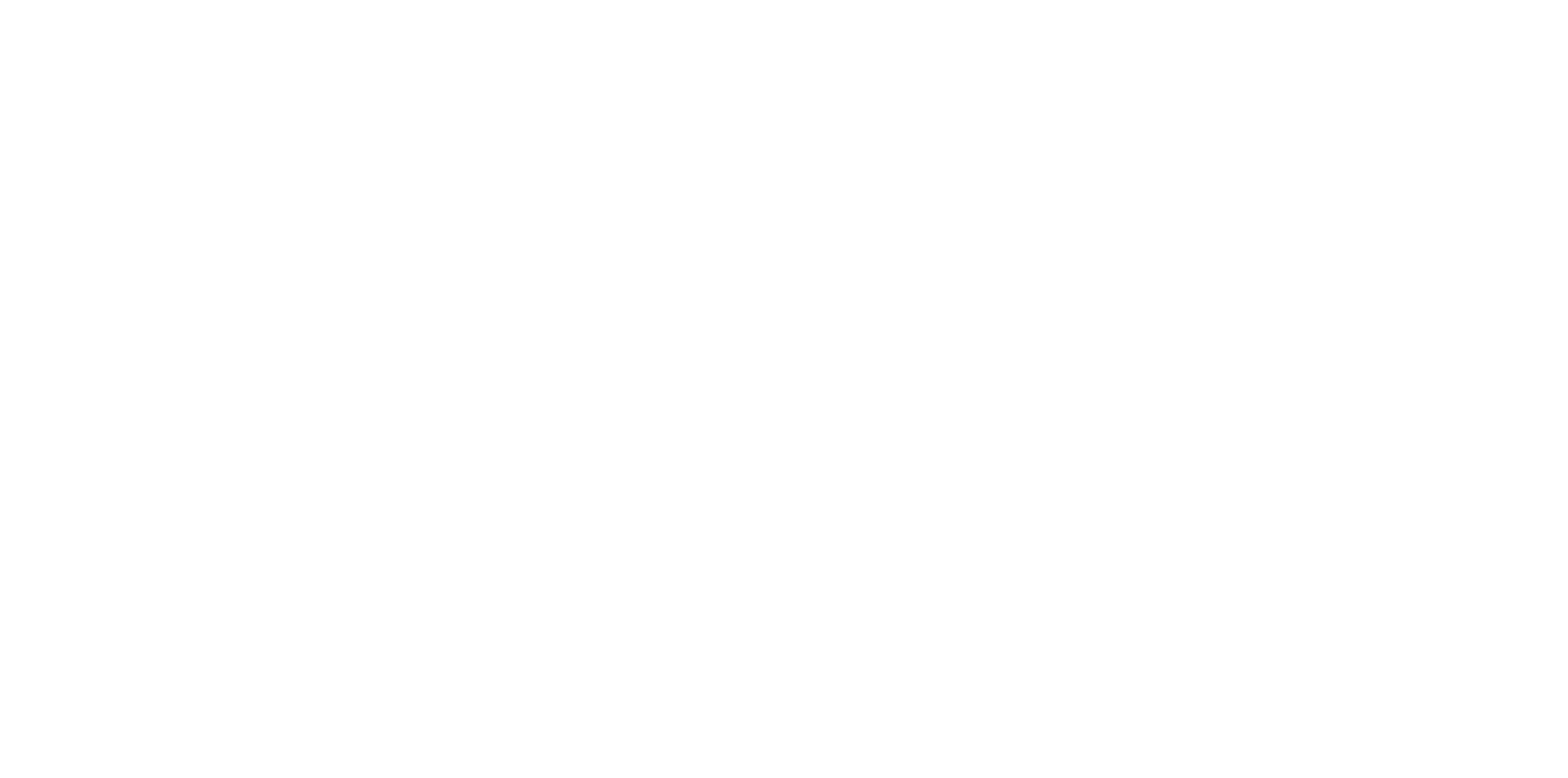
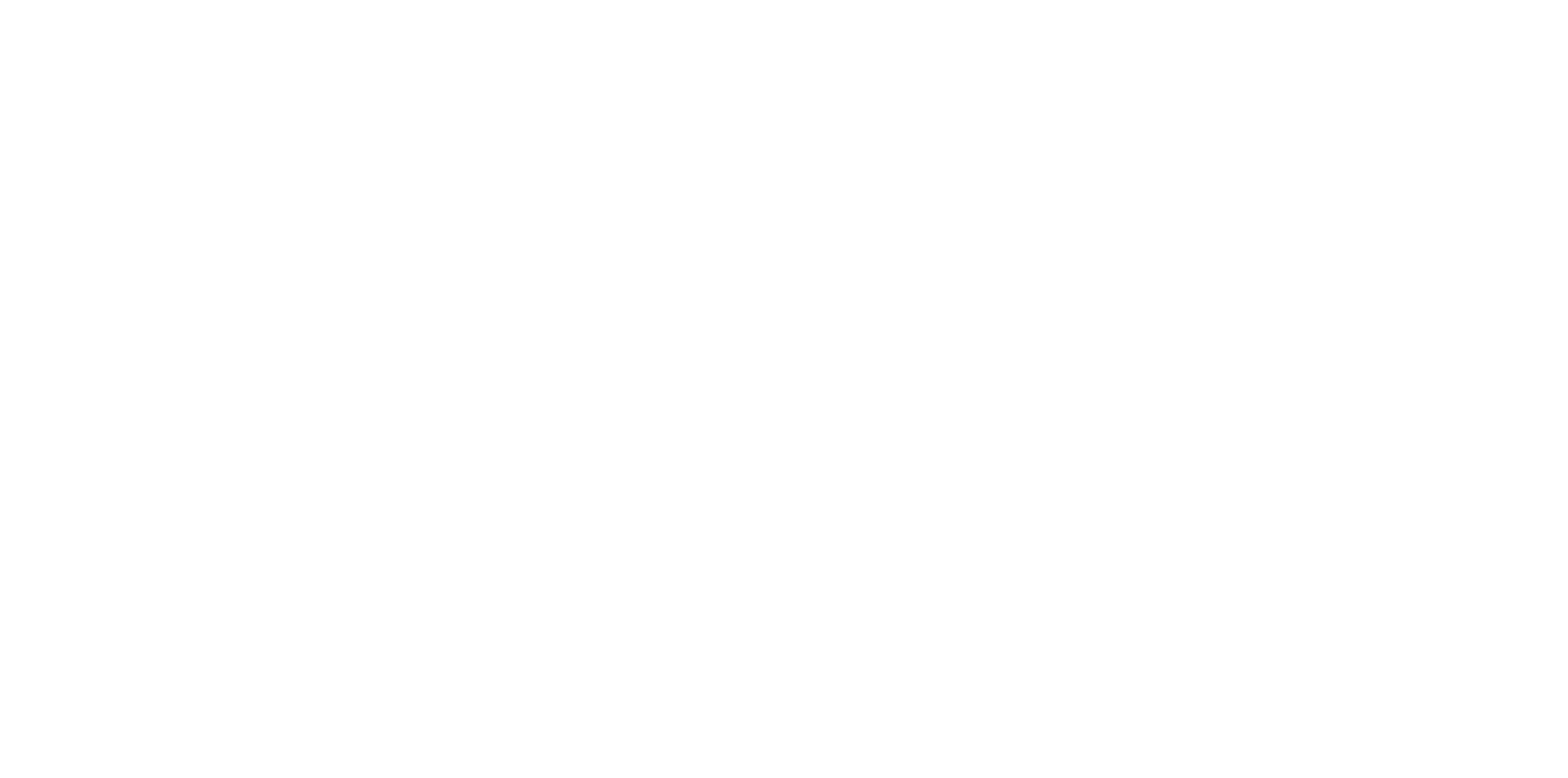
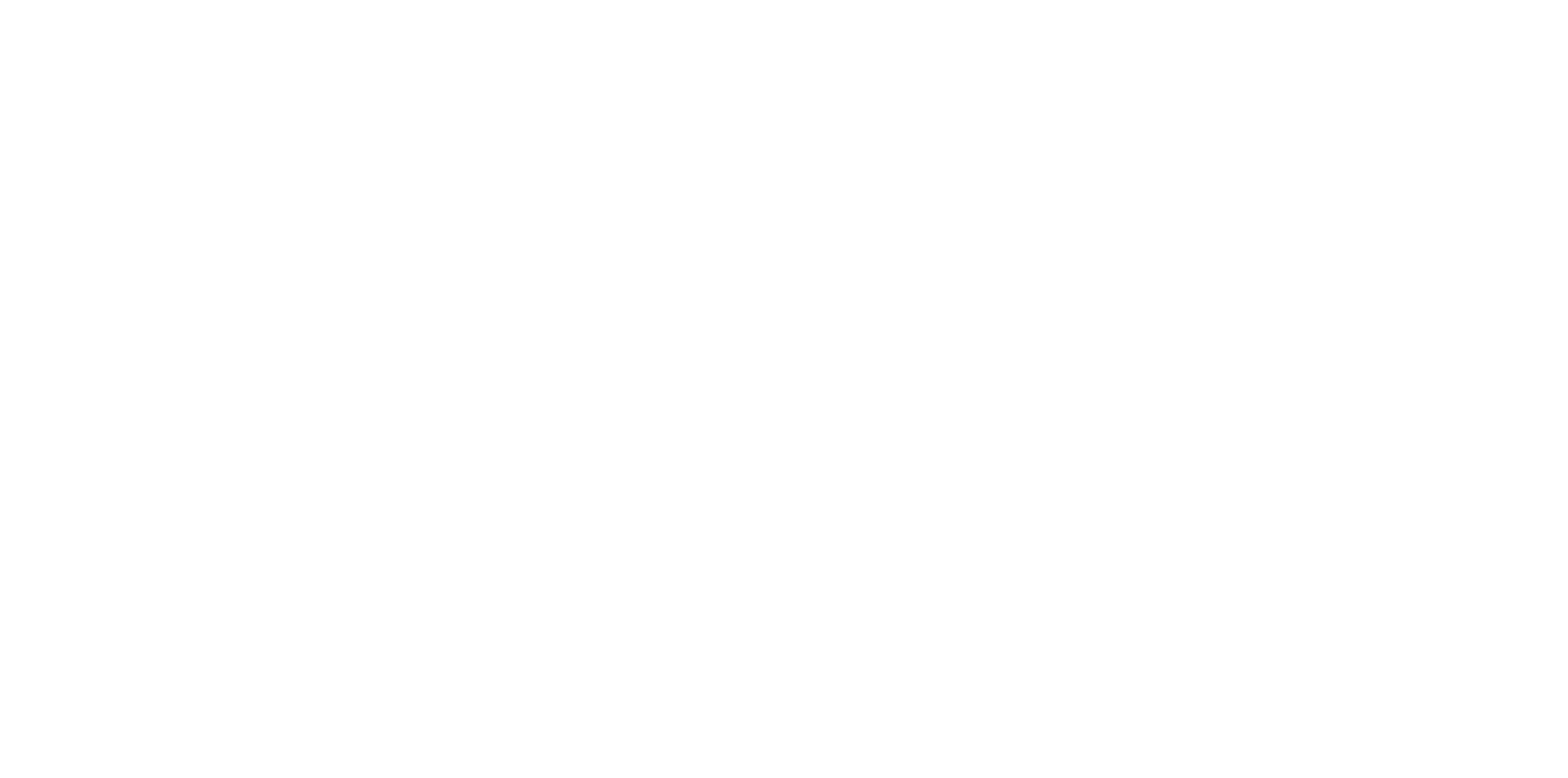








Causal Analysis
Causal Analysis module within TRAIN lets the users to apply causal models and
contextualized hypotheses to make complex decisions
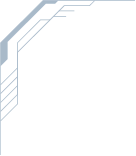
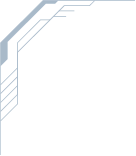
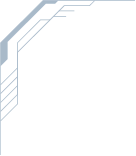
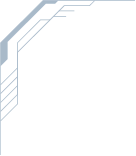
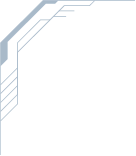
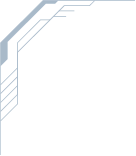
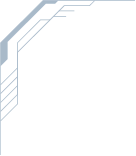
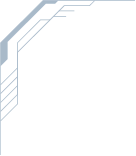
Simulation
Applying causal analysis on the learned causal model, TRAIN simulates various scenarios including counterfactual ones – to helps the user plan for scenarios, such as supplier disruption in a supply chain, or machines operating outside of usual conditions in manufacturing.










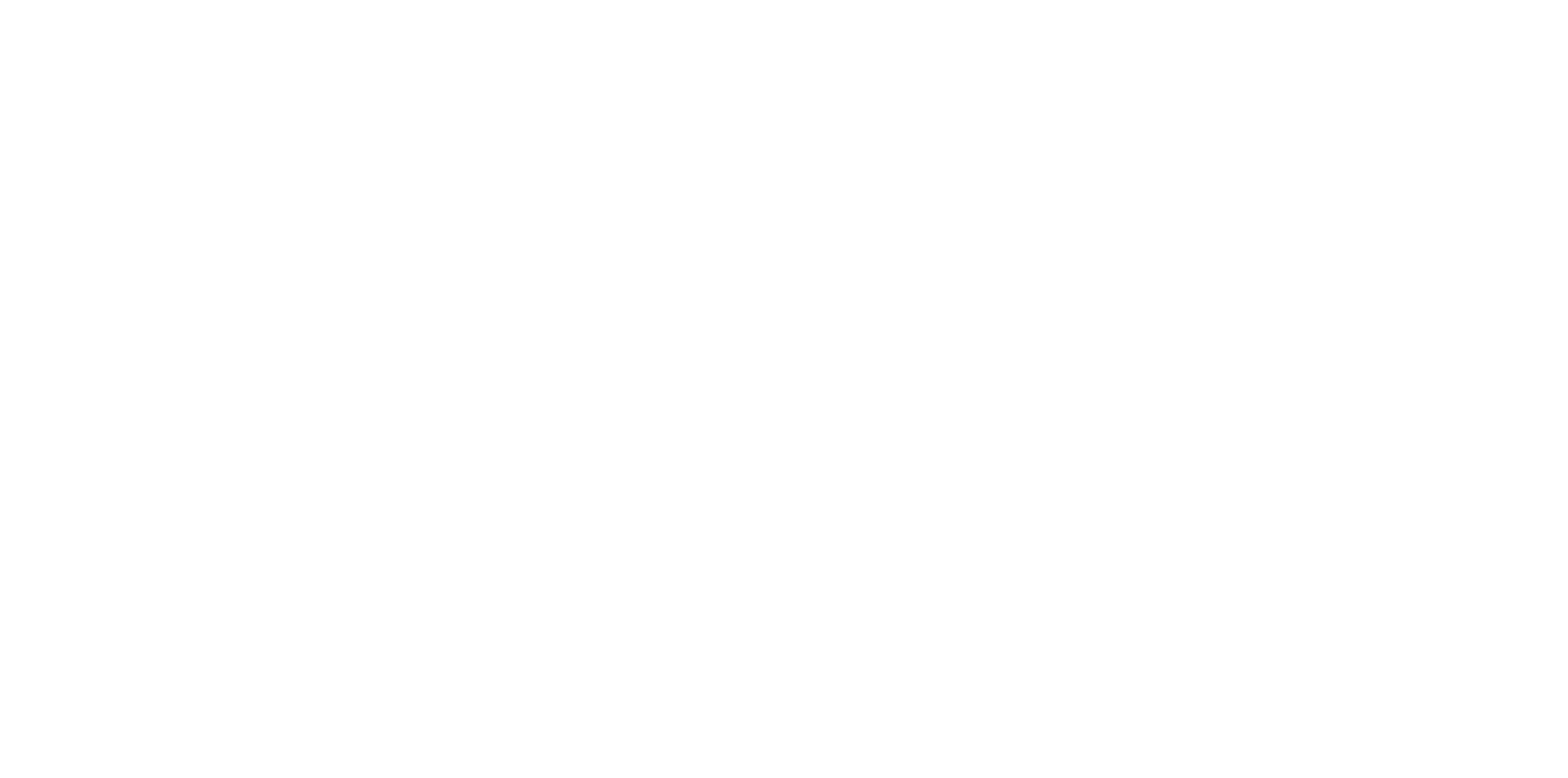
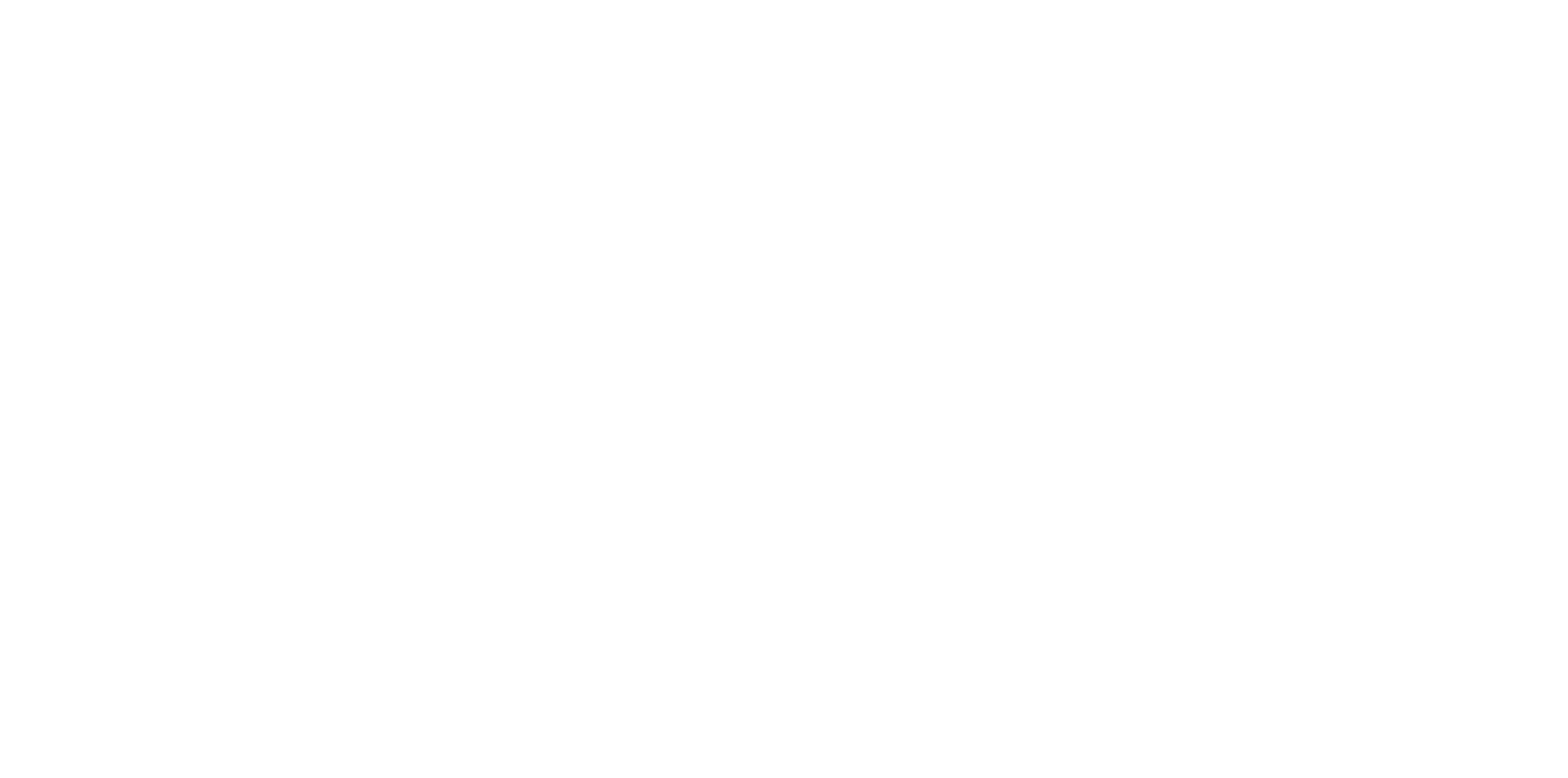
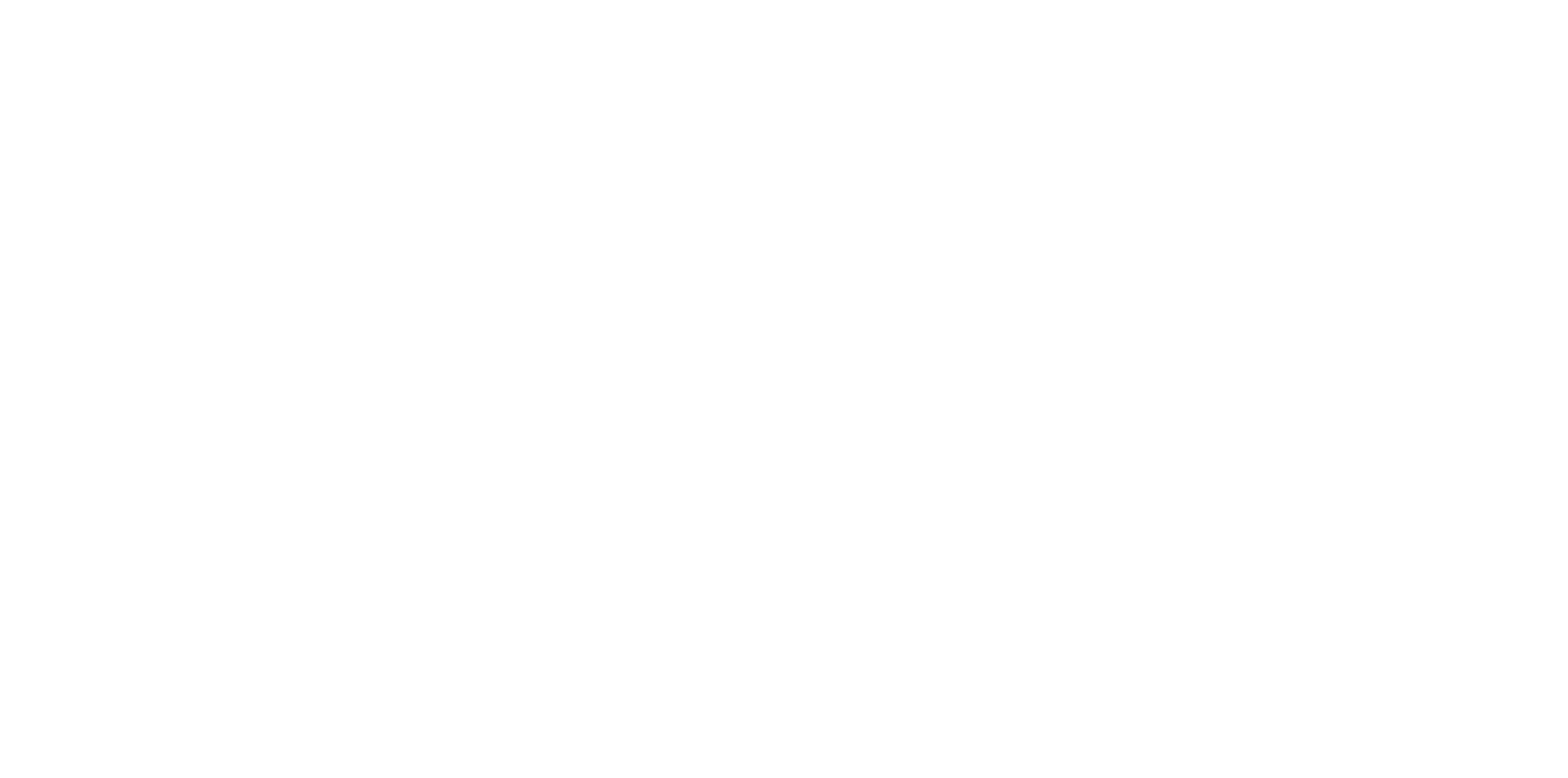
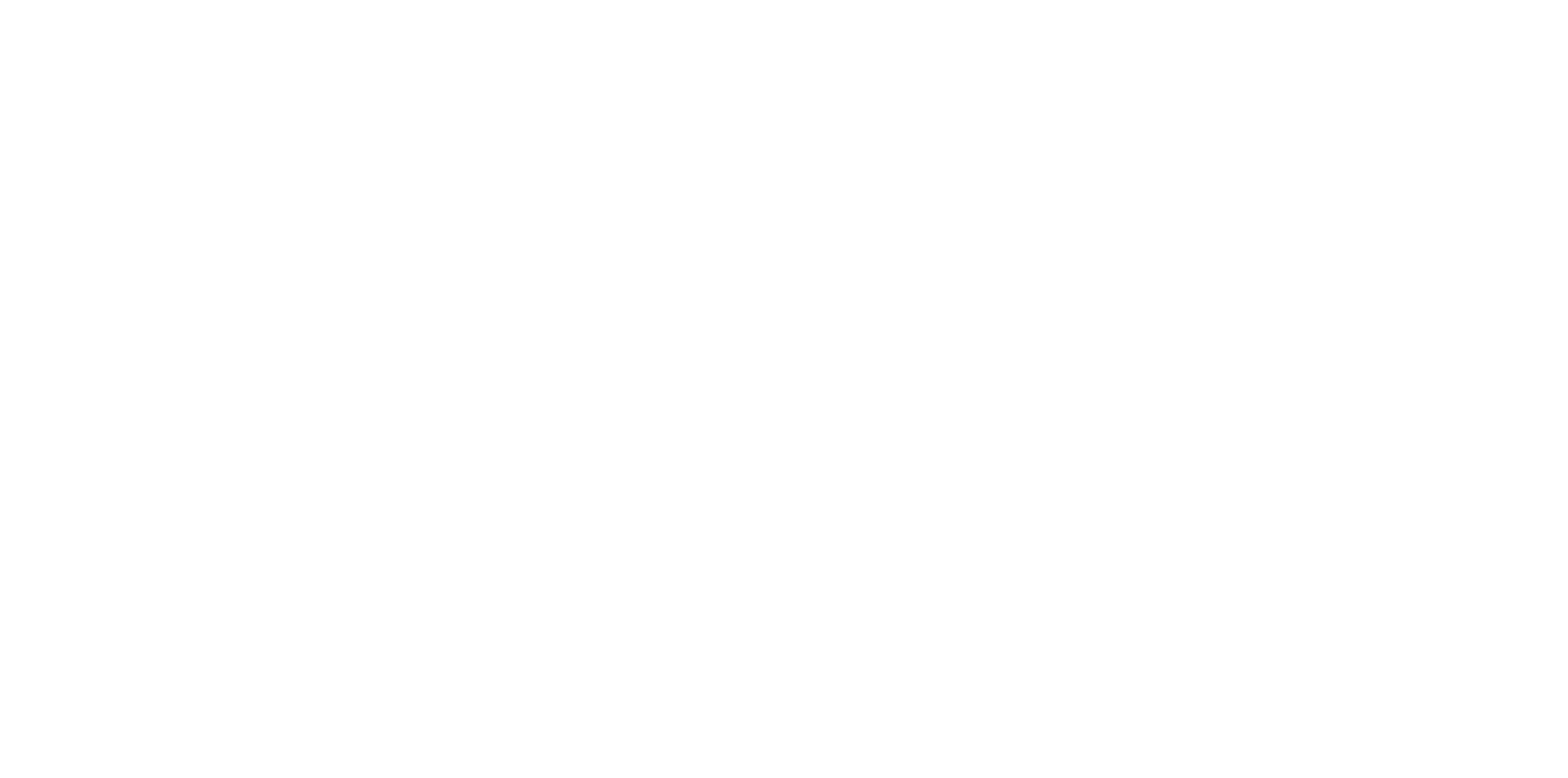
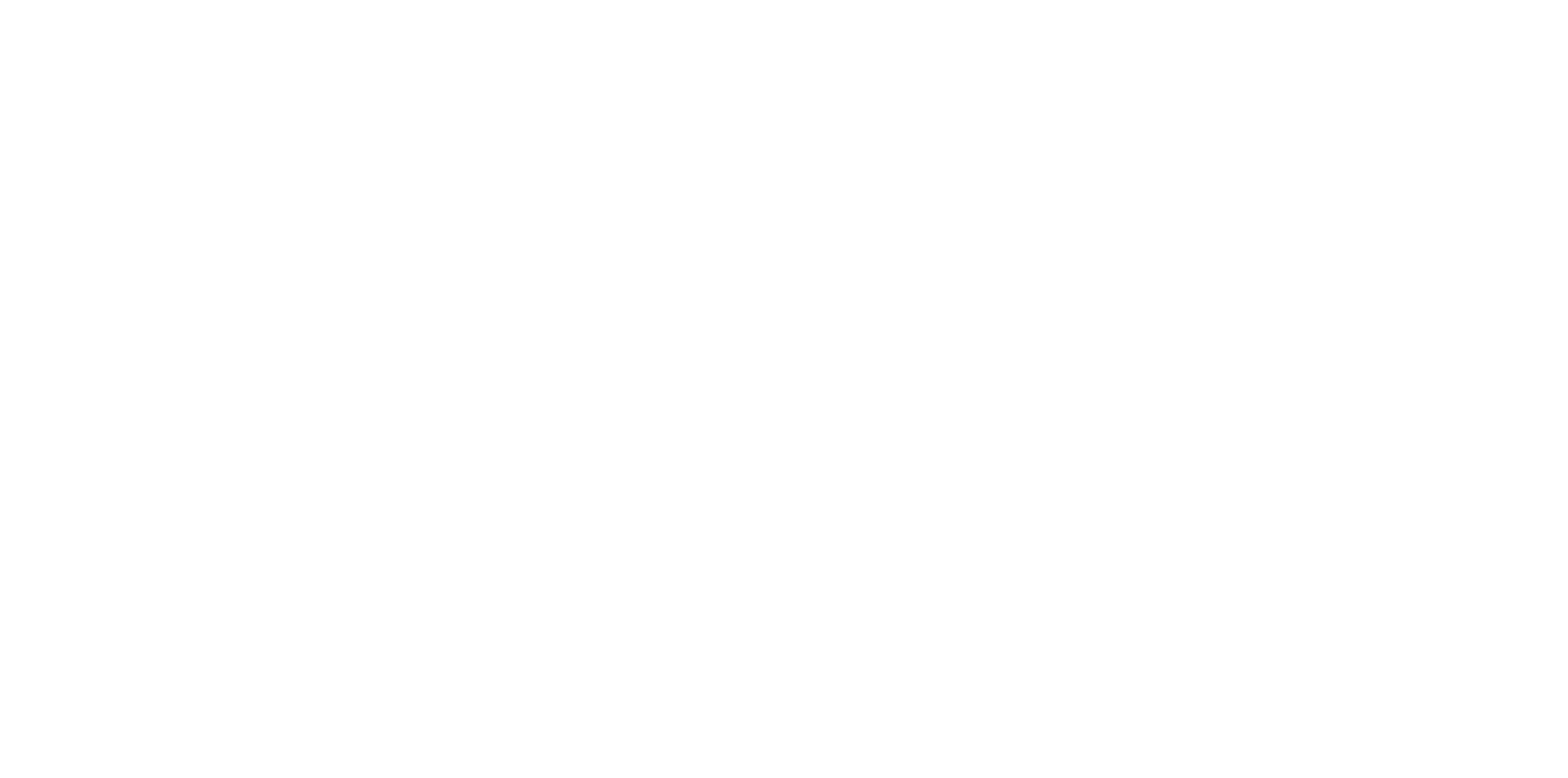
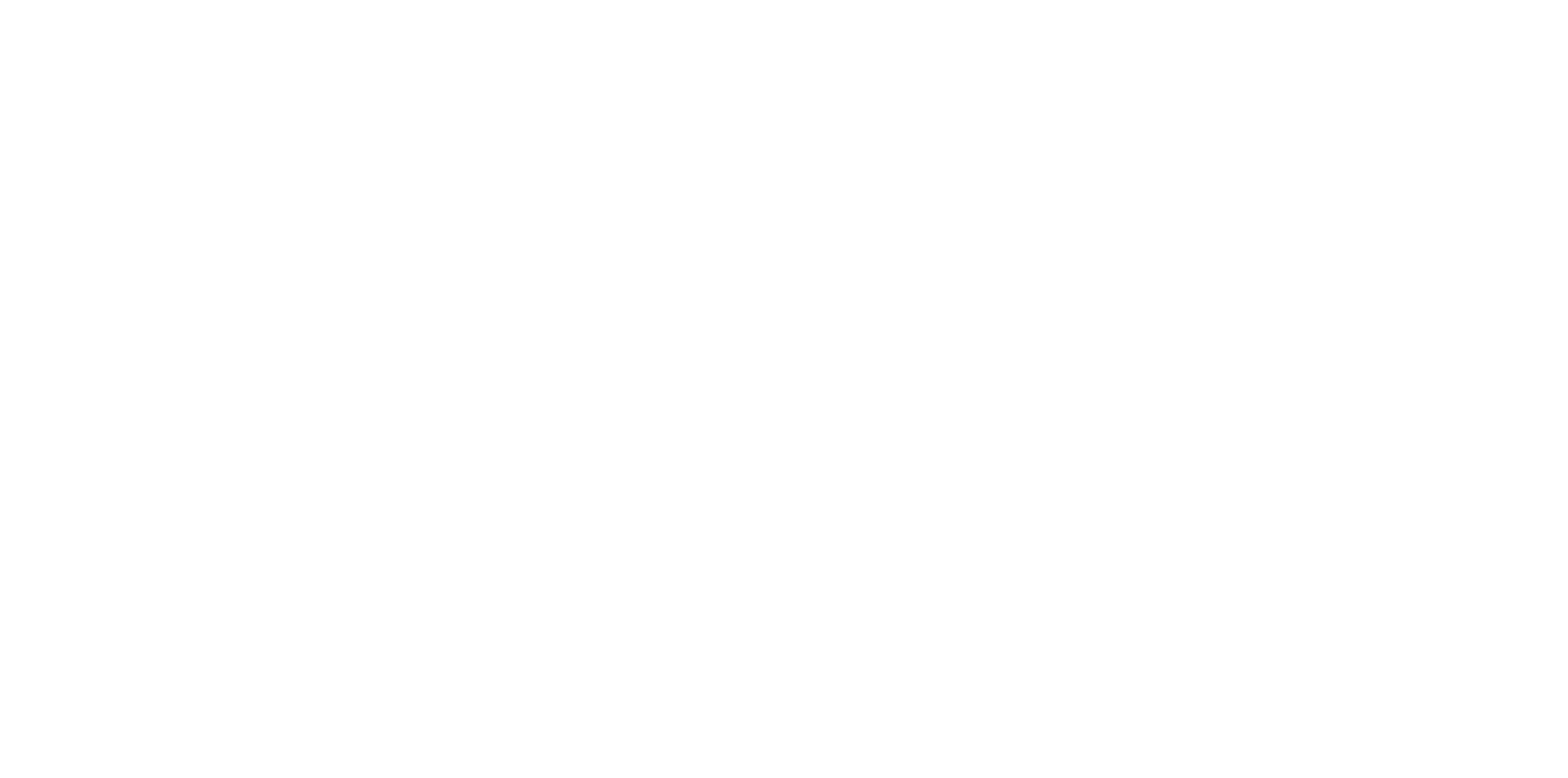
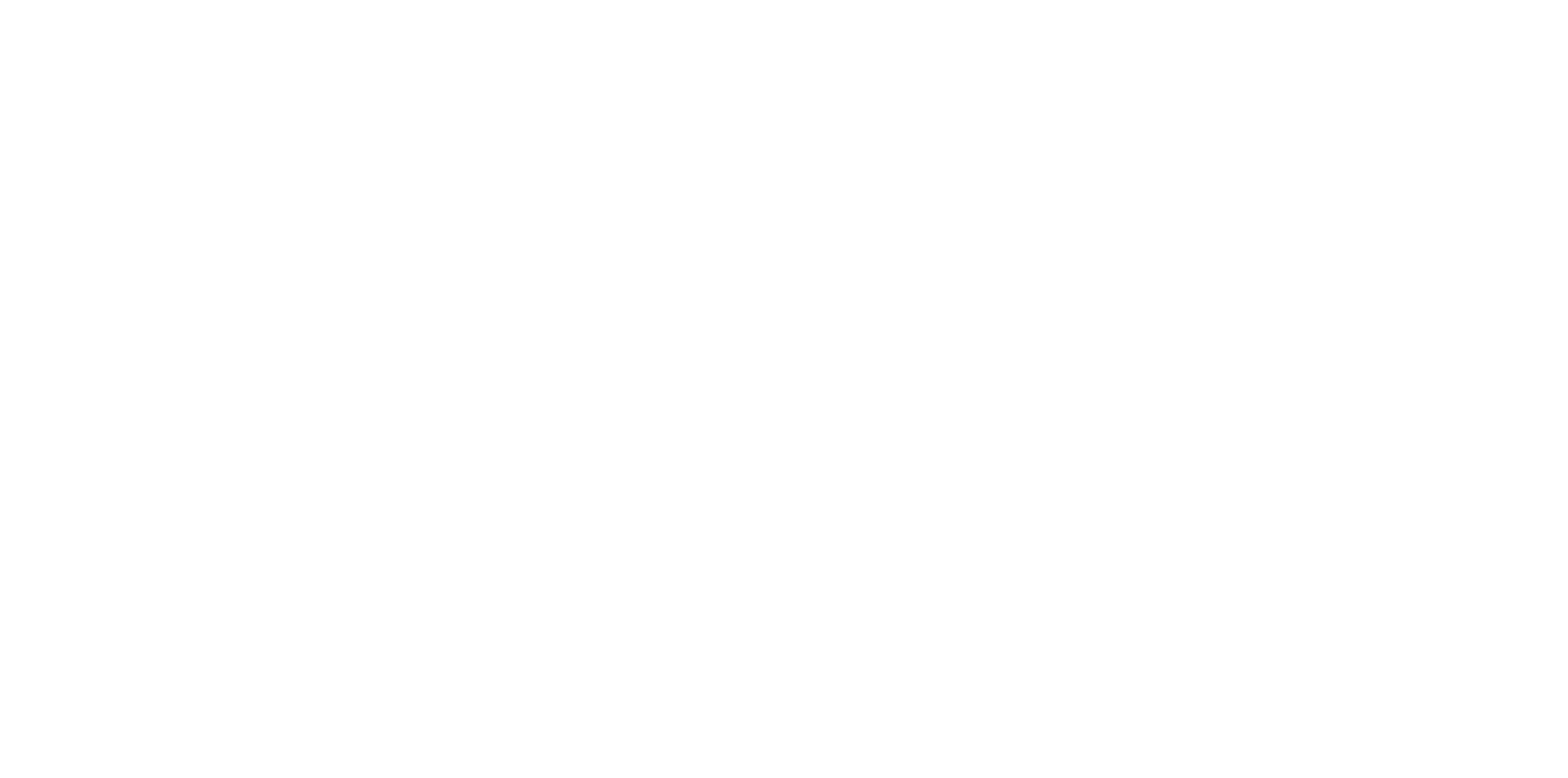
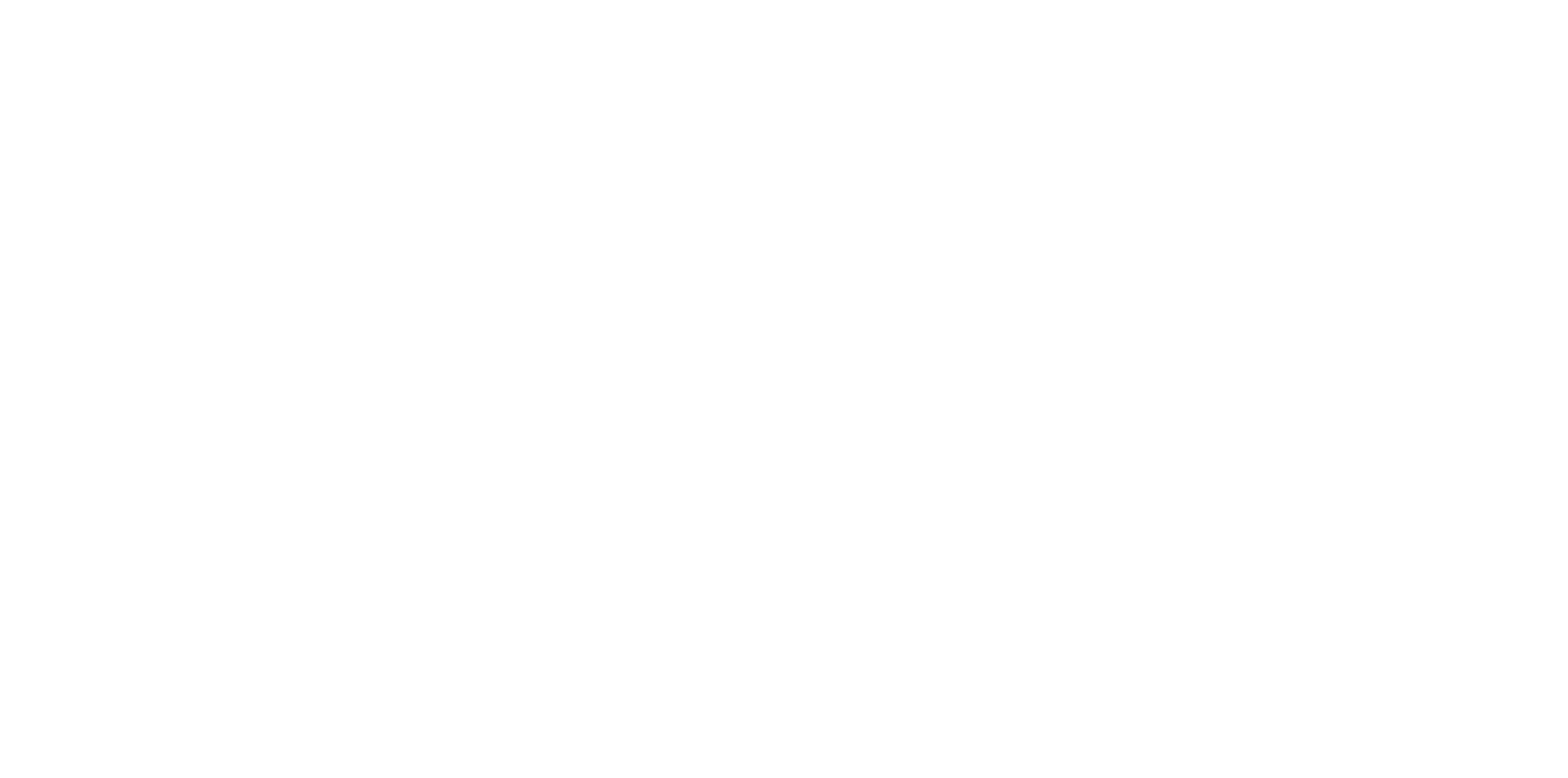
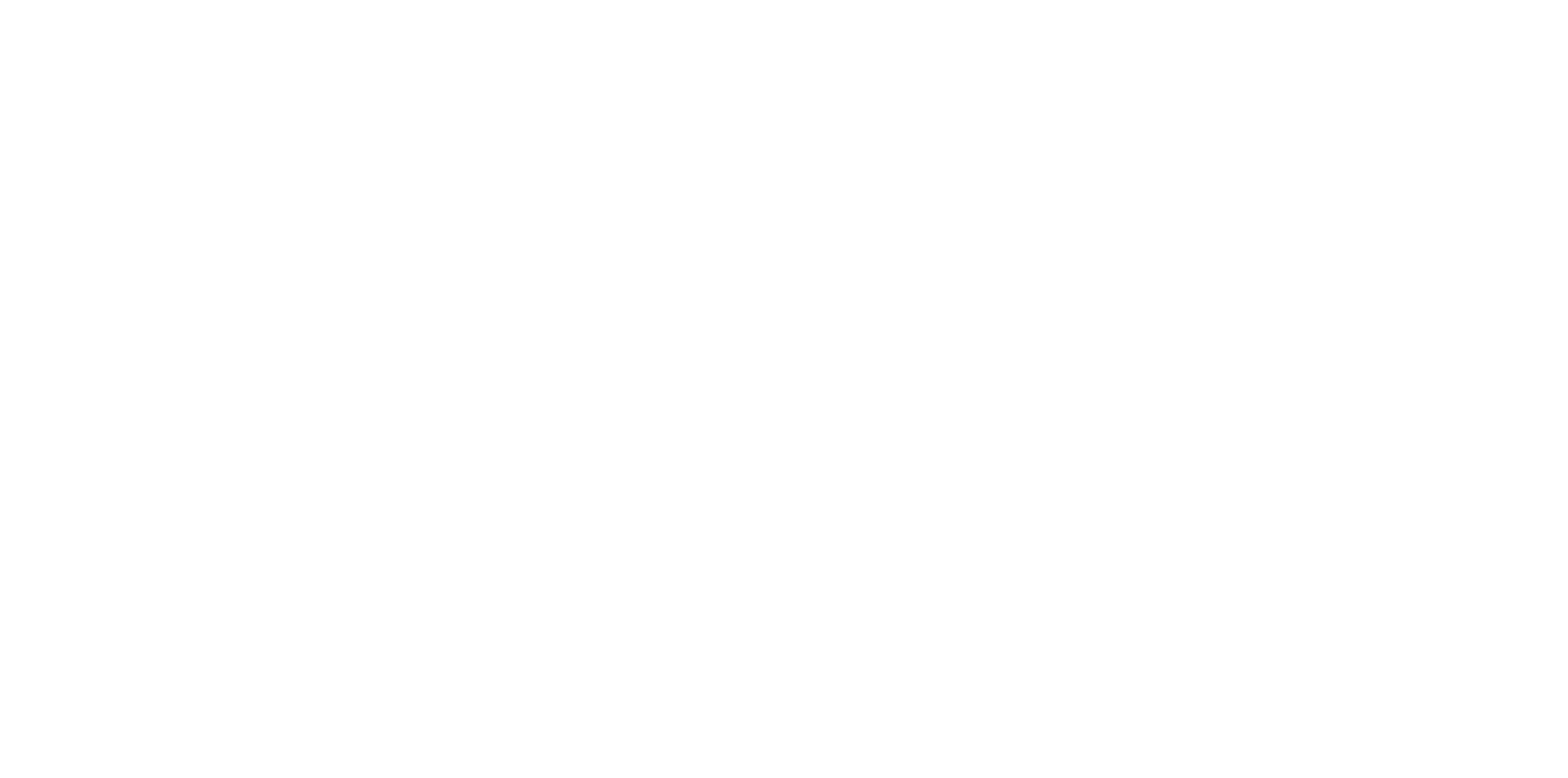
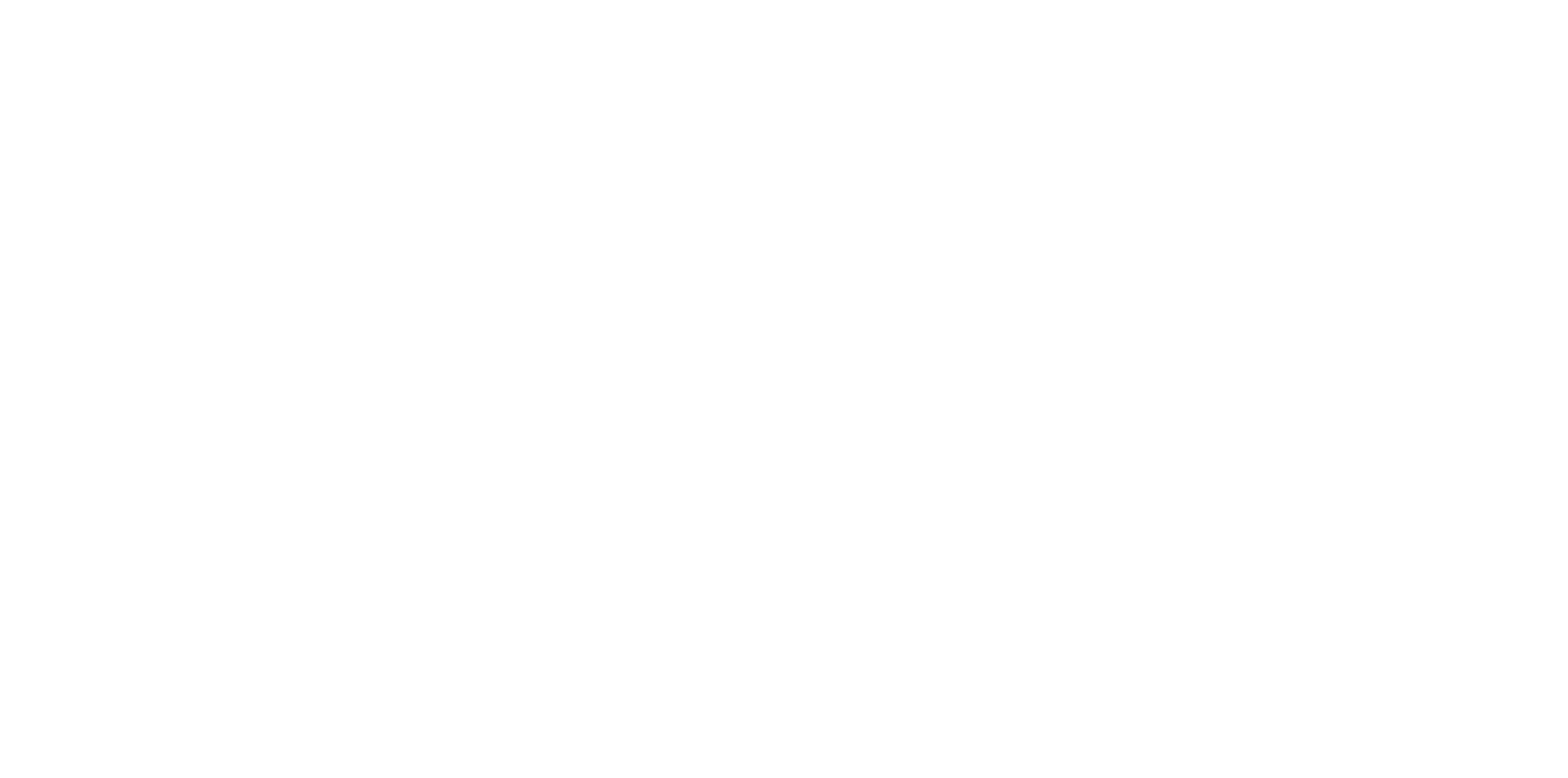








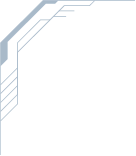
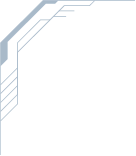
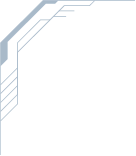
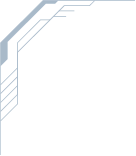
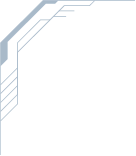
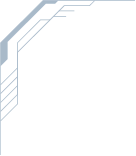
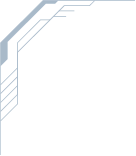
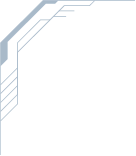
Optimization
TRAIN systematically assists users in configuring optimization models, including selecting suitable decision variables and implementing the right constraints for pre-defined objectives. This enables multi-objective optimization across various functions like supply chain and manufacturing operations, leveraging causal knowledge specific to each domain.










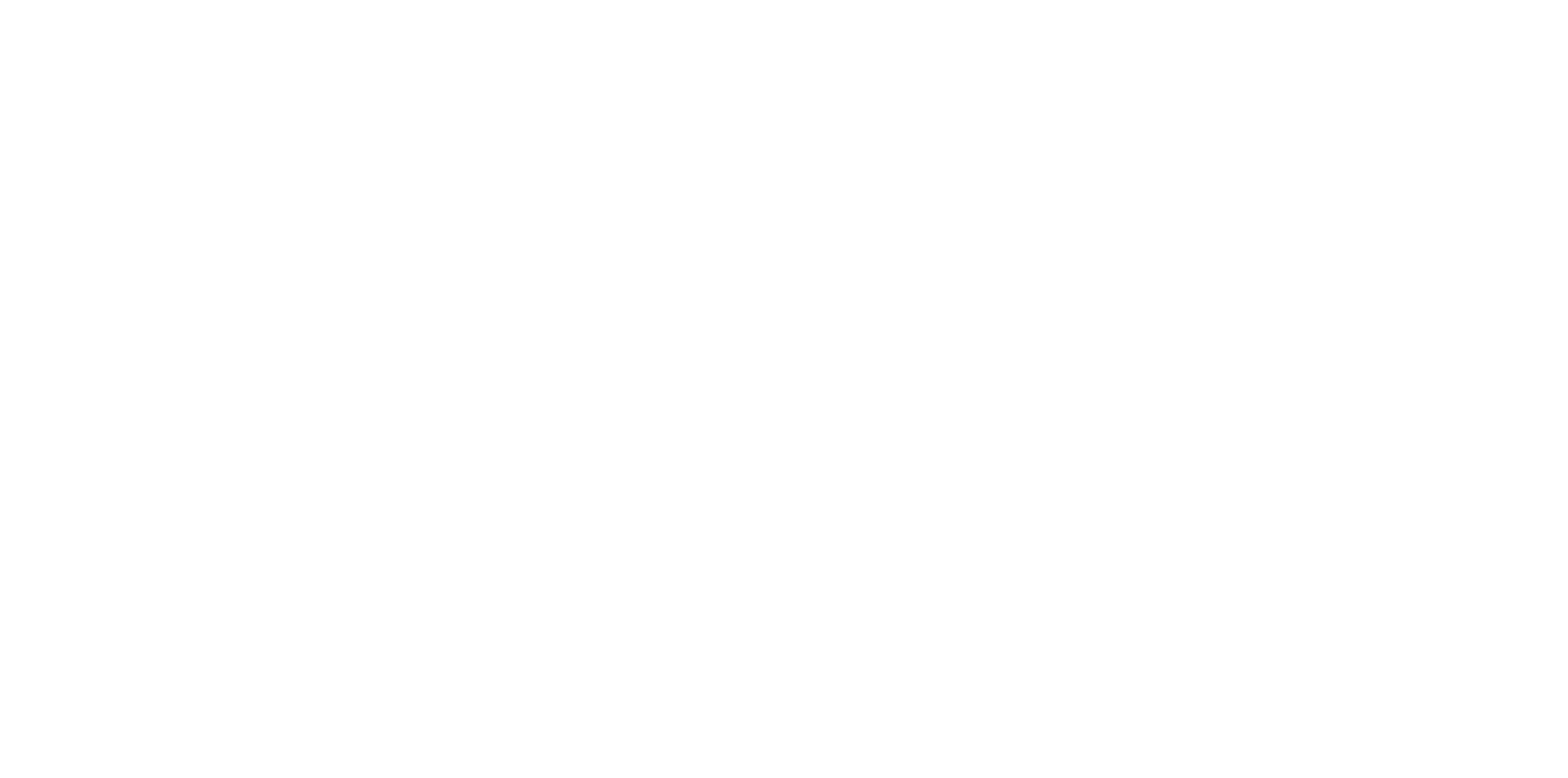
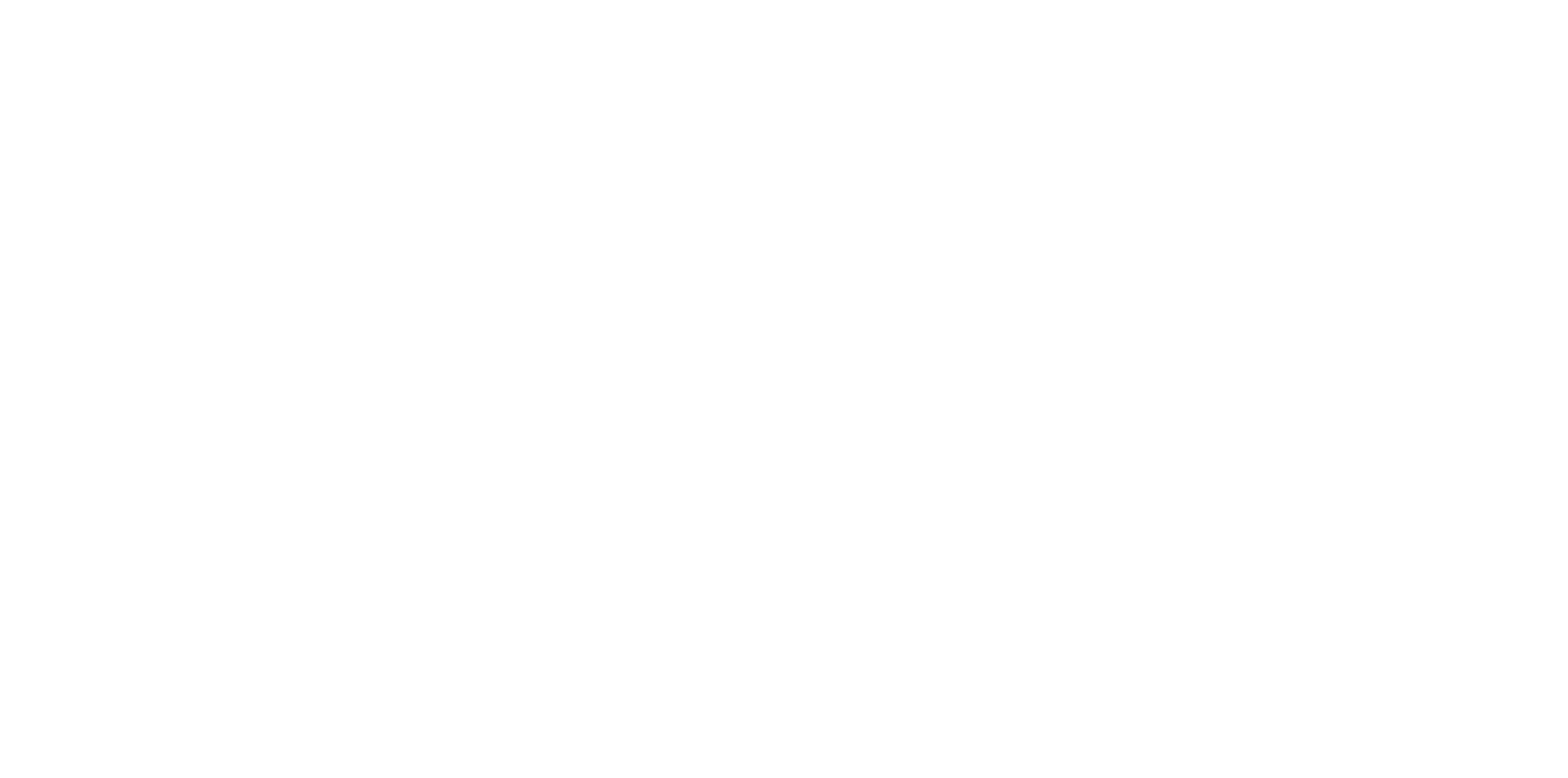
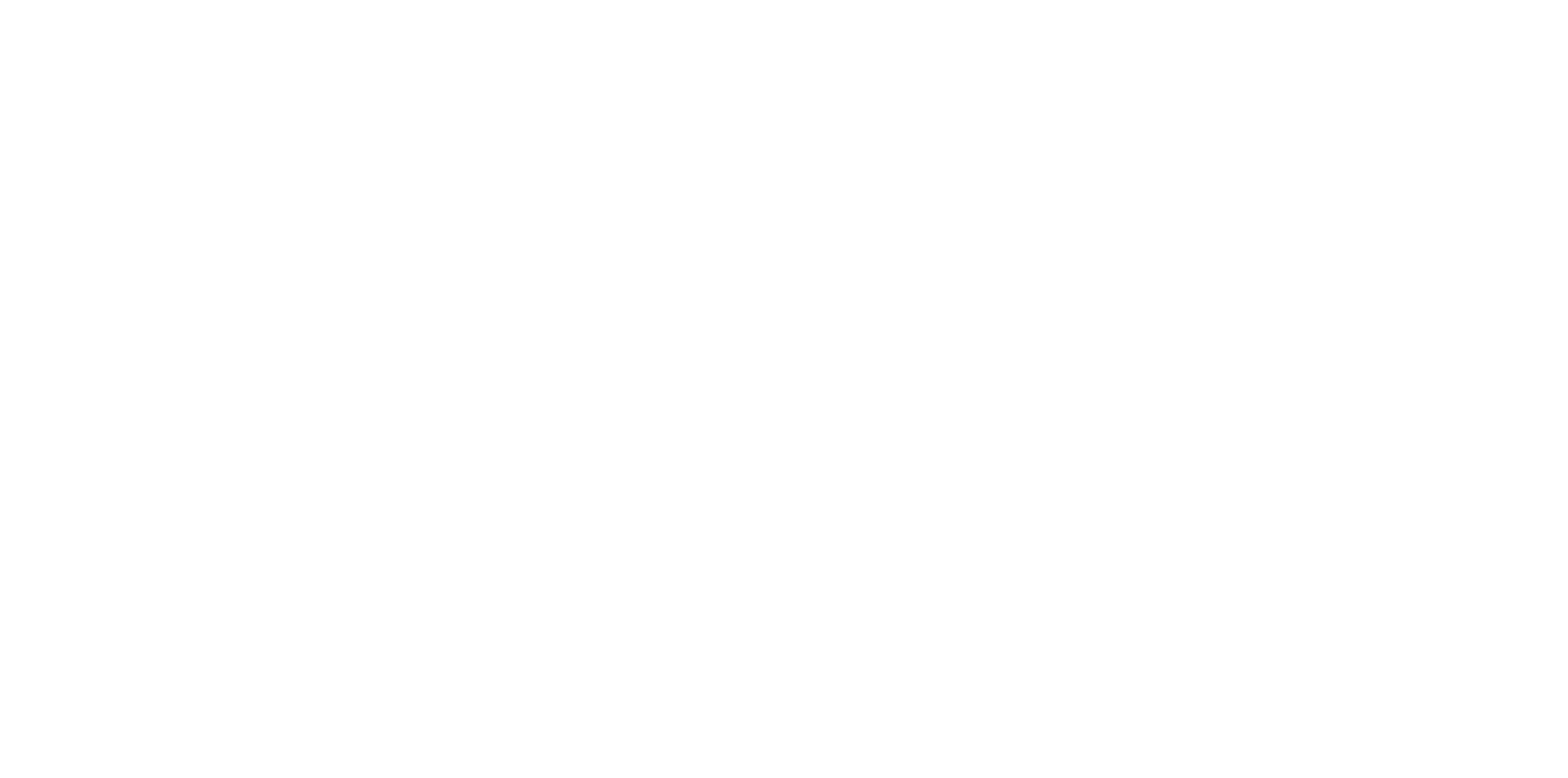
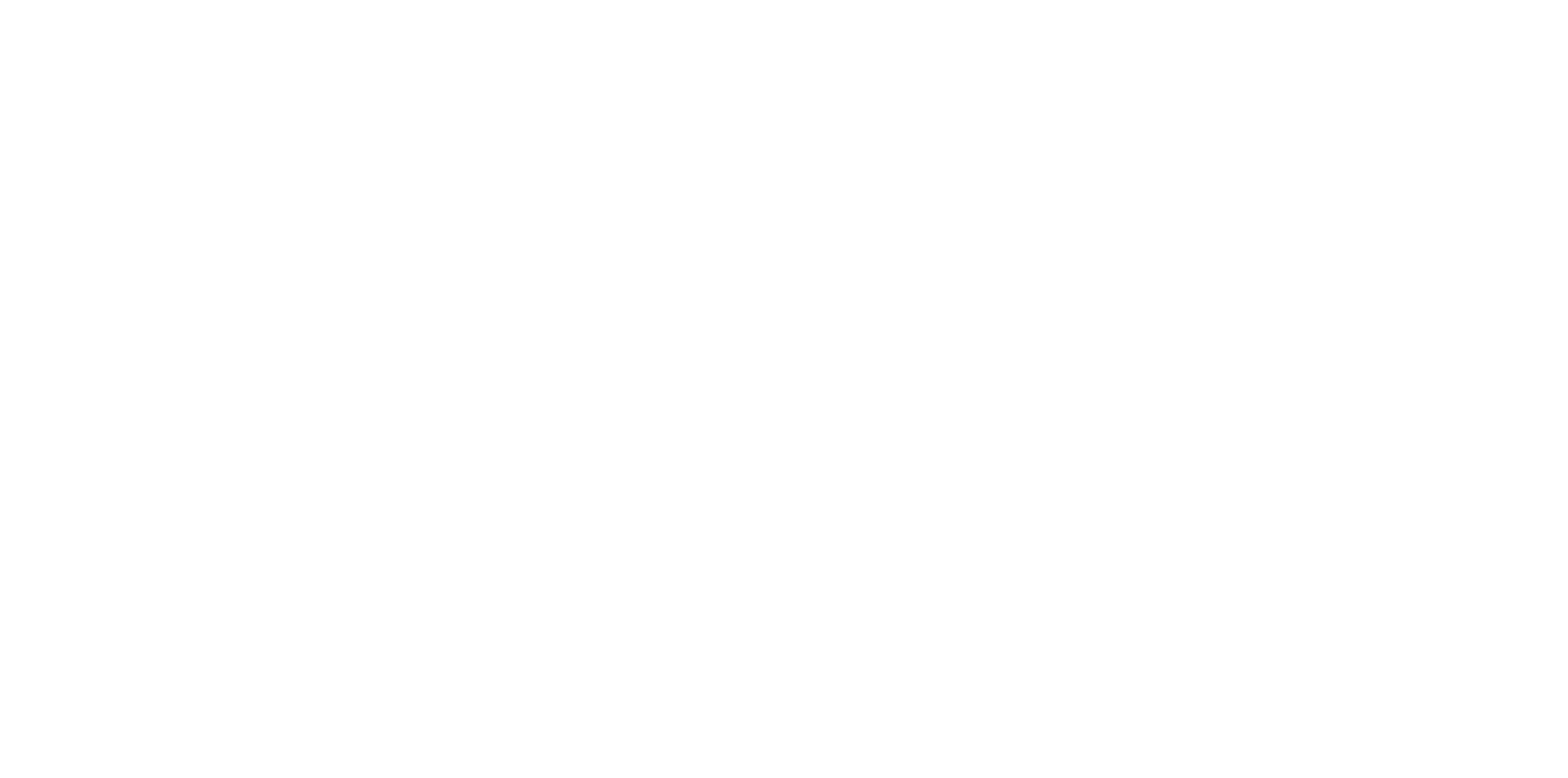
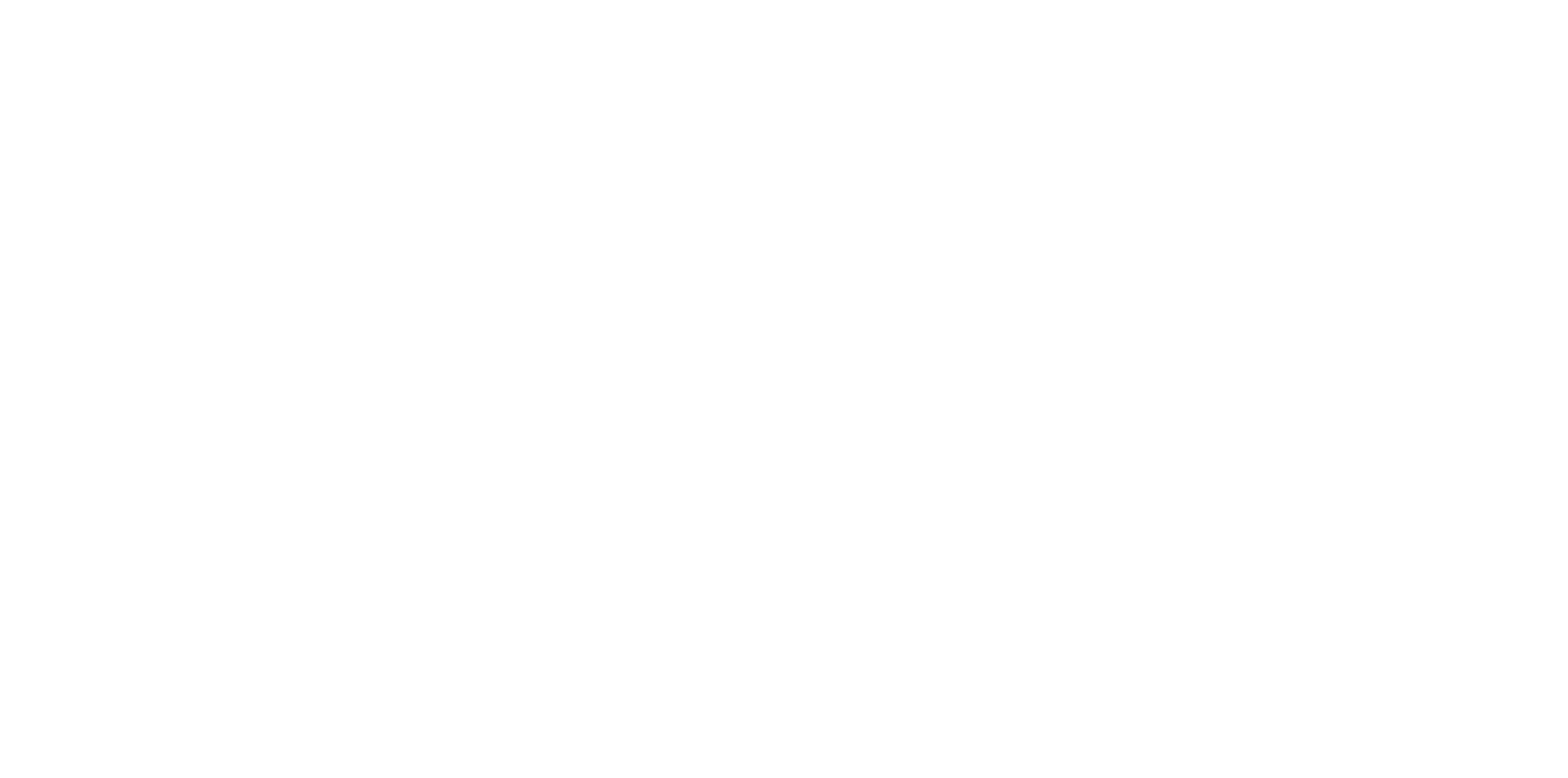
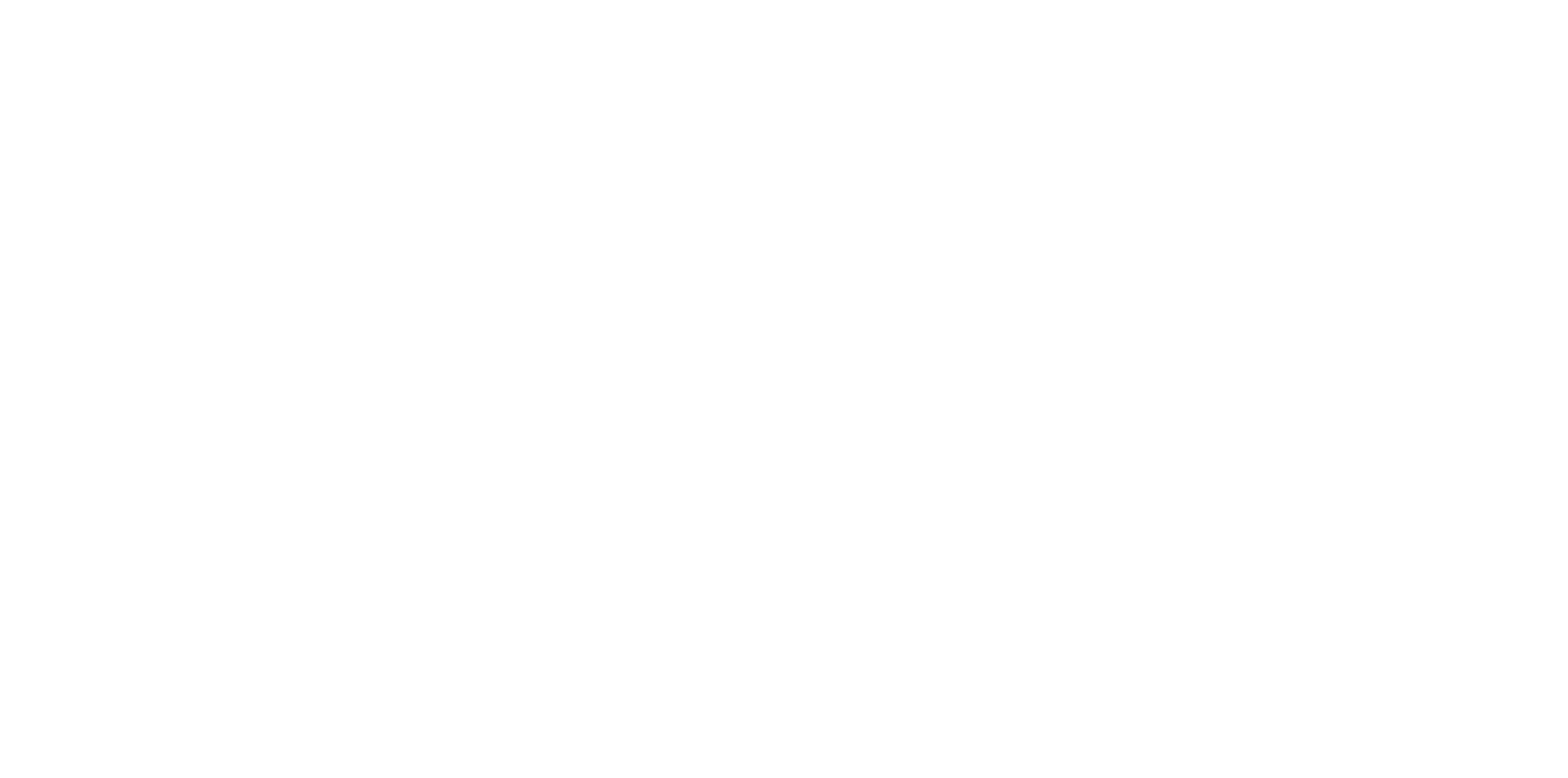
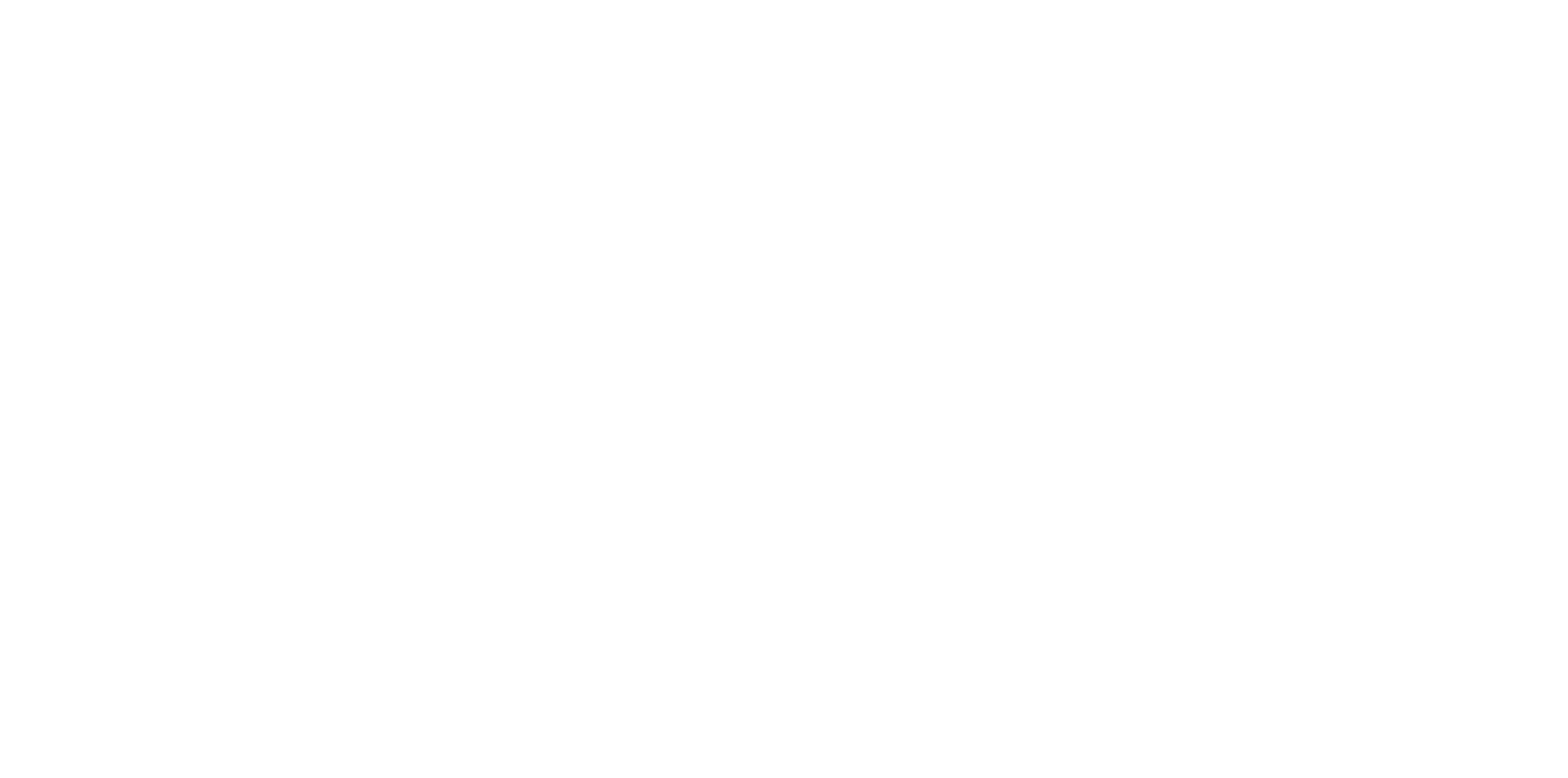
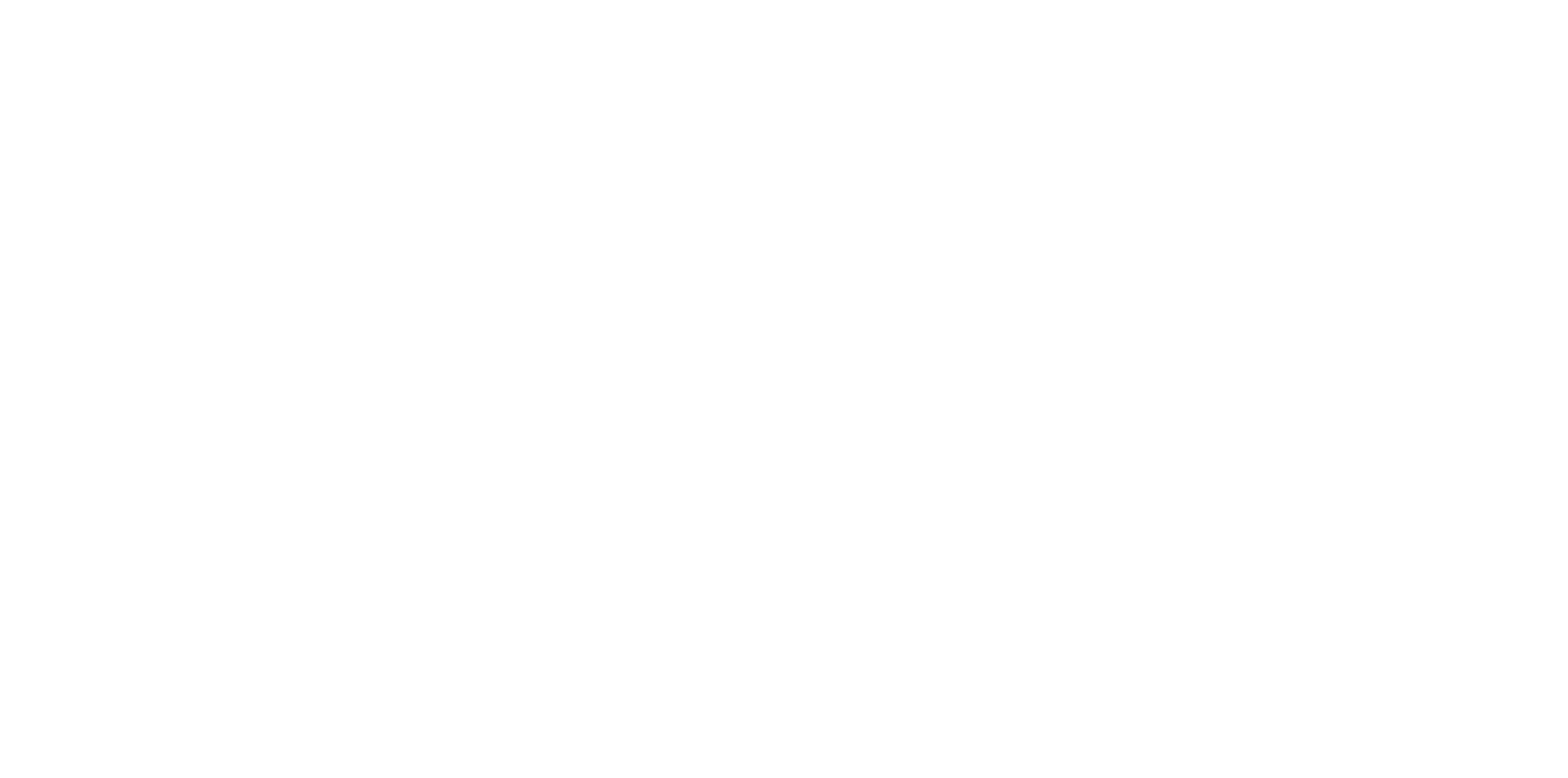
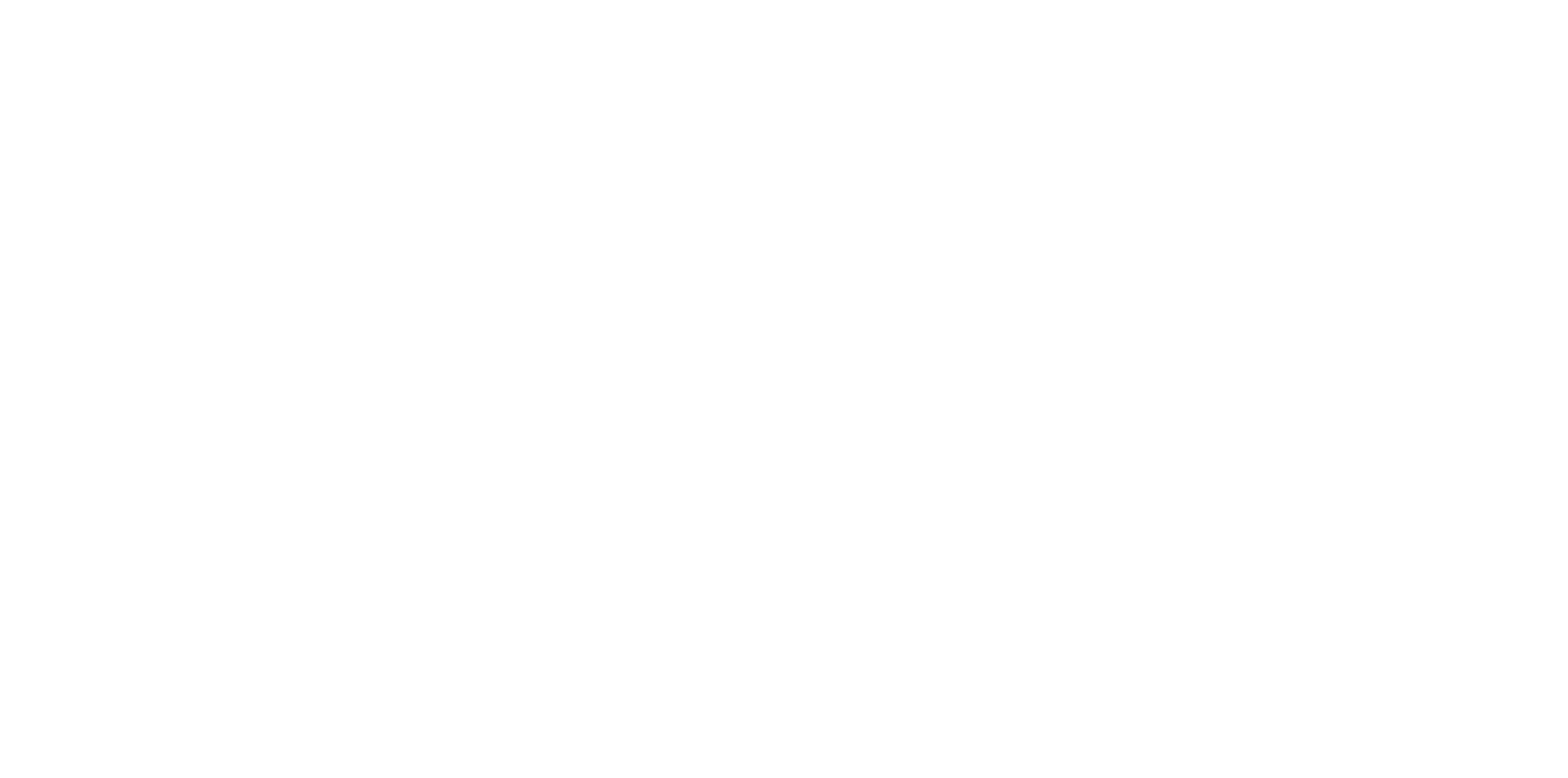
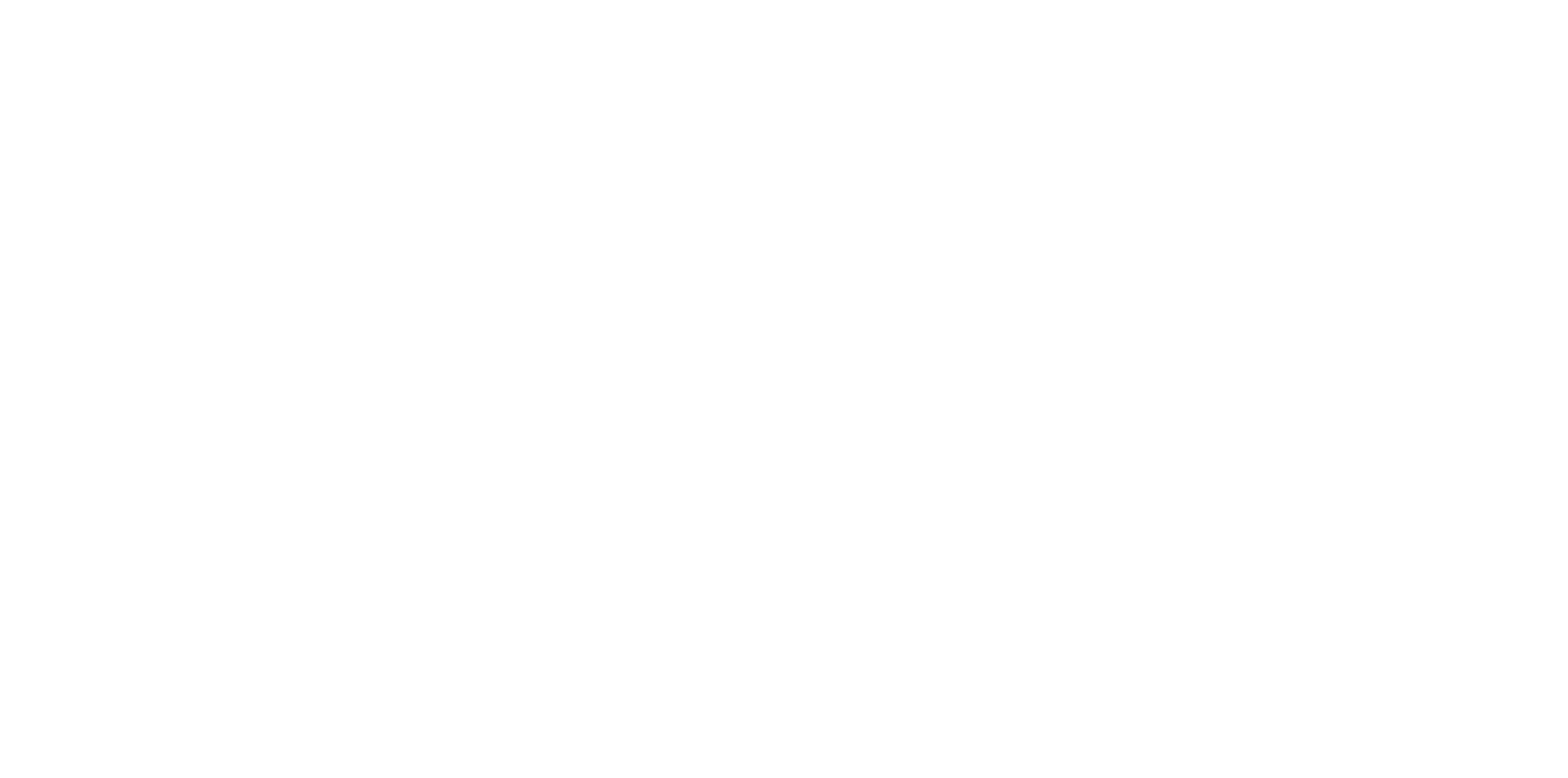








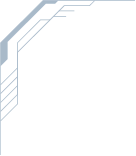
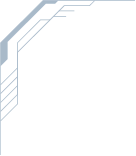
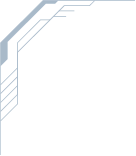
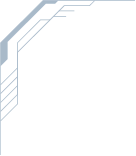
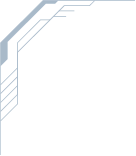
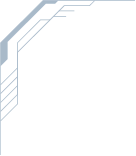
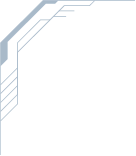
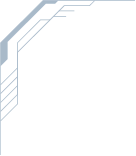
Root Cause Analysis
TRAIN systematically isolates cause-effect relationships for each KPI and contributing variables, uncovering the strongest causal chains that influence the KPIs. This helps identify the root causes of undesirable outcomes, such as low yield or a pipe line leakage. Additionally, TRAIN suggests corrective actions to address these issues.










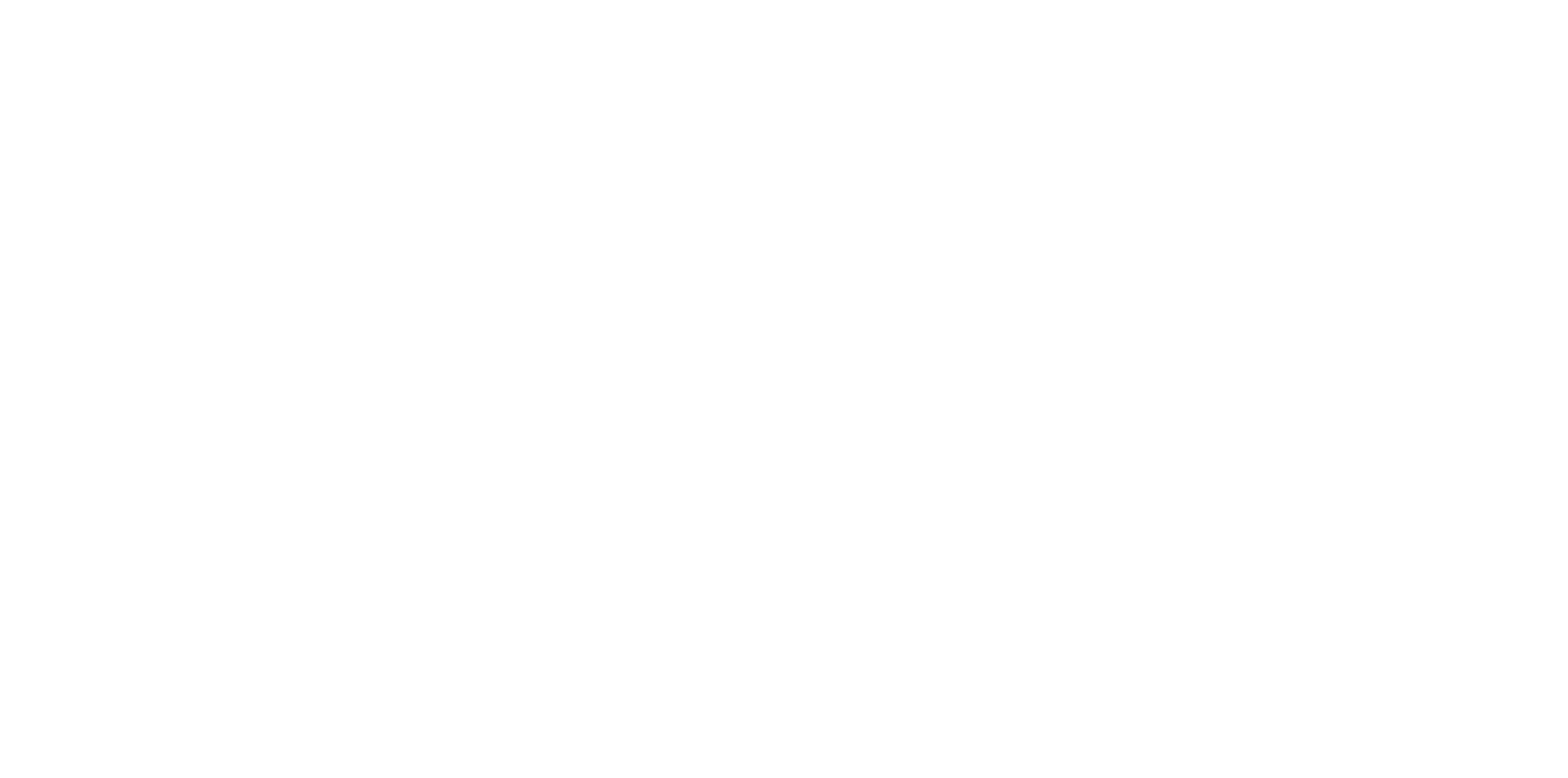
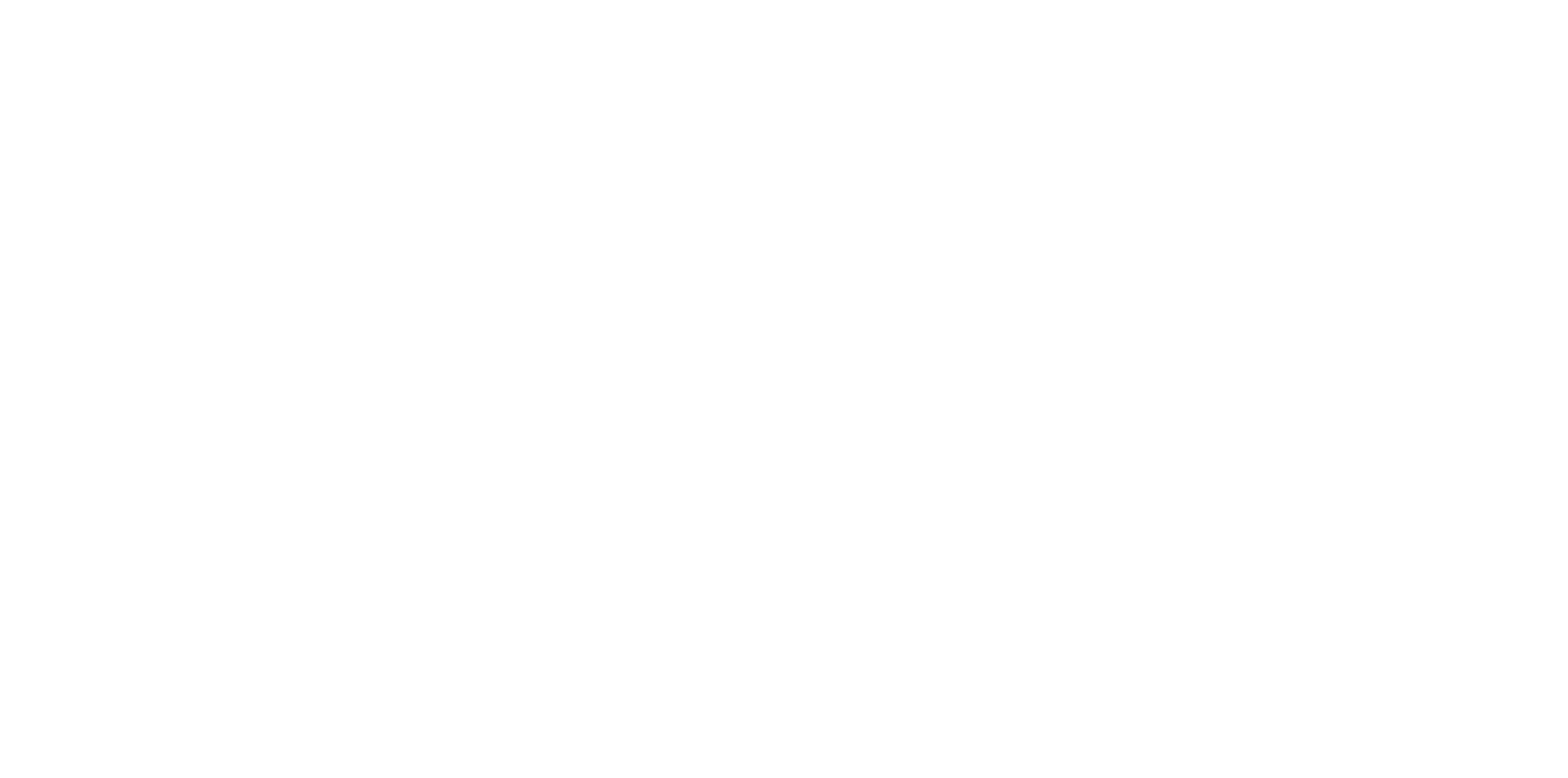
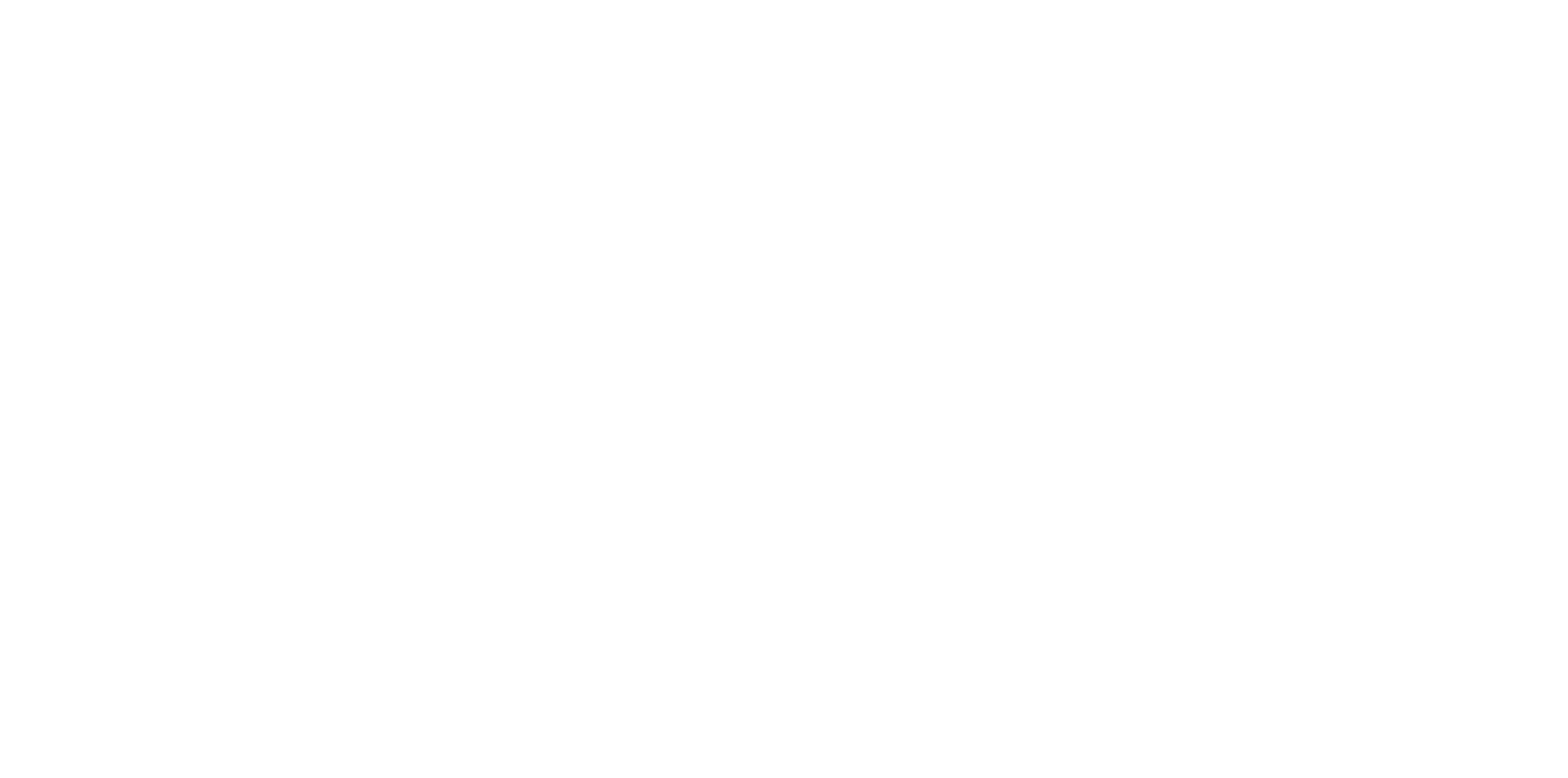
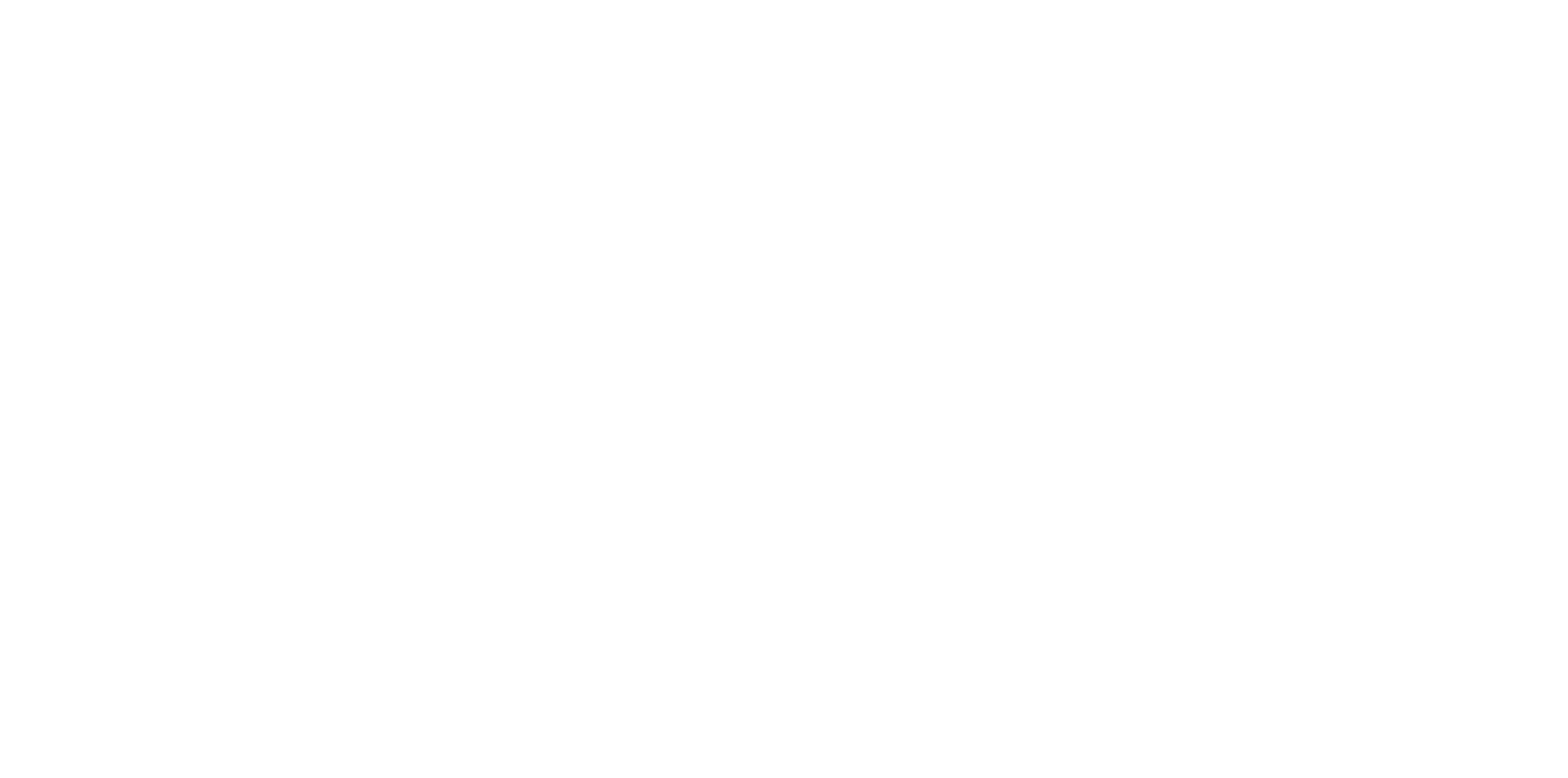
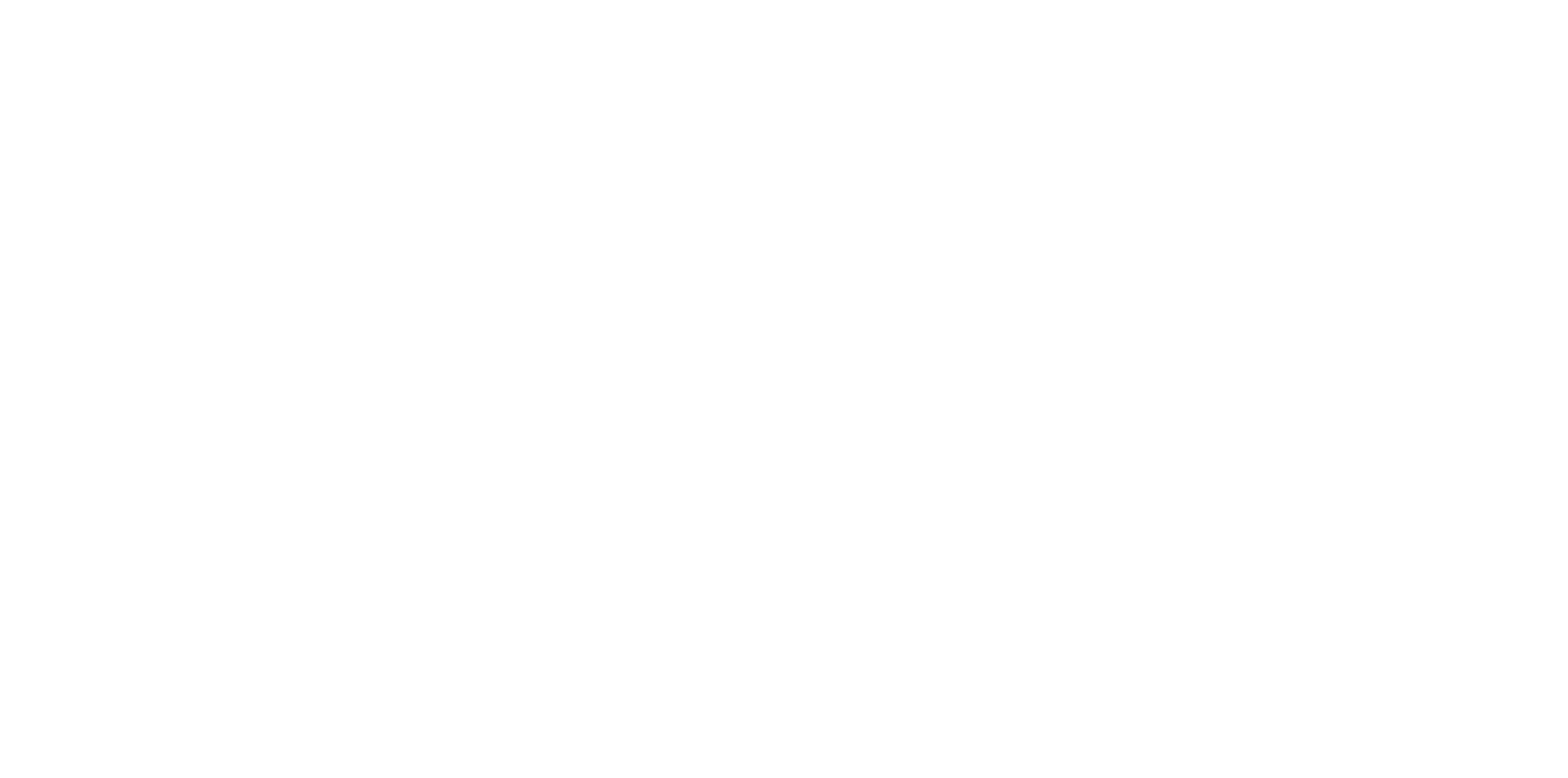
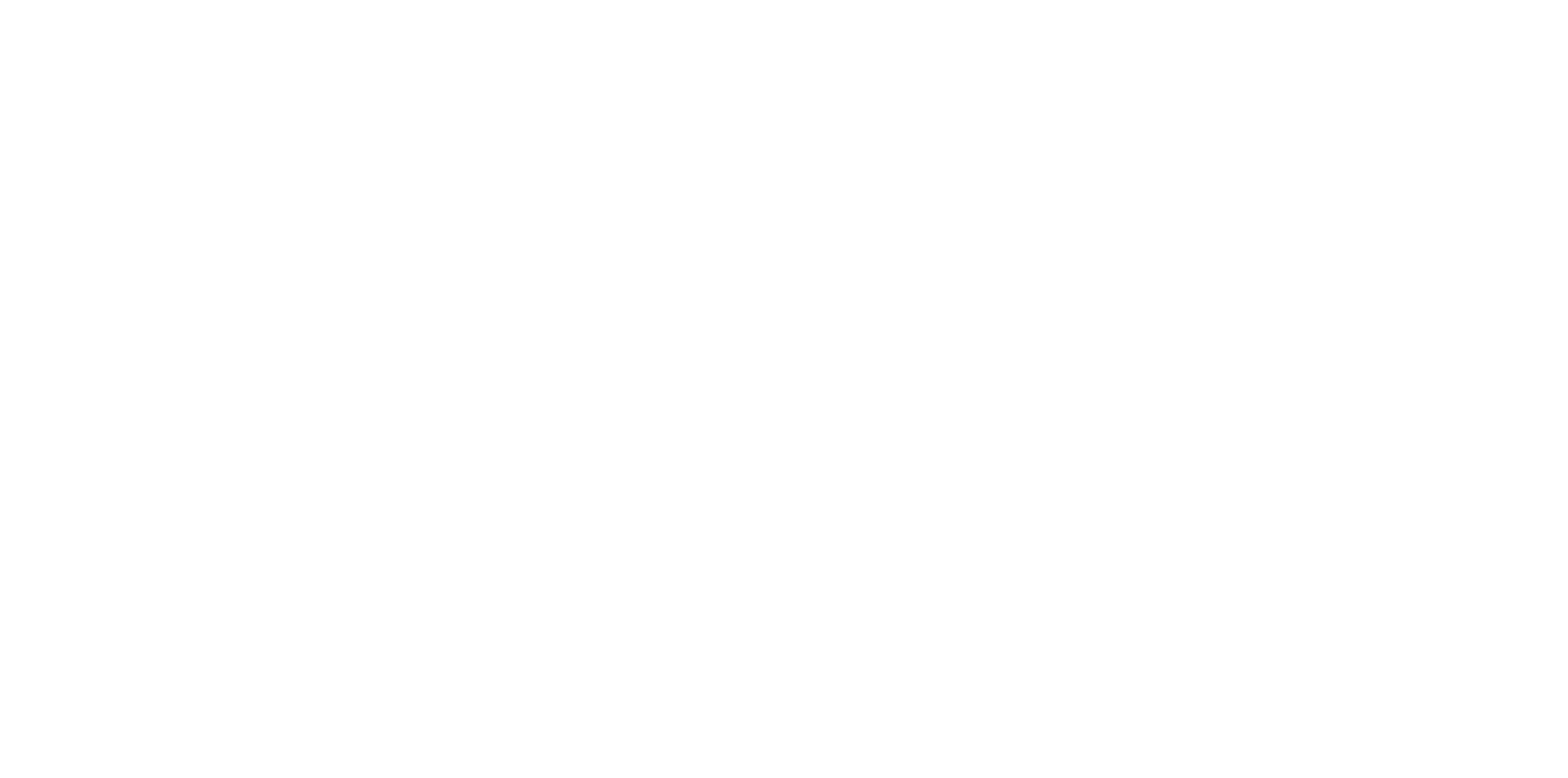
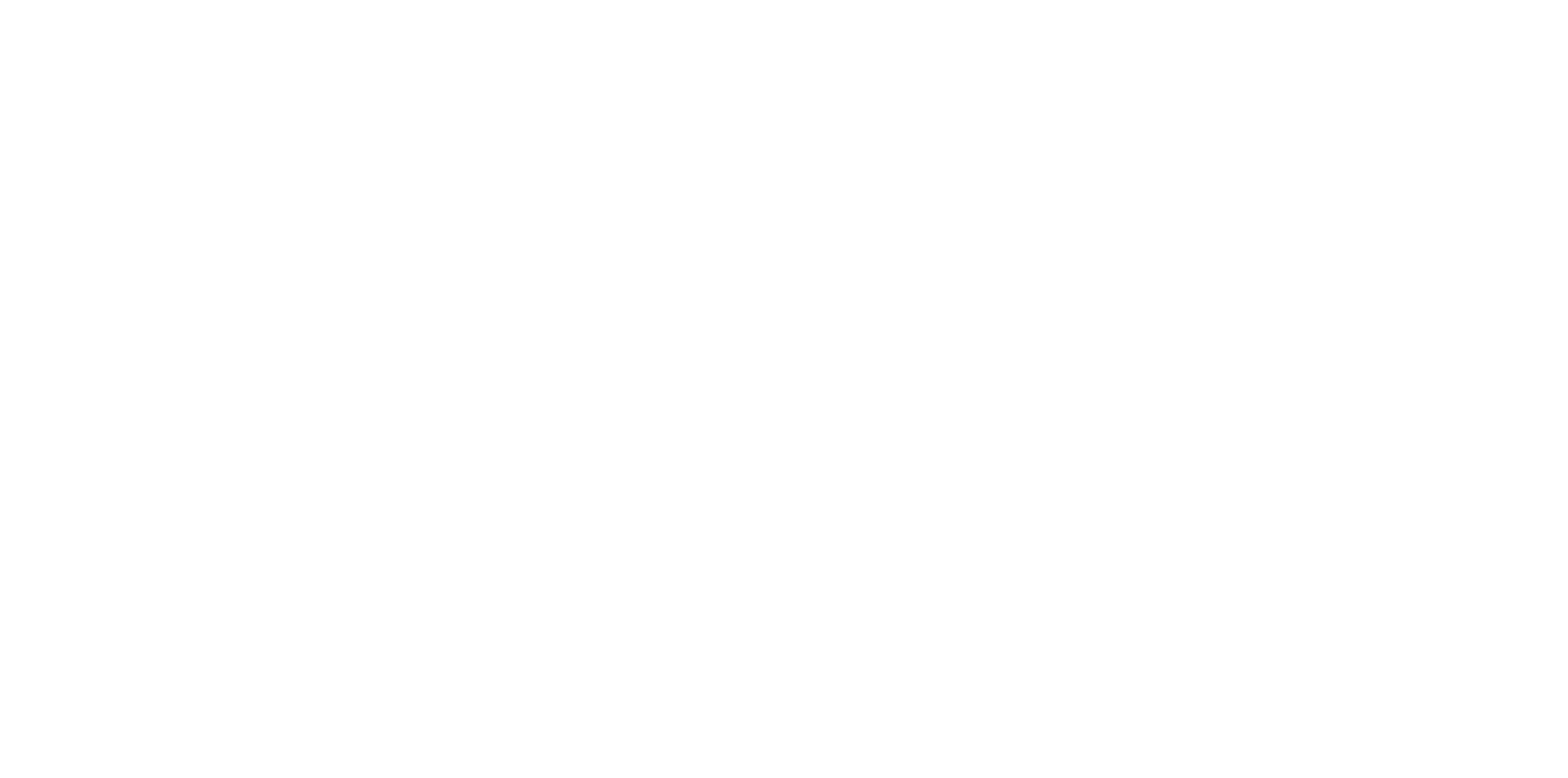
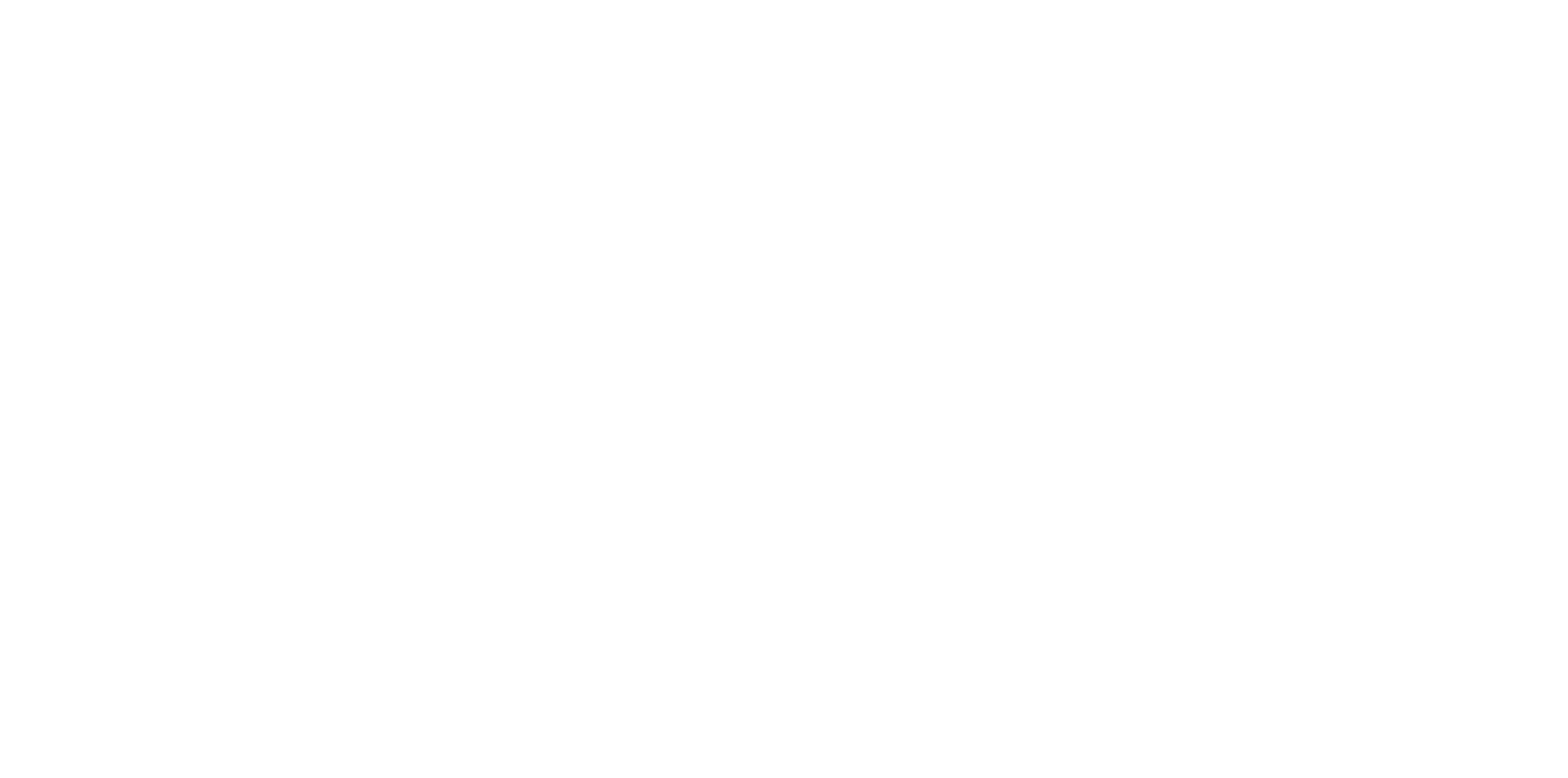
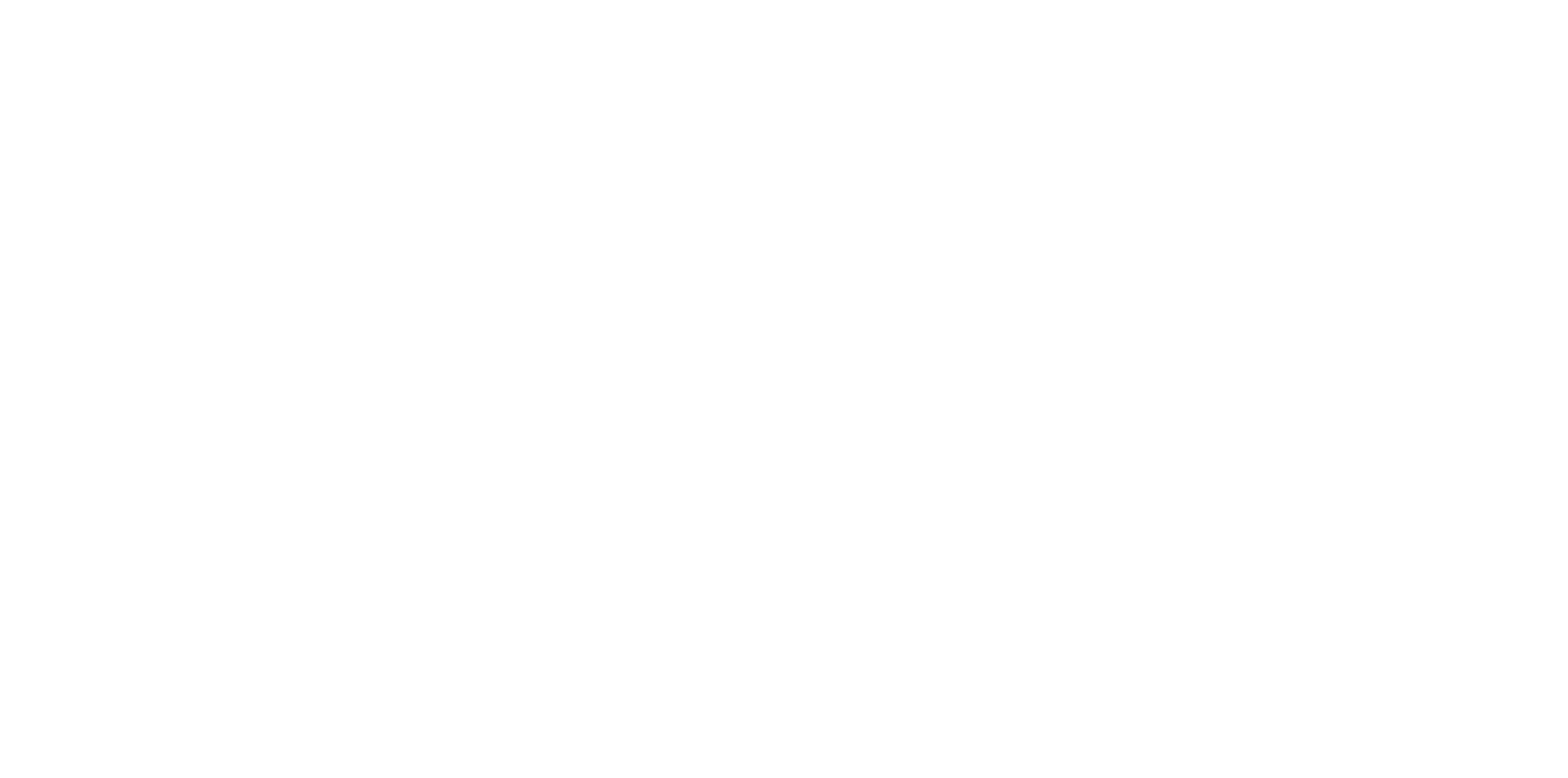
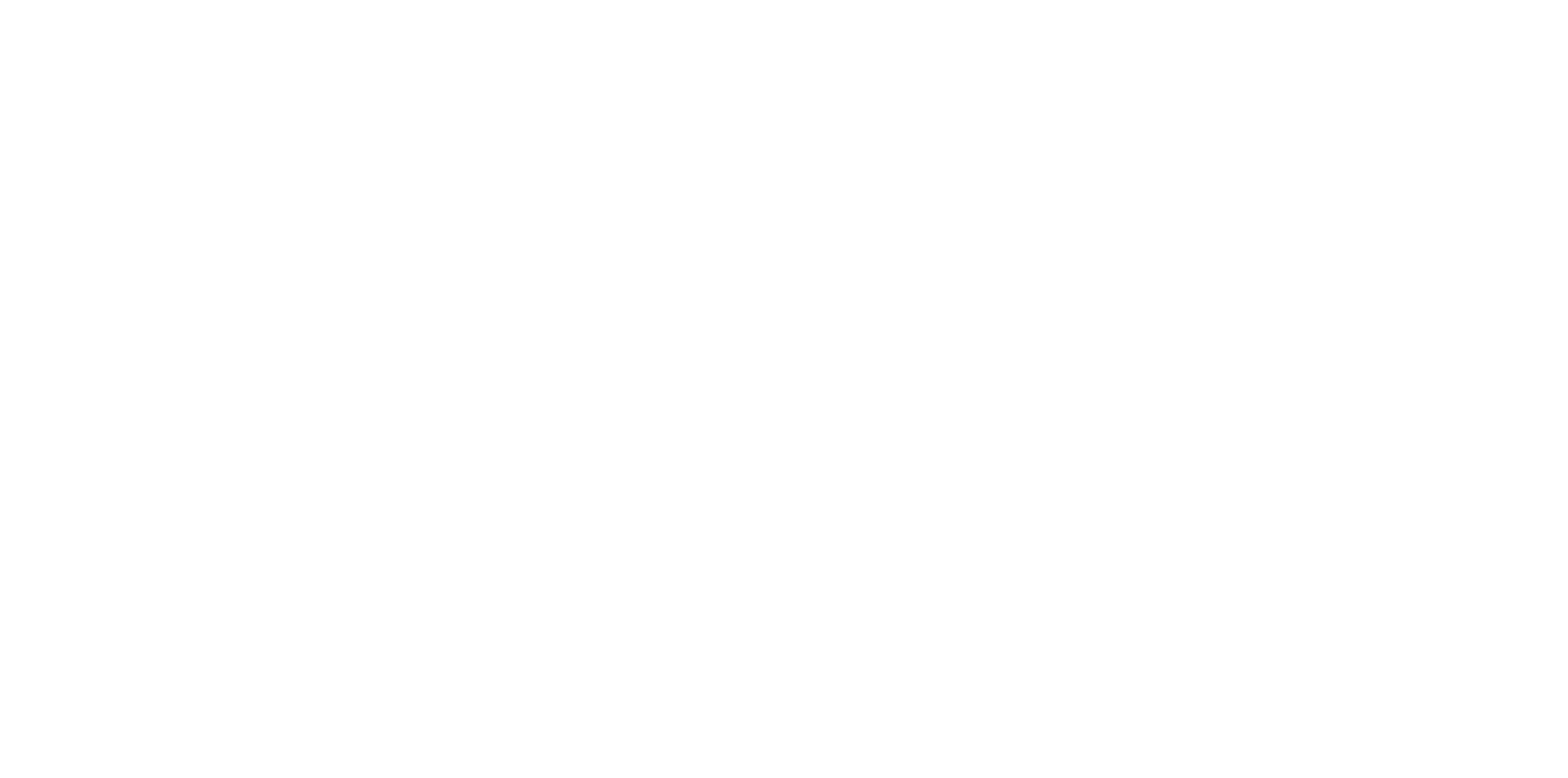








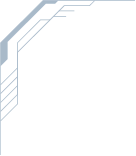
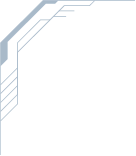
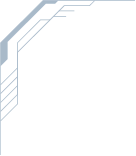
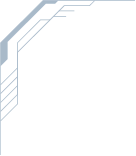
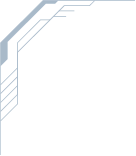
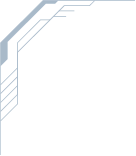
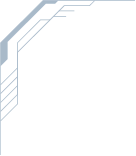
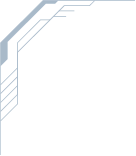
Anomaly Detection
TRAIN automatically detects anomalies by analyzing causal relationship trends and comparing them to the established reference patterns. This detection process also includes root cause analysis and offers remedial actions.










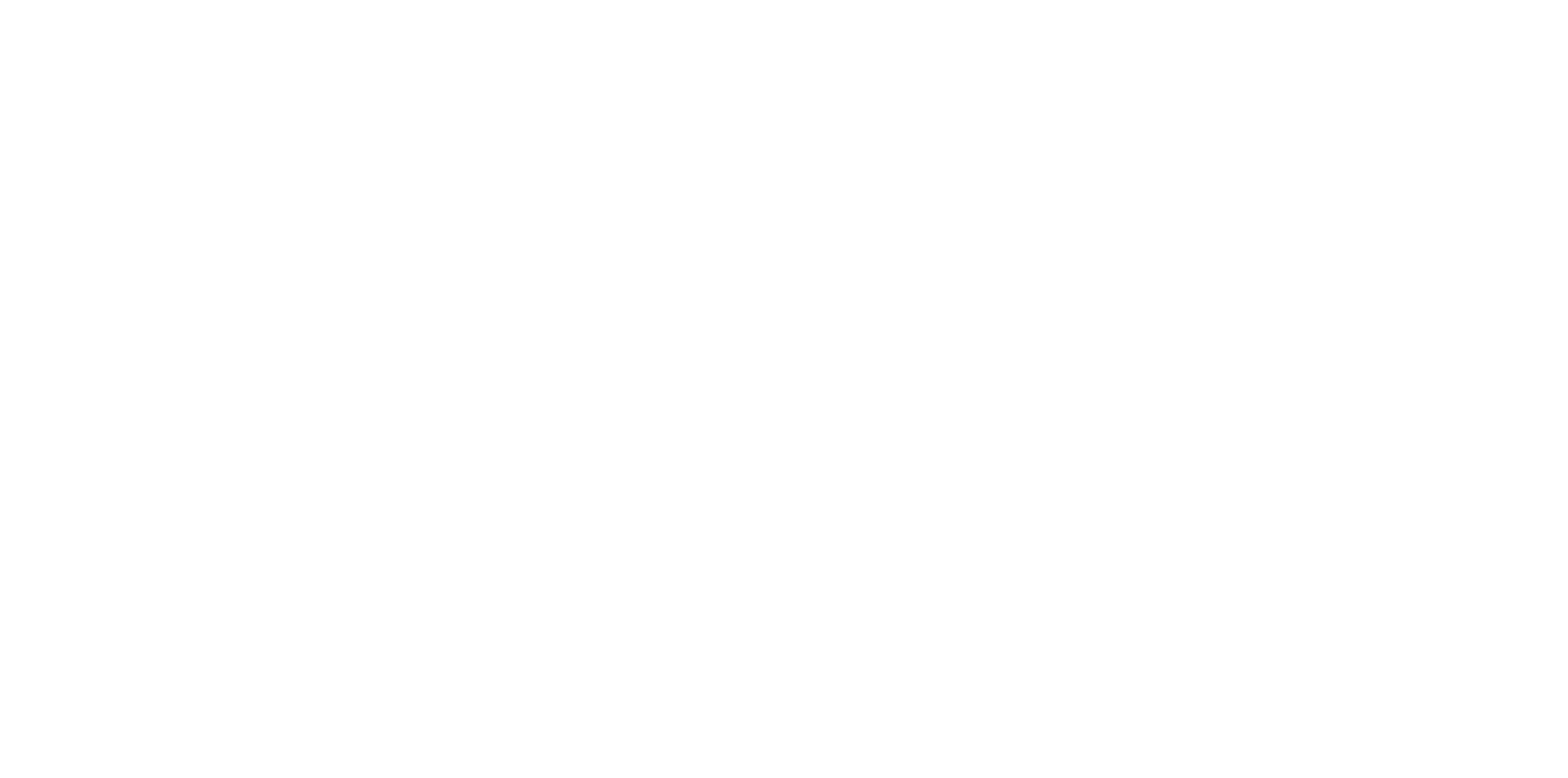
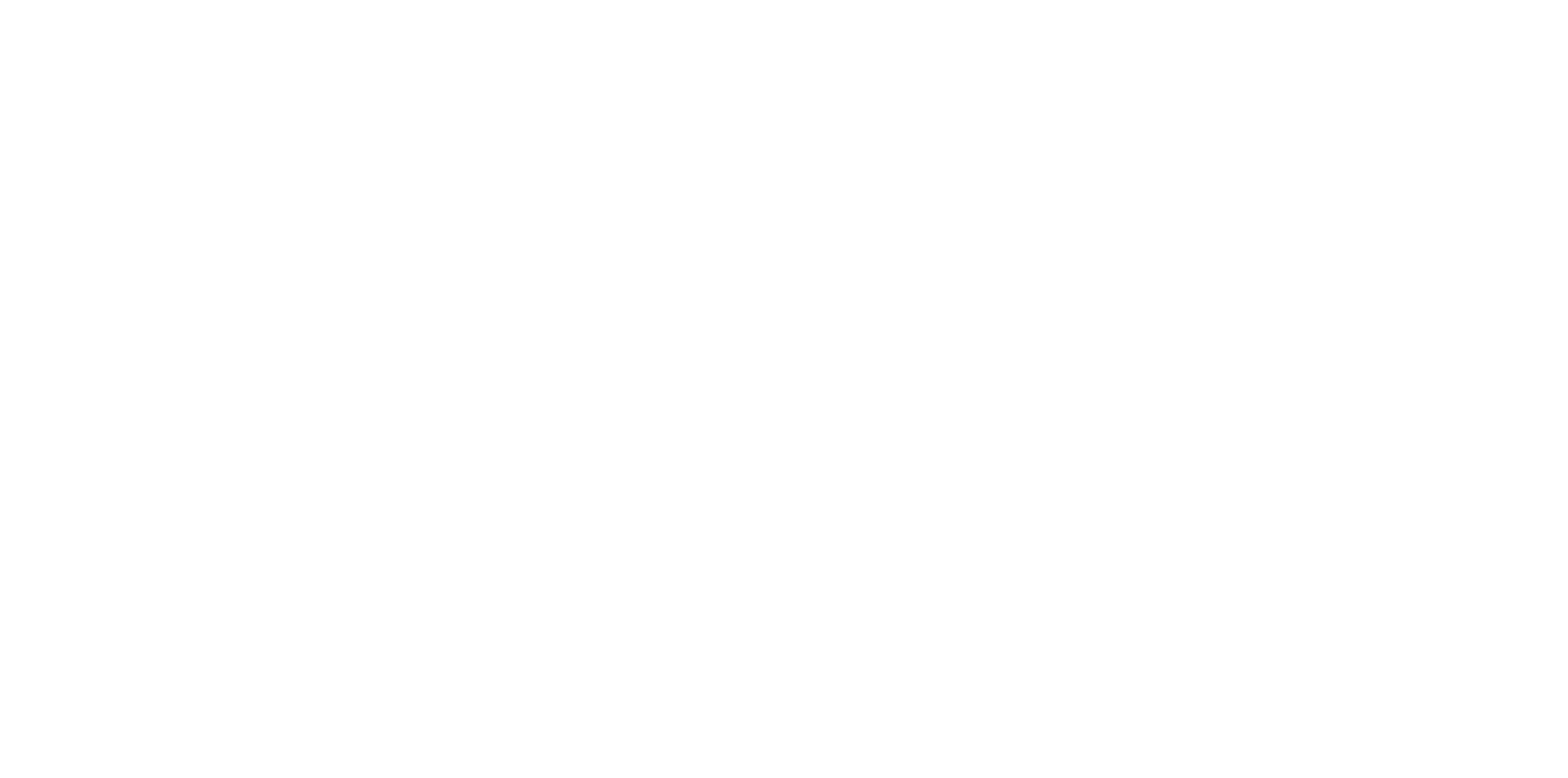
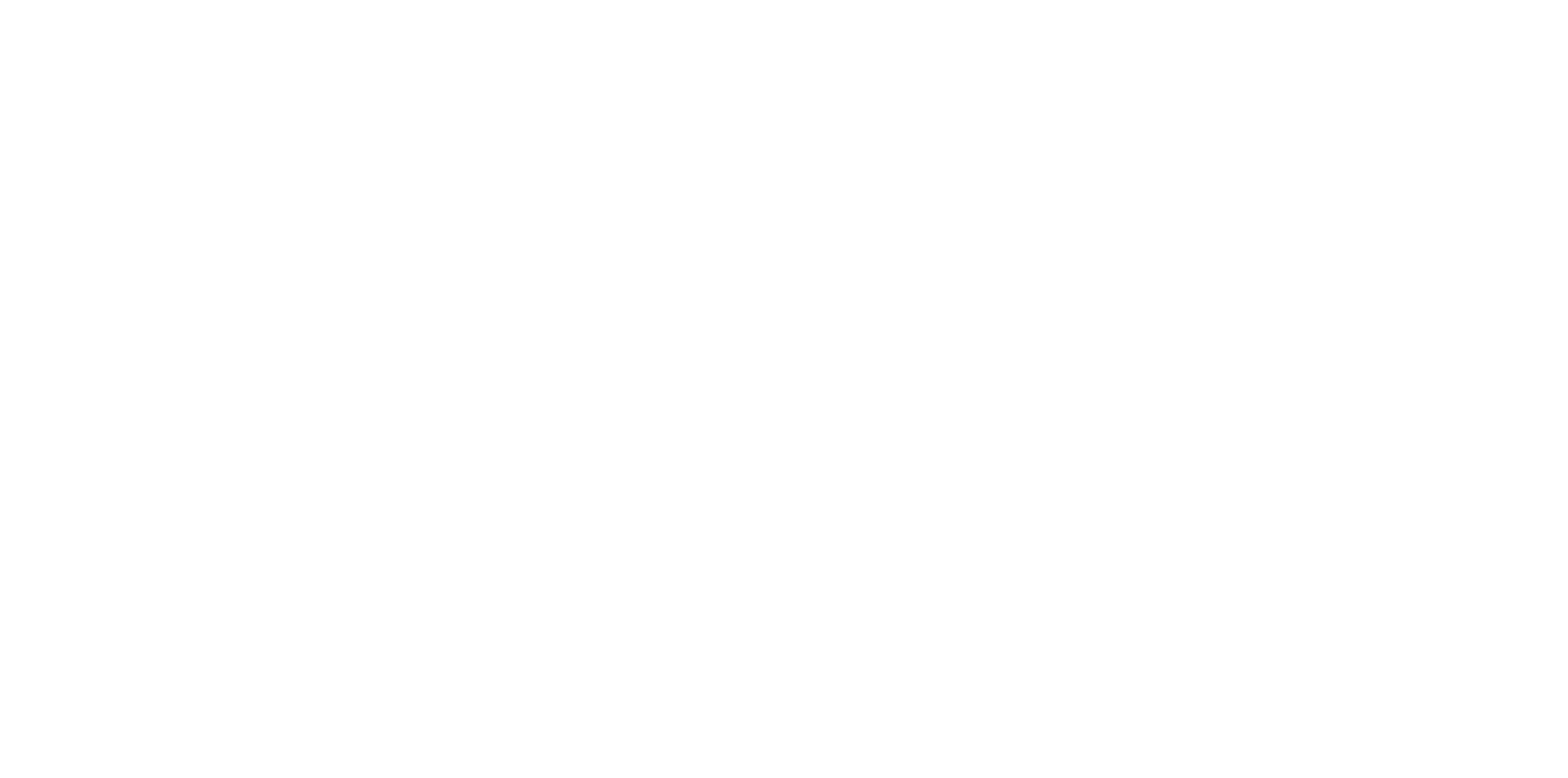
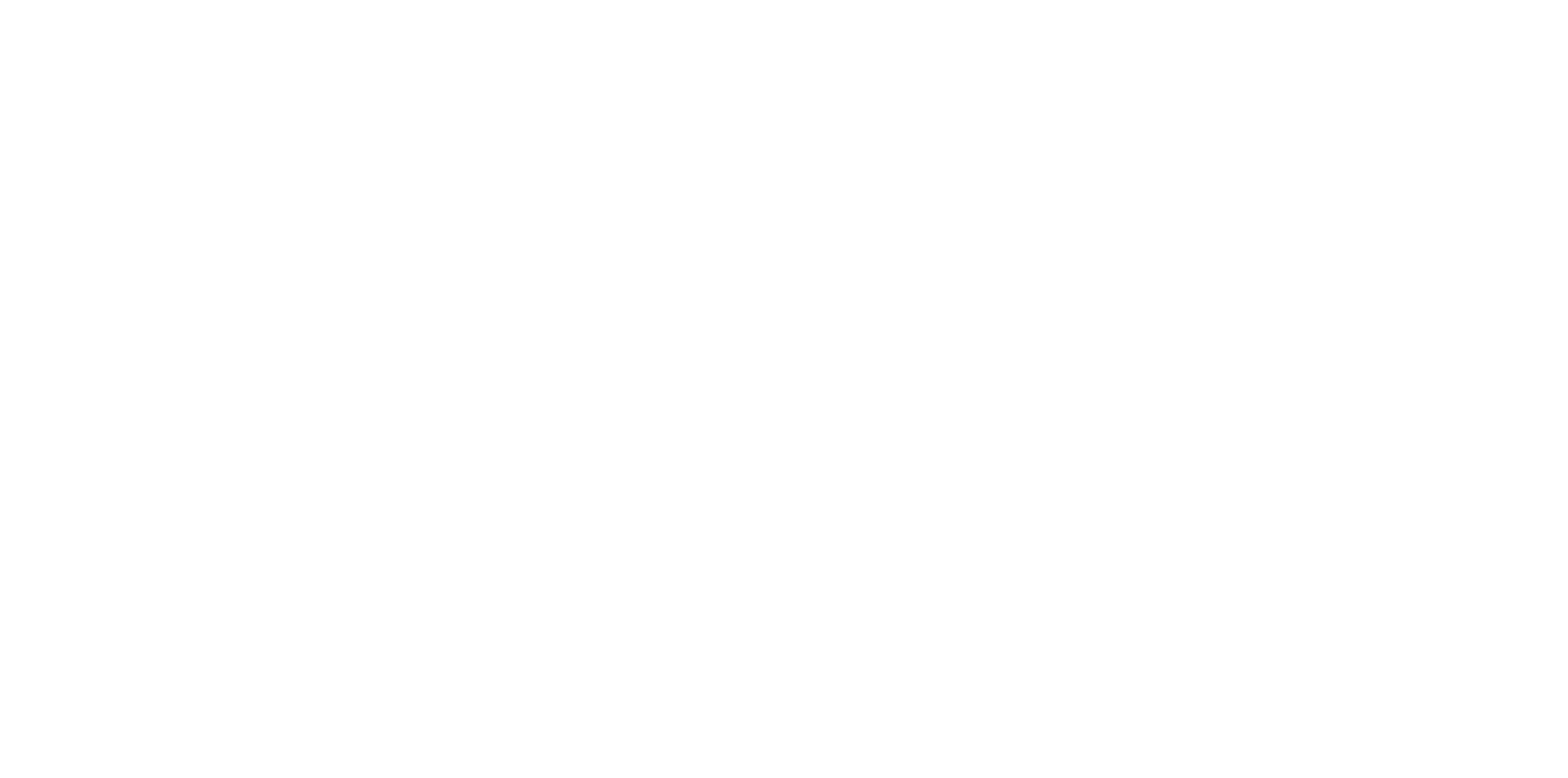
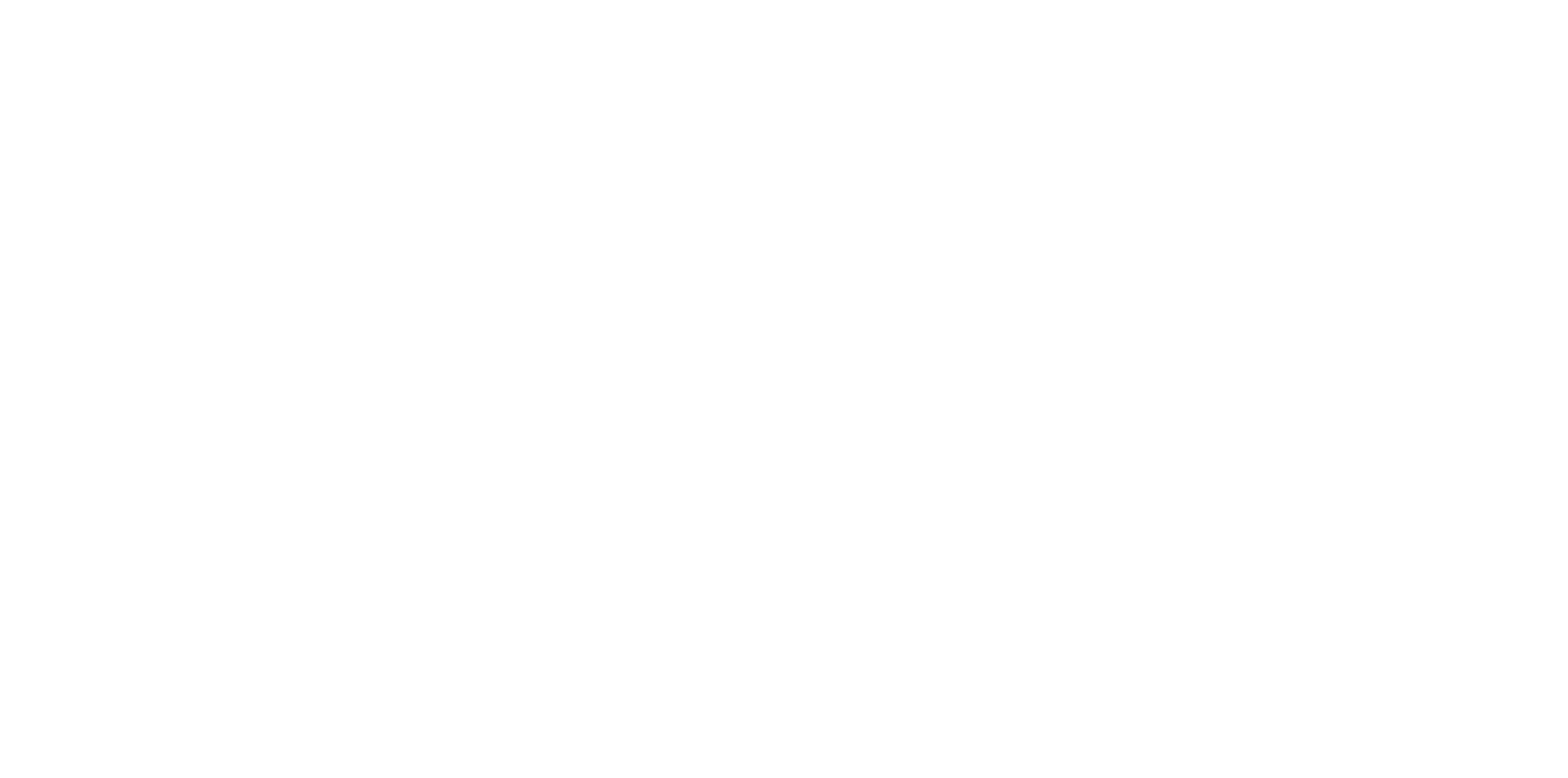
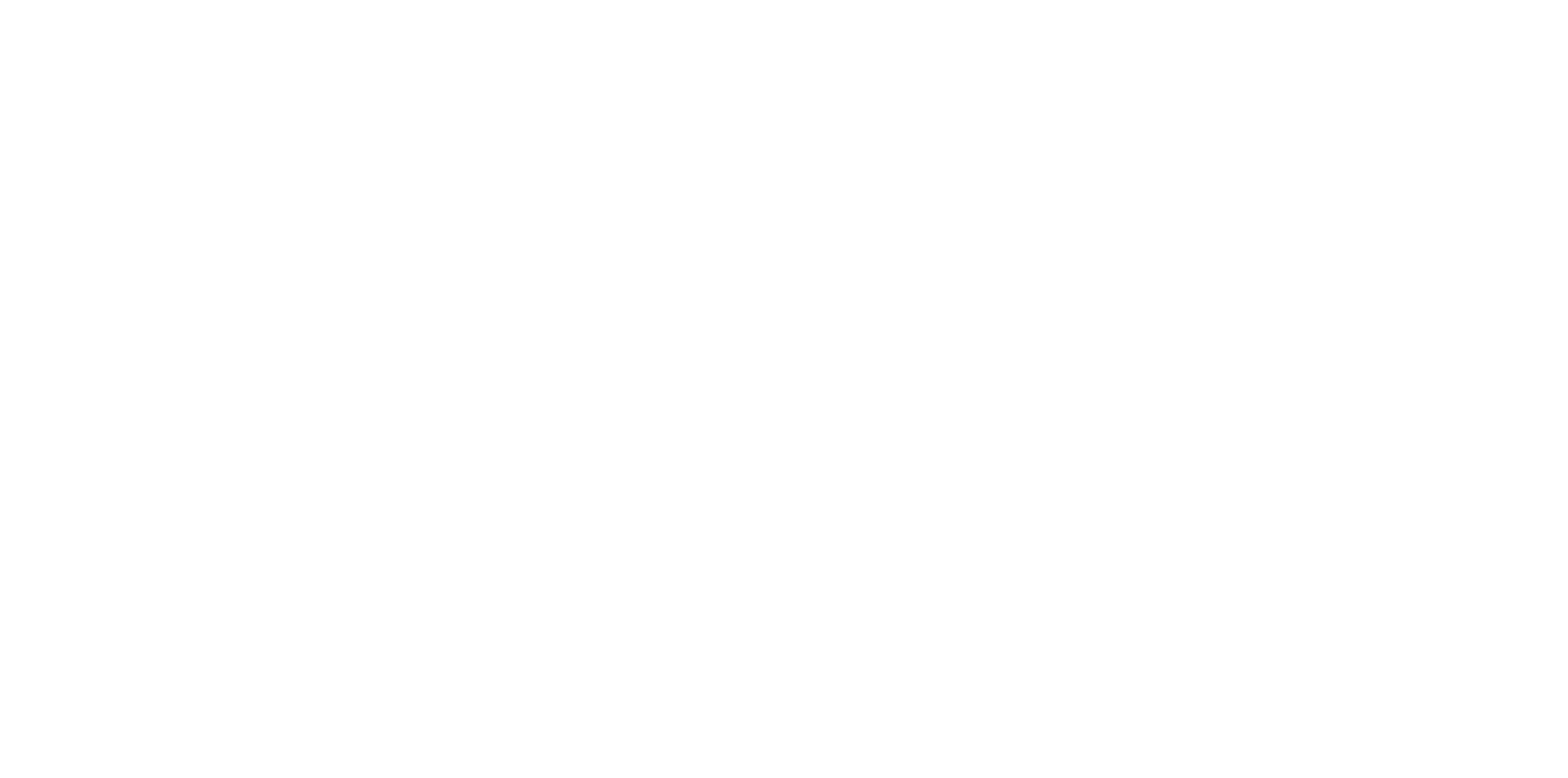
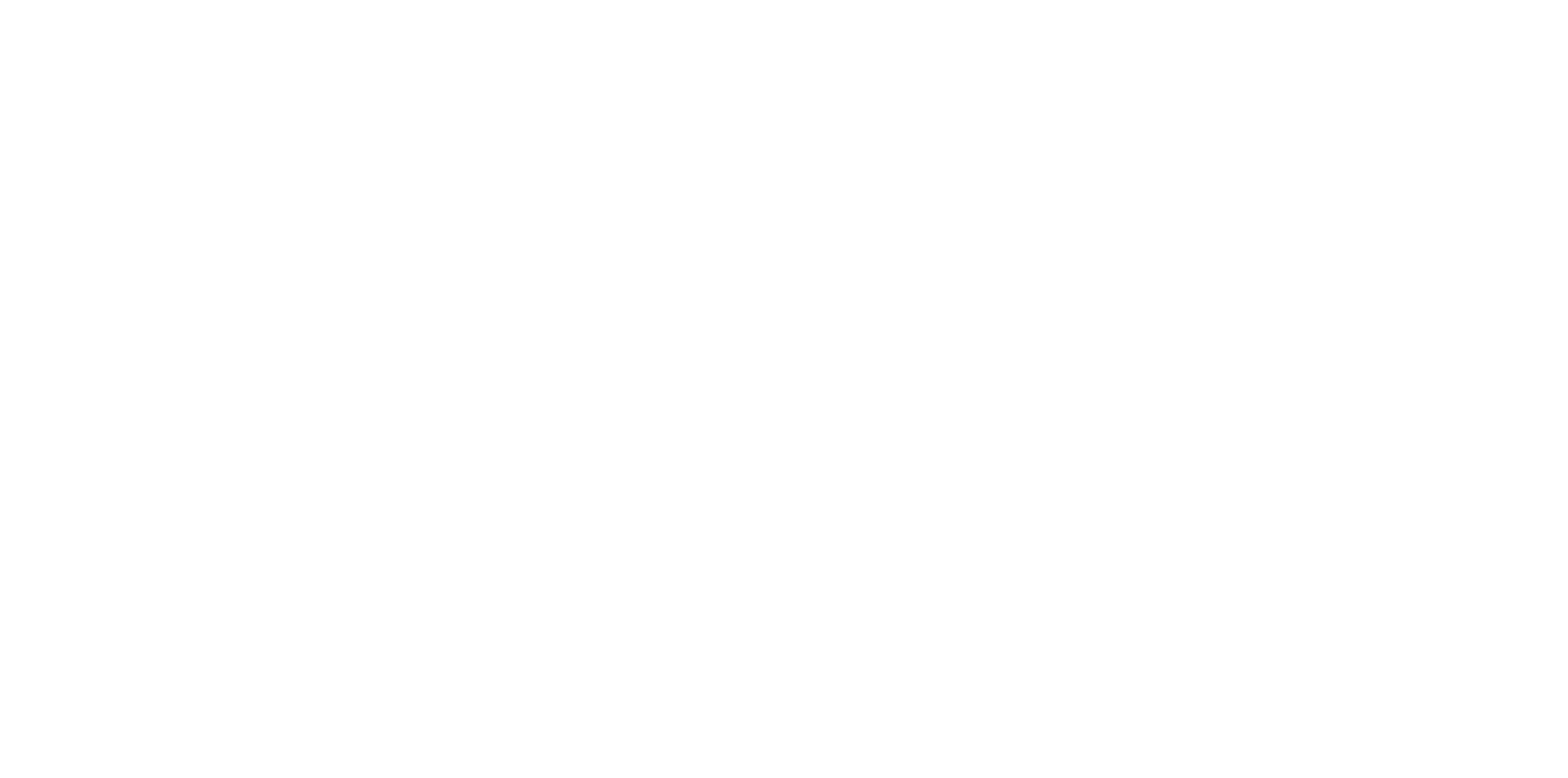
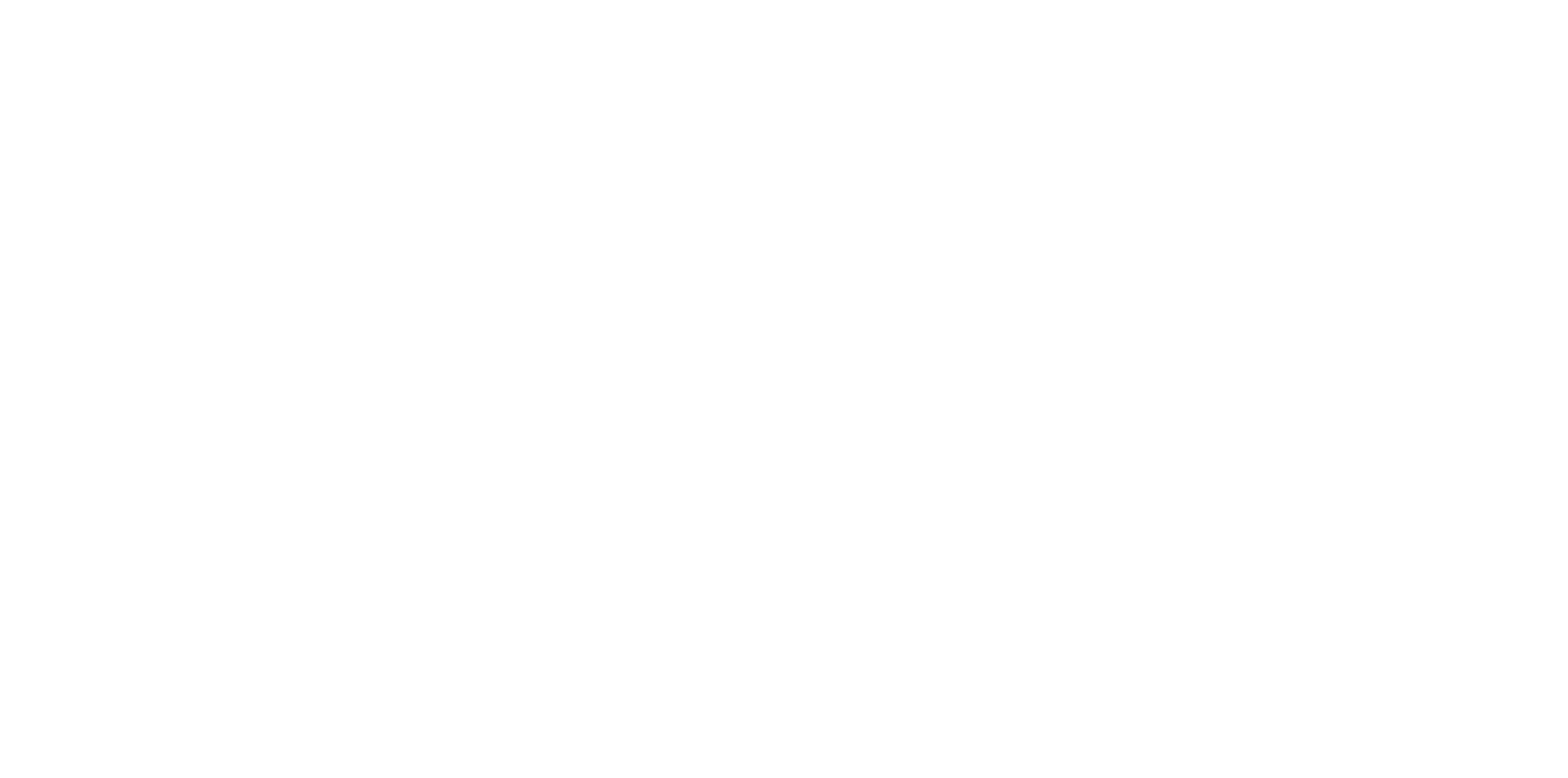
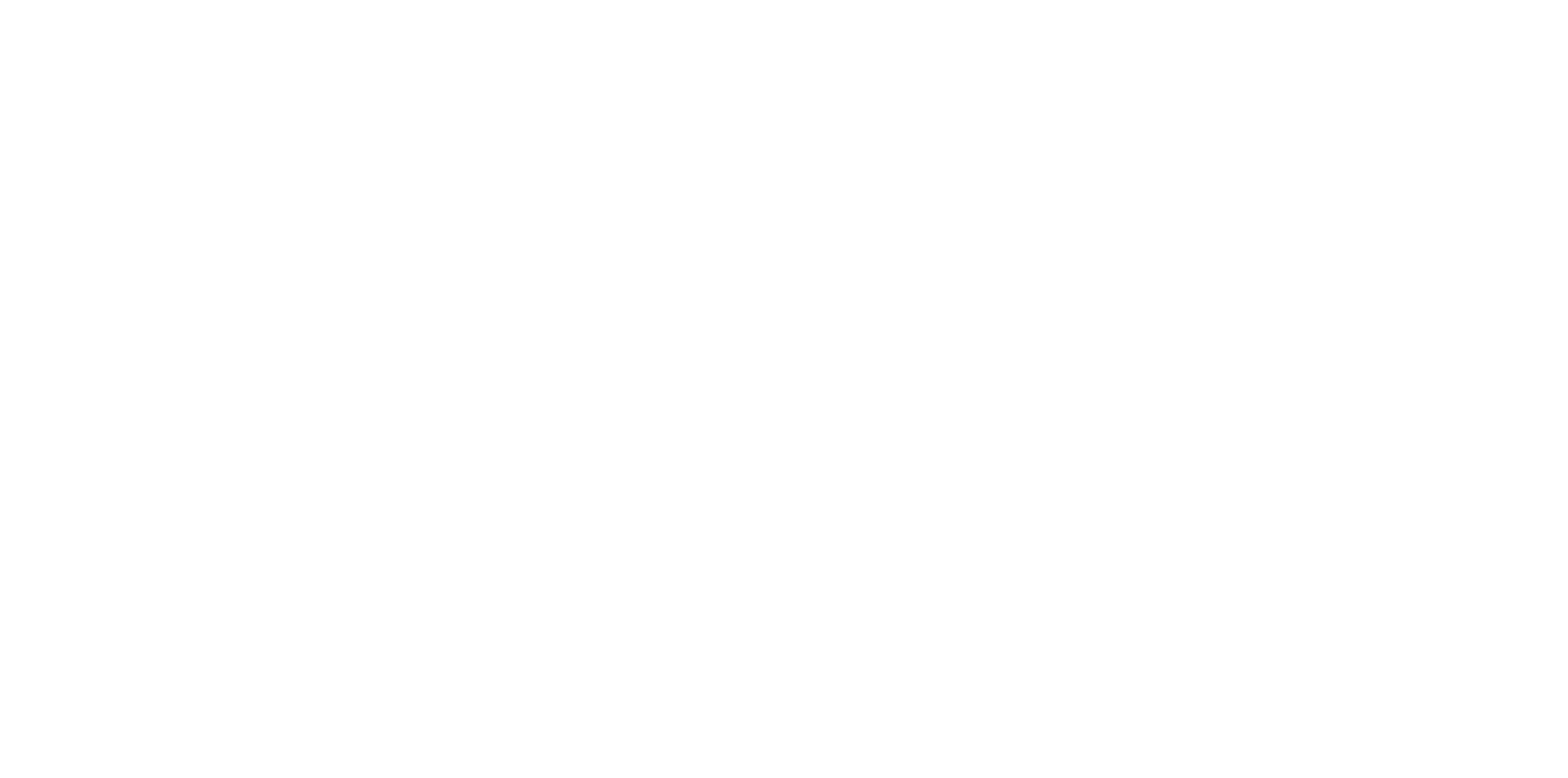
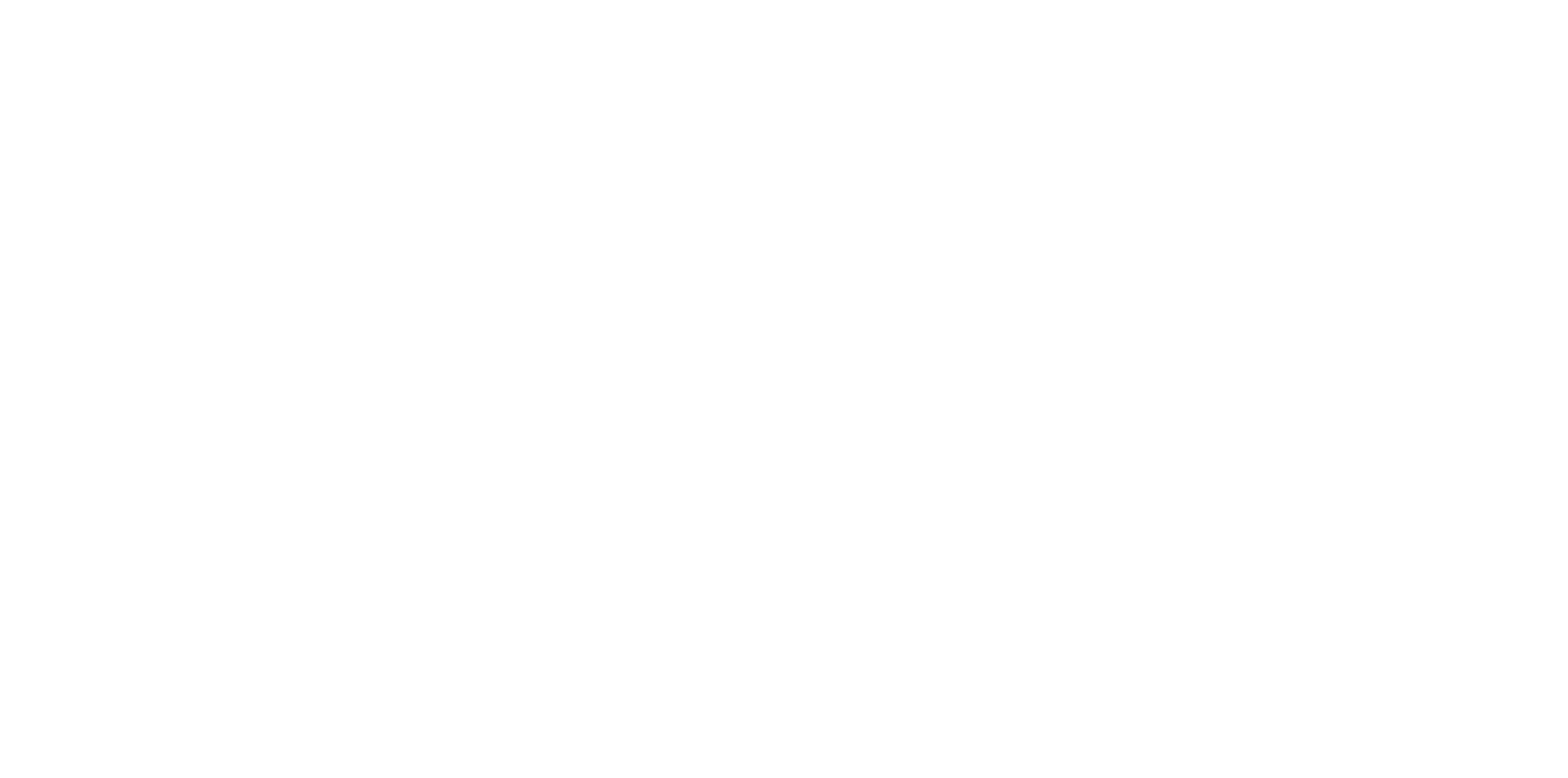








Causal AI Agents
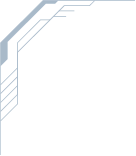
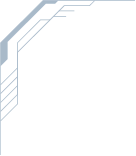
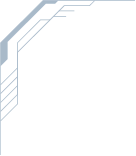
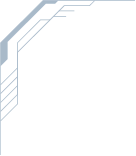
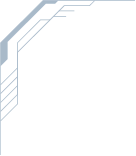
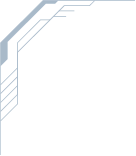
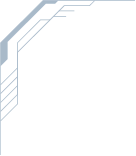
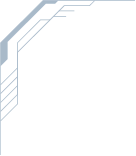
Real solutions for real-world problems
AI agents brings use case specific causal analysis and decision support using the learned causal models and causal analysis across supply chain, process control, energy optimization, worker’s safety and asset reliability domain.








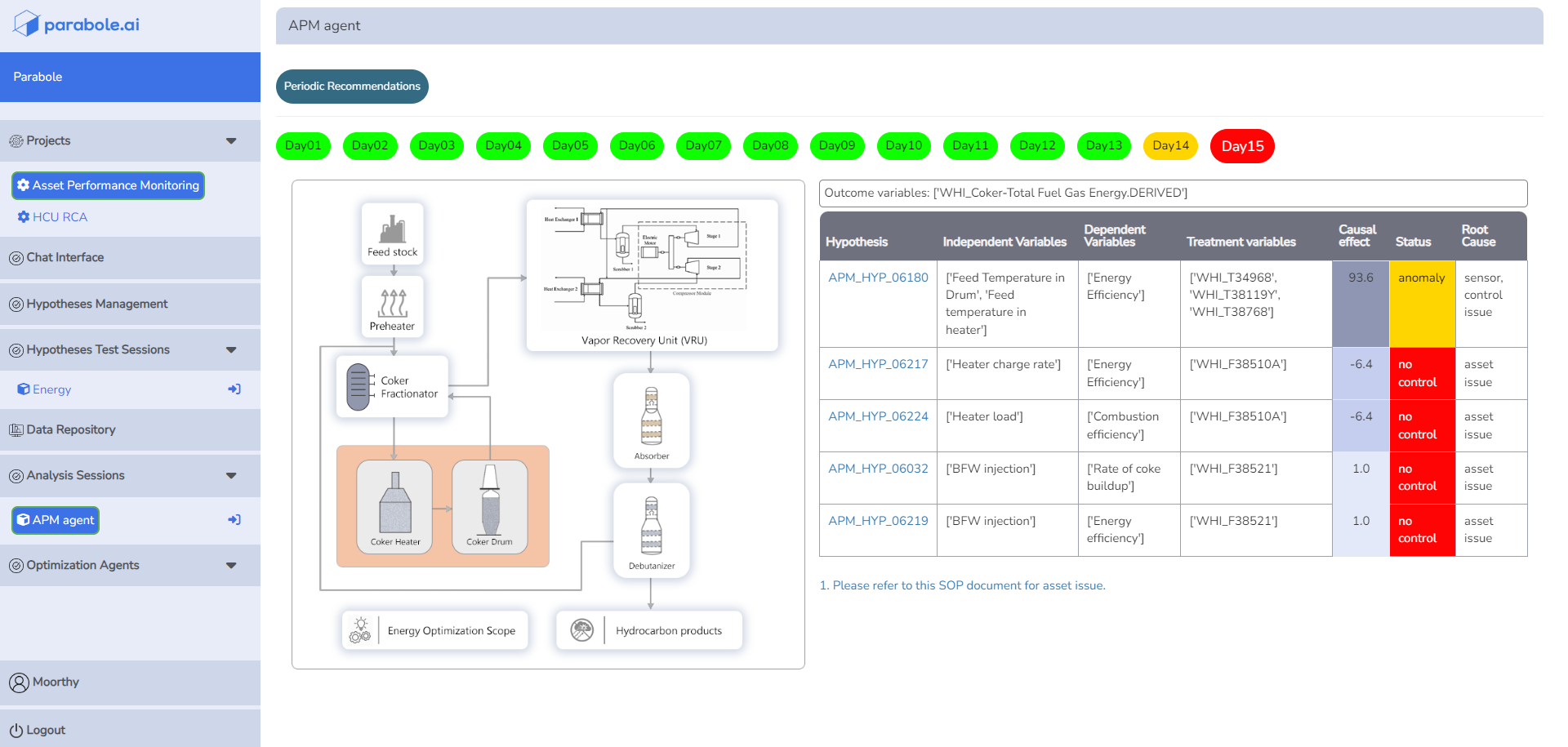
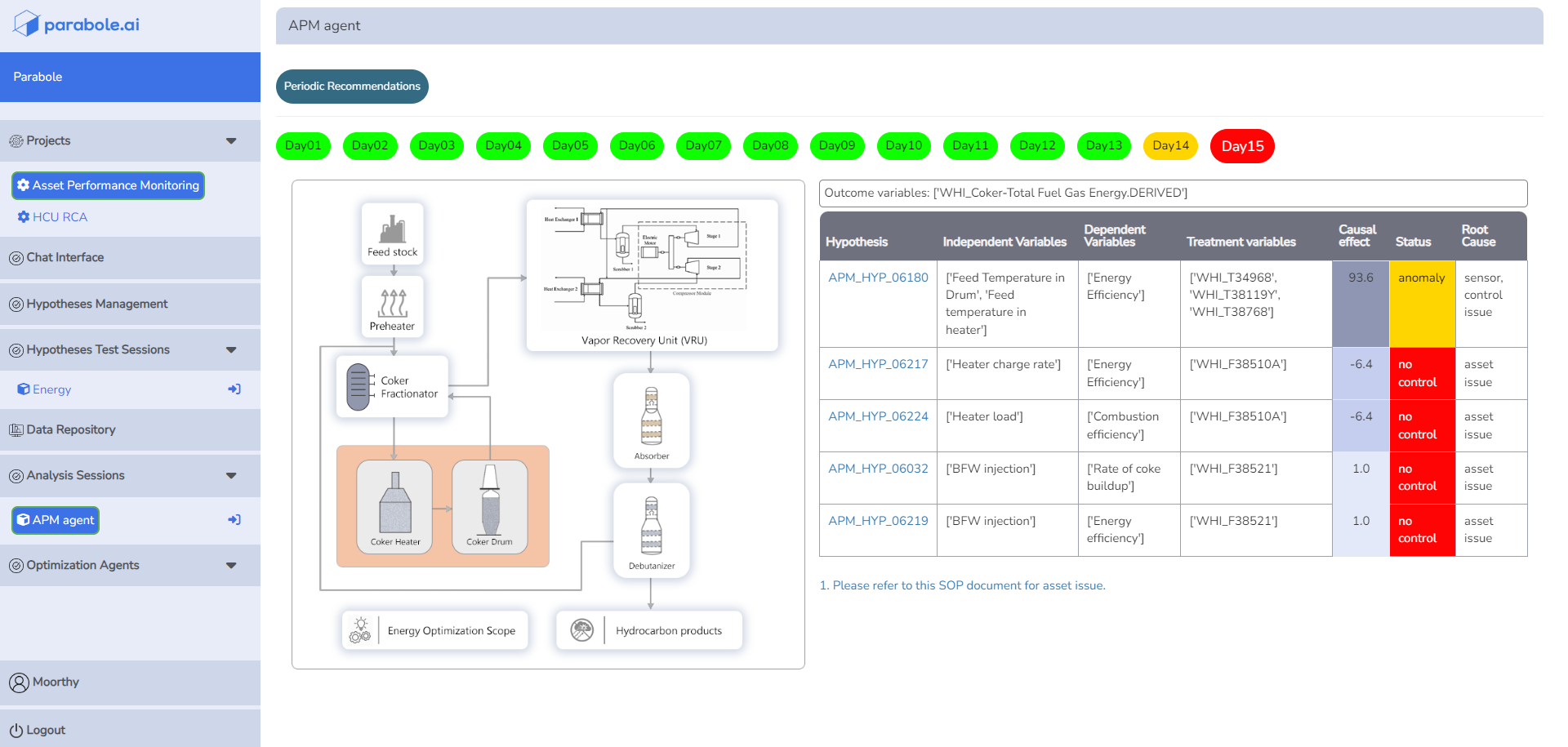
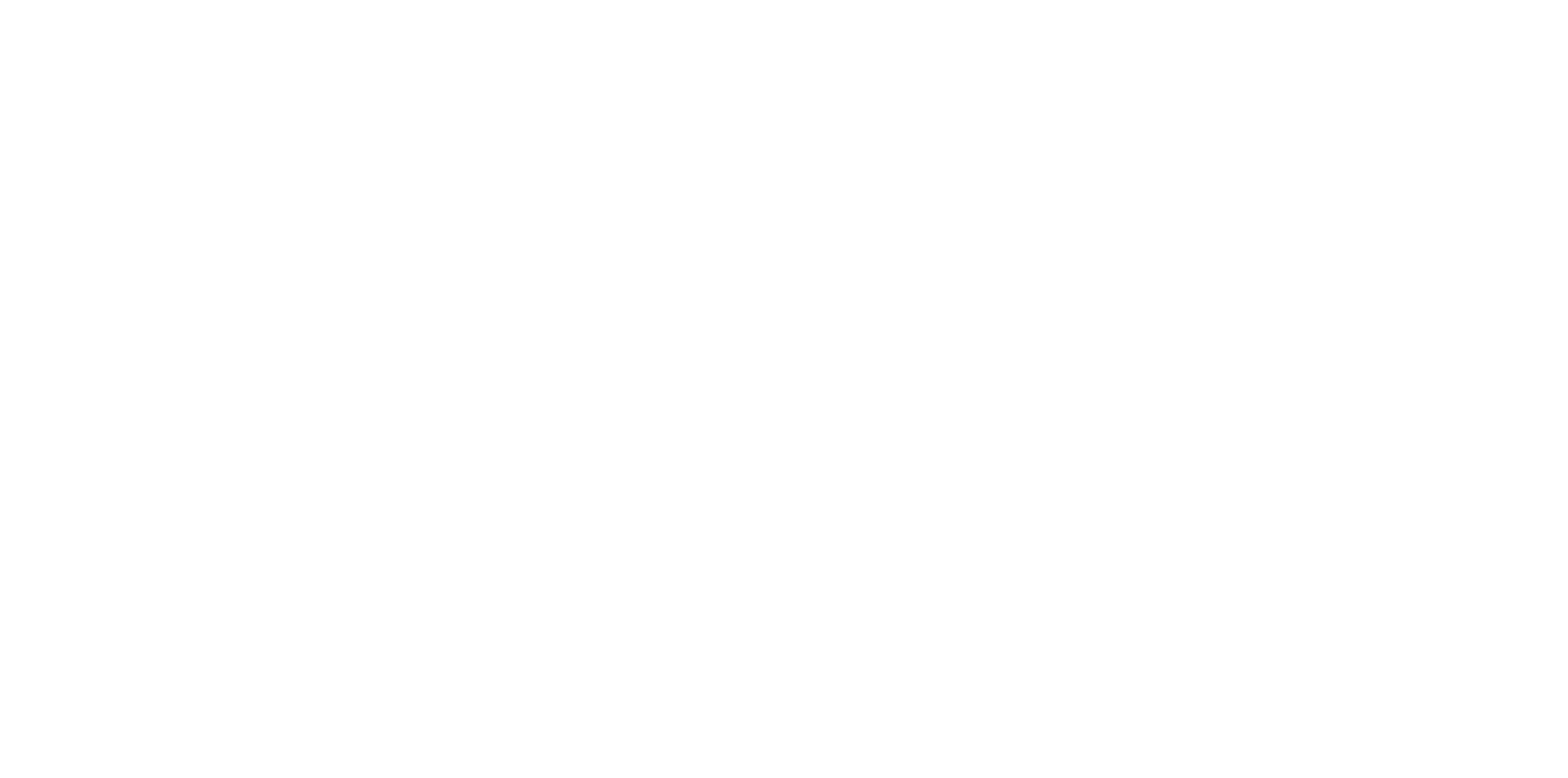
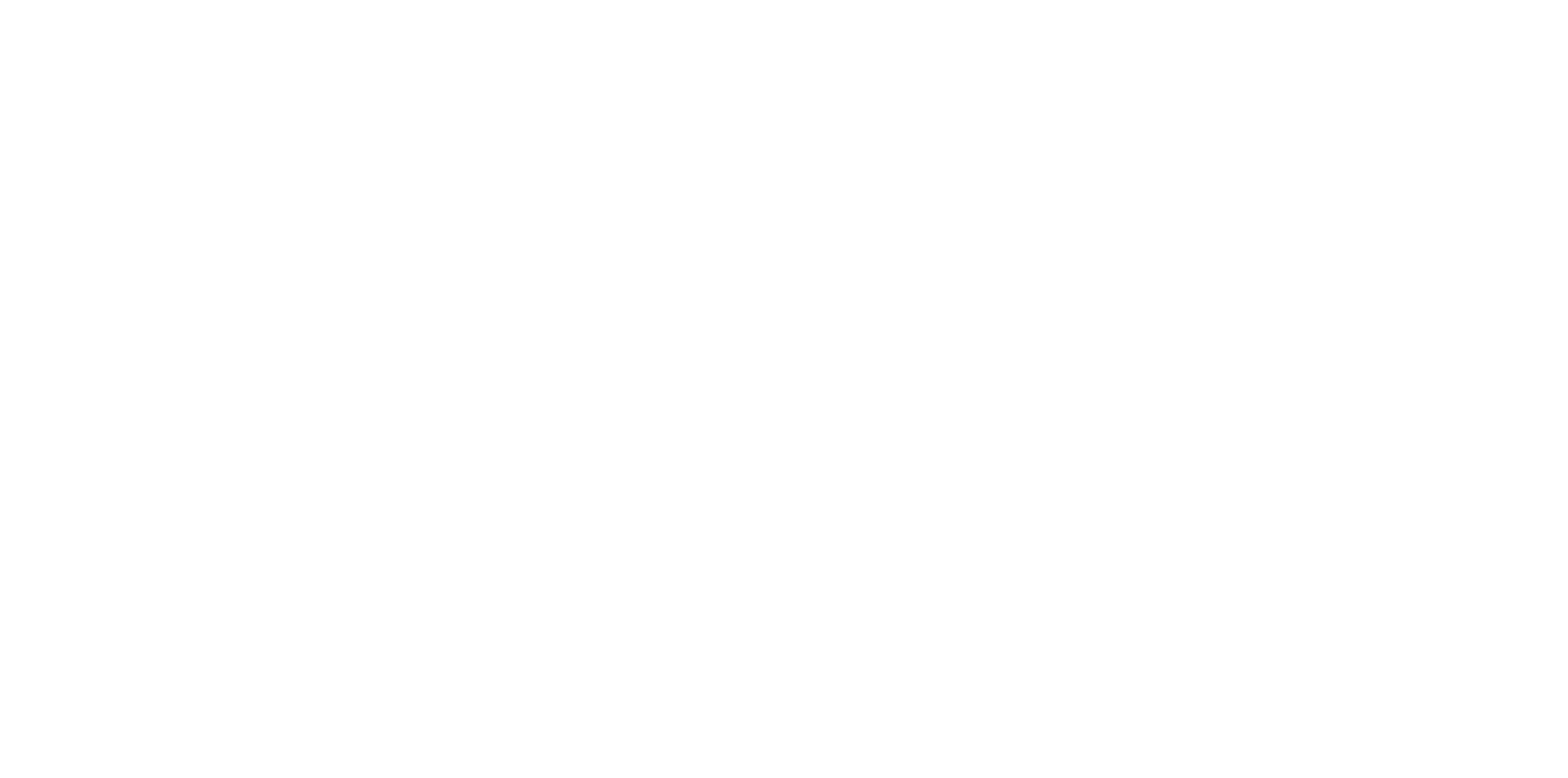
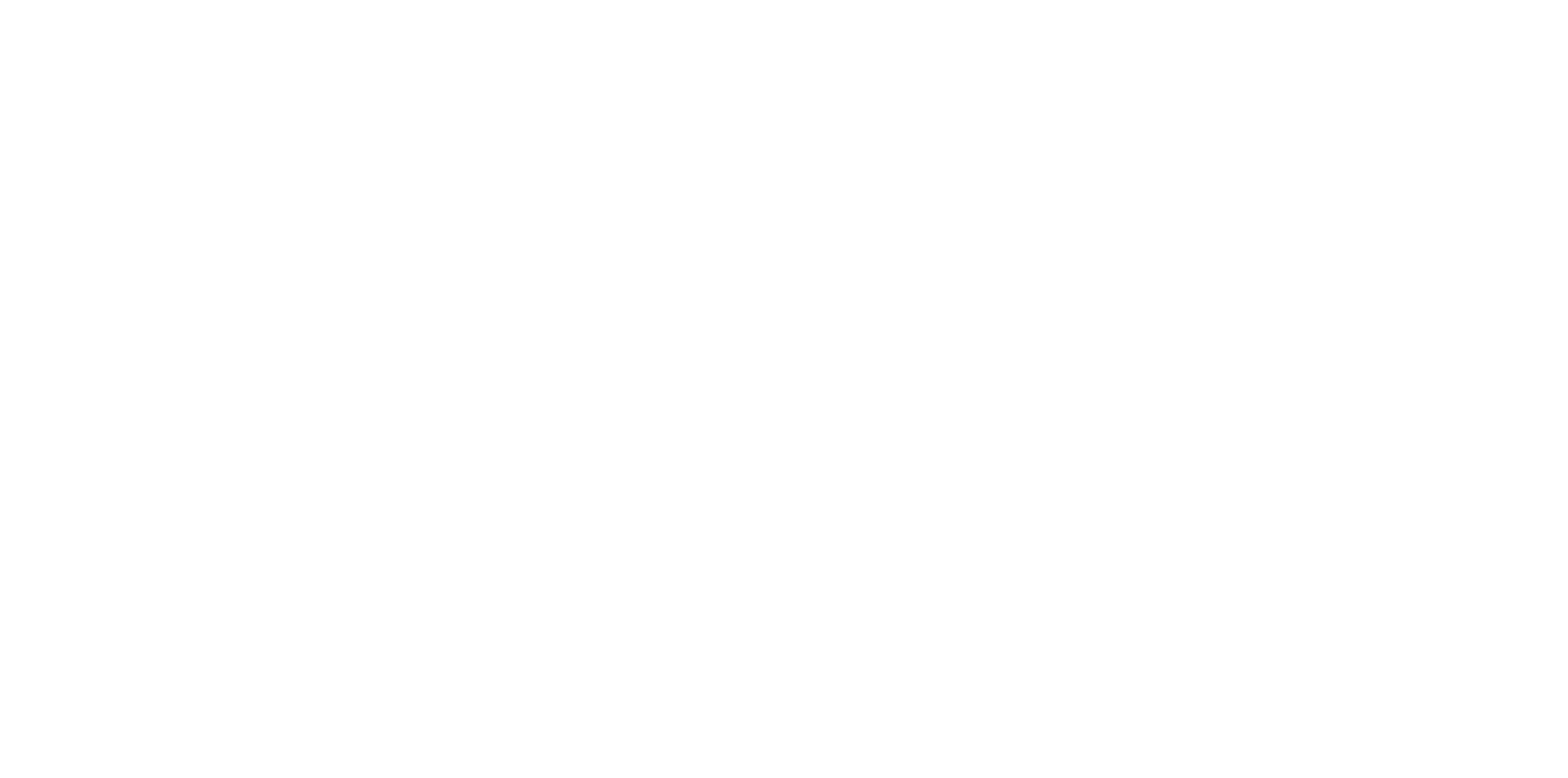
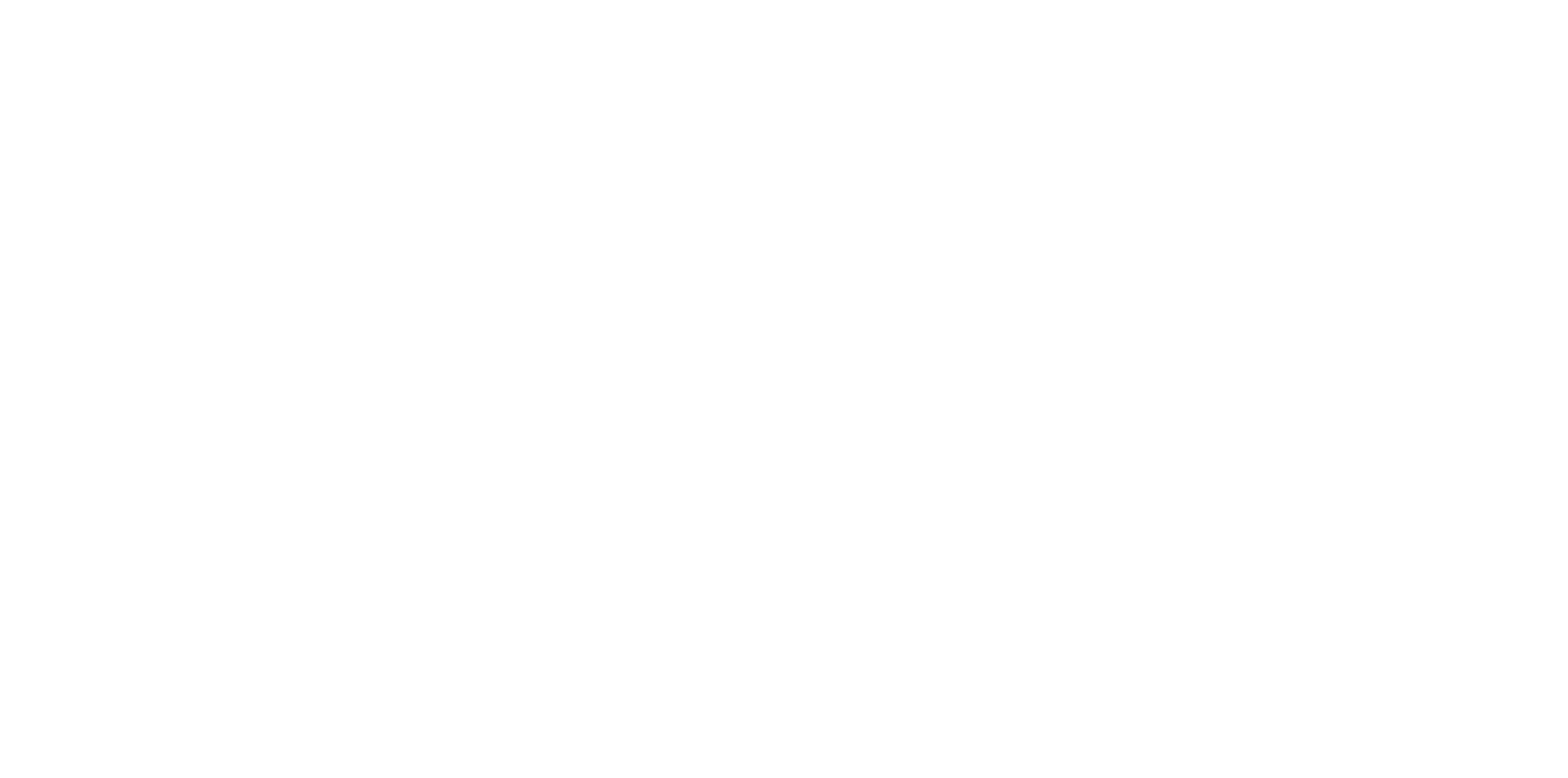
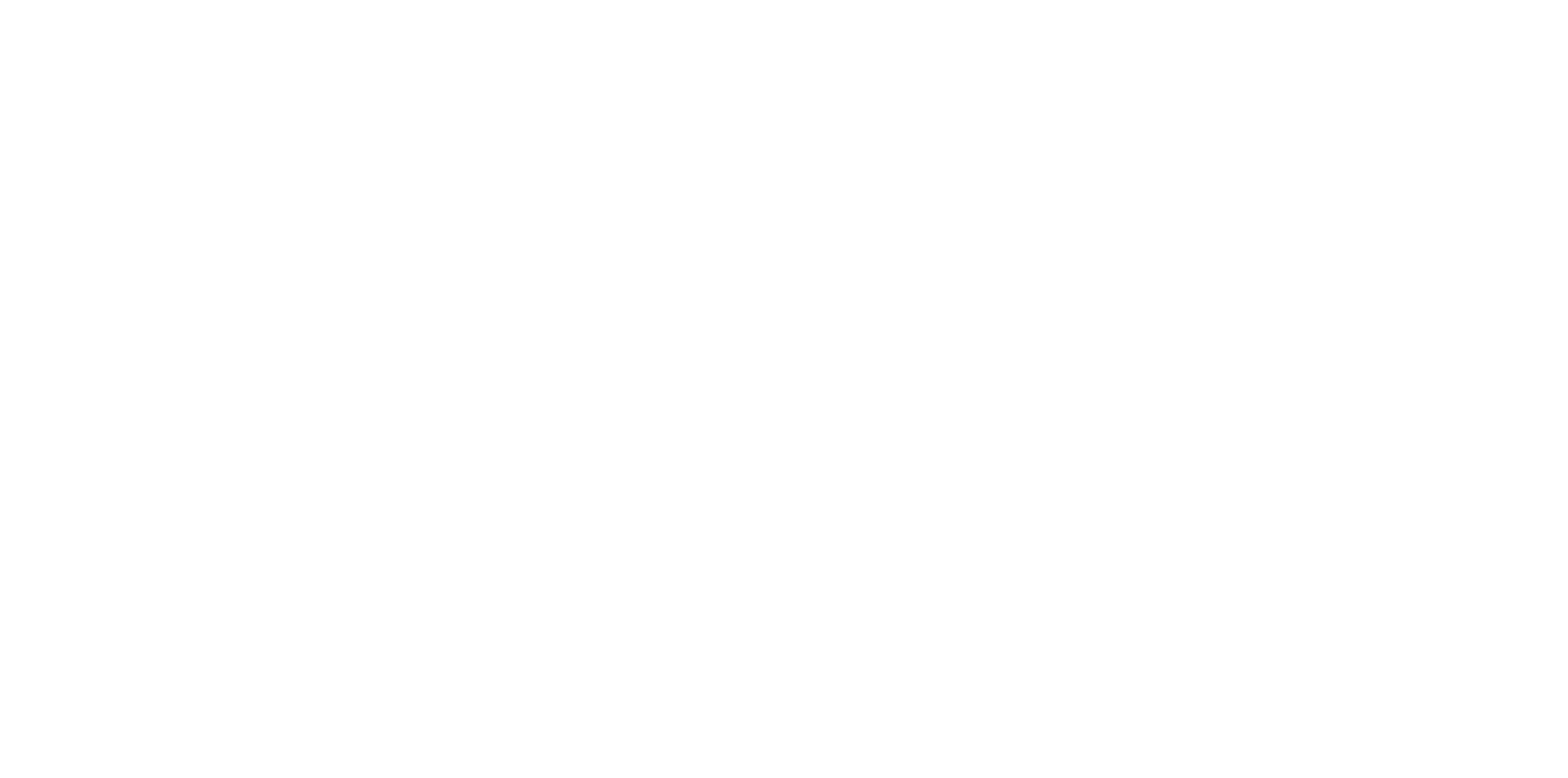
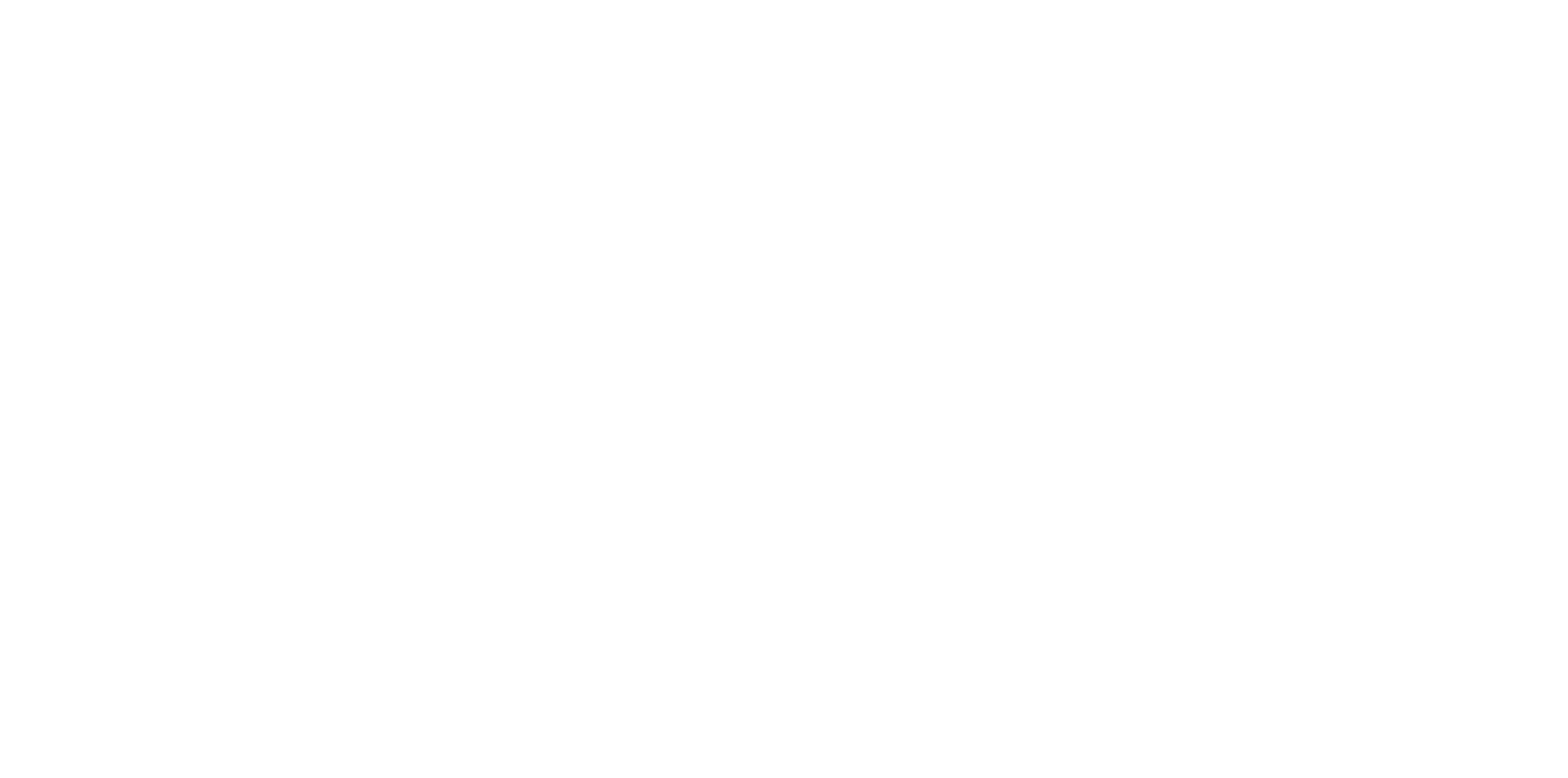
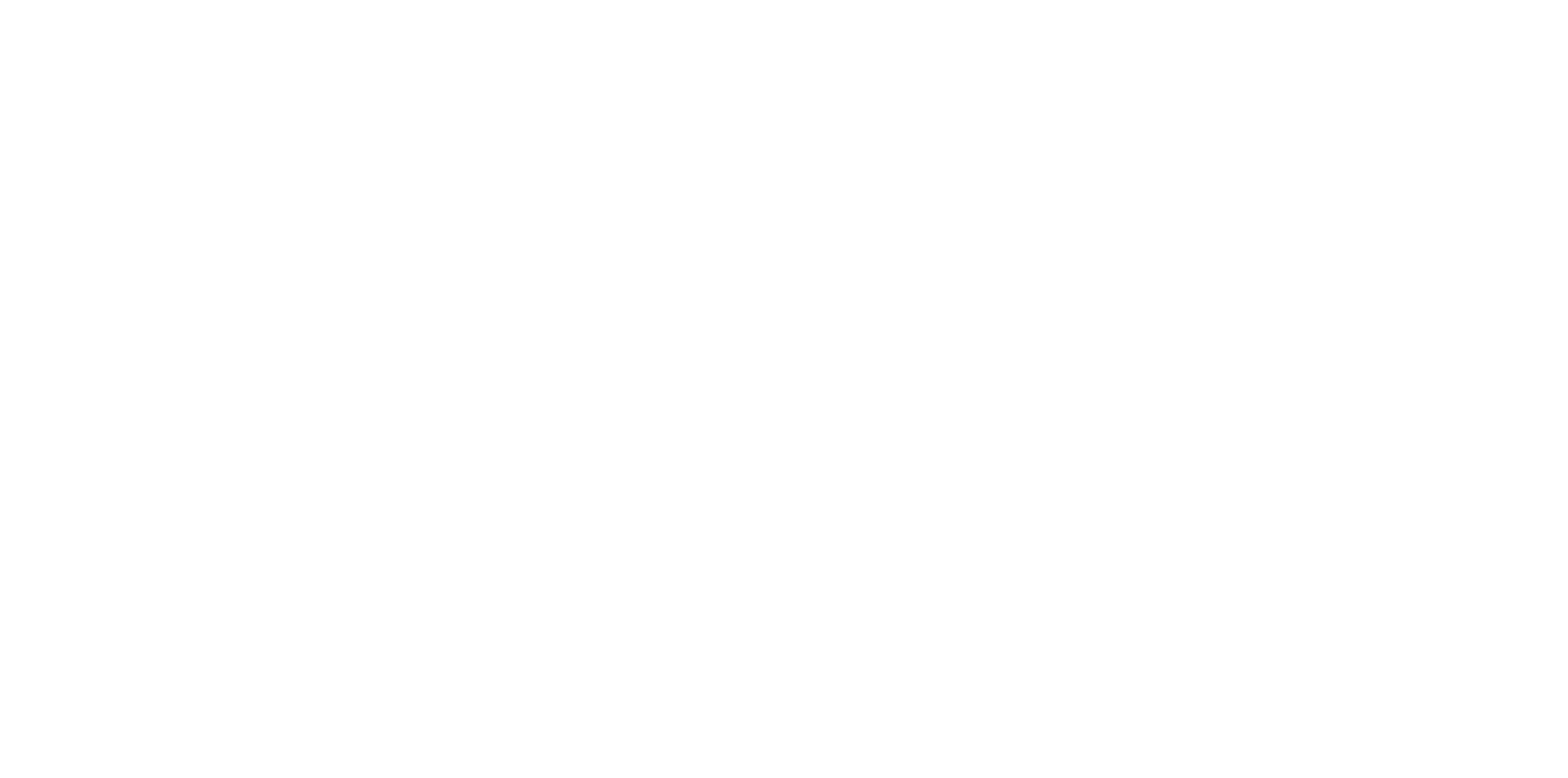
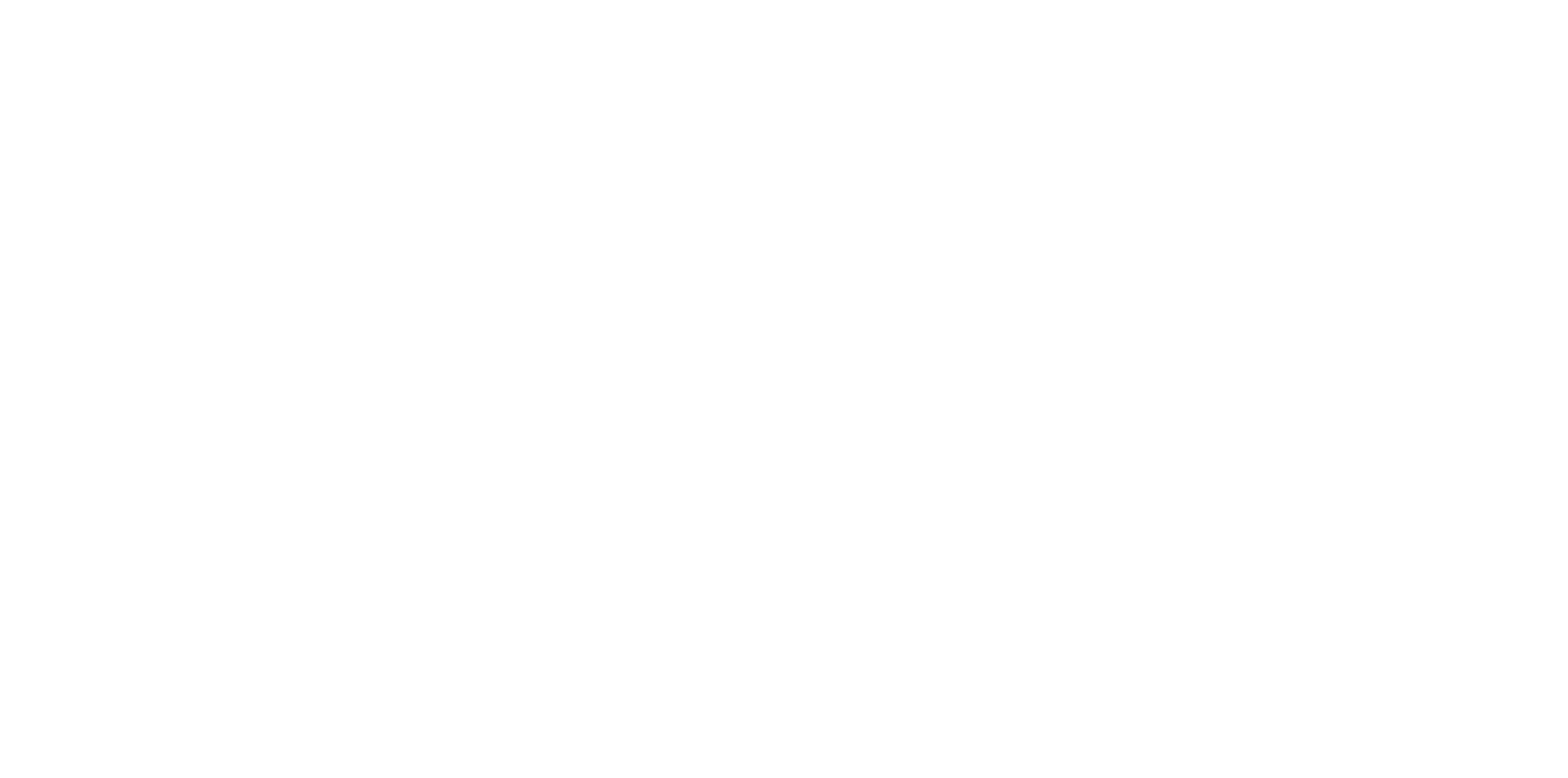
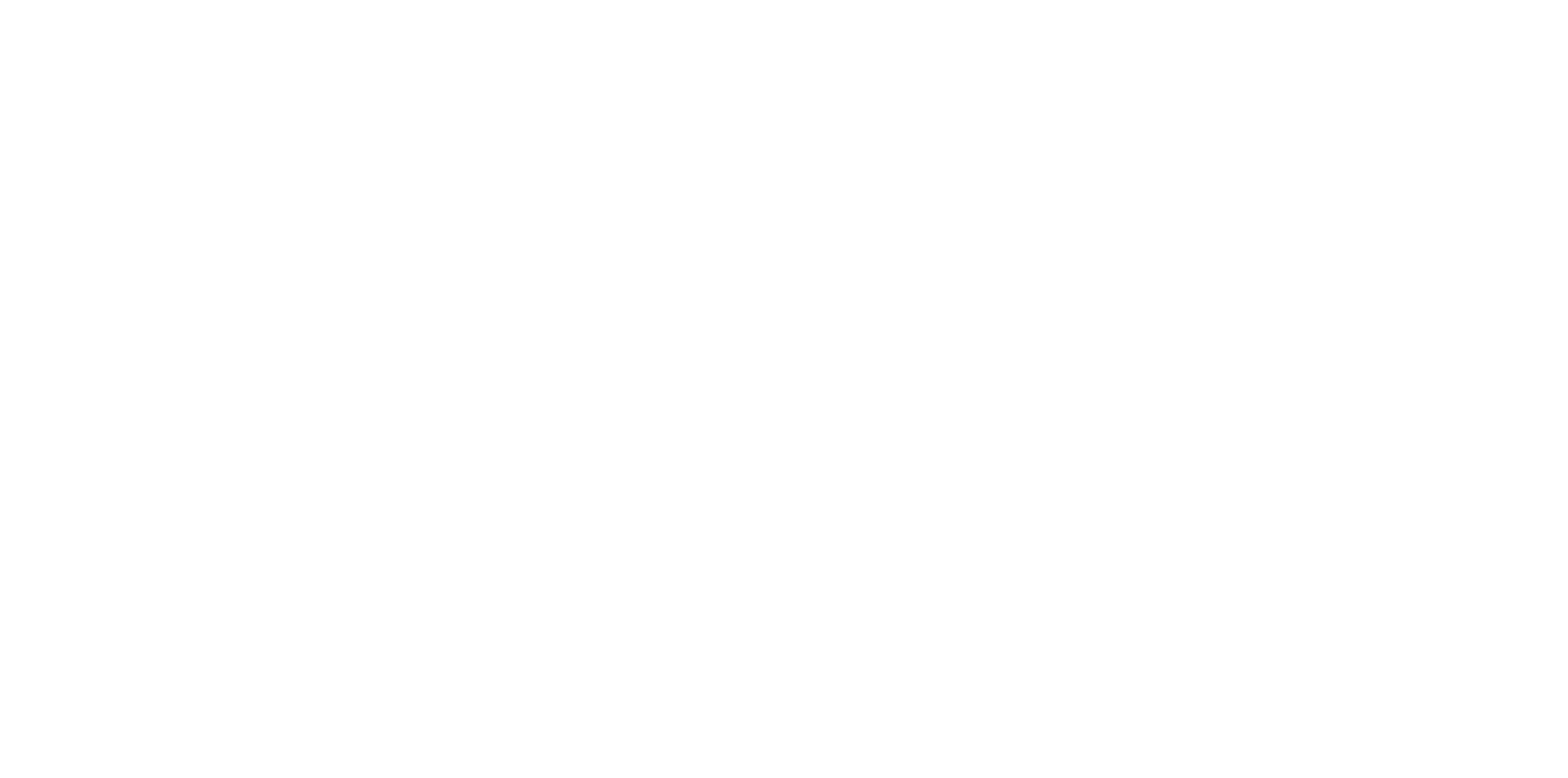
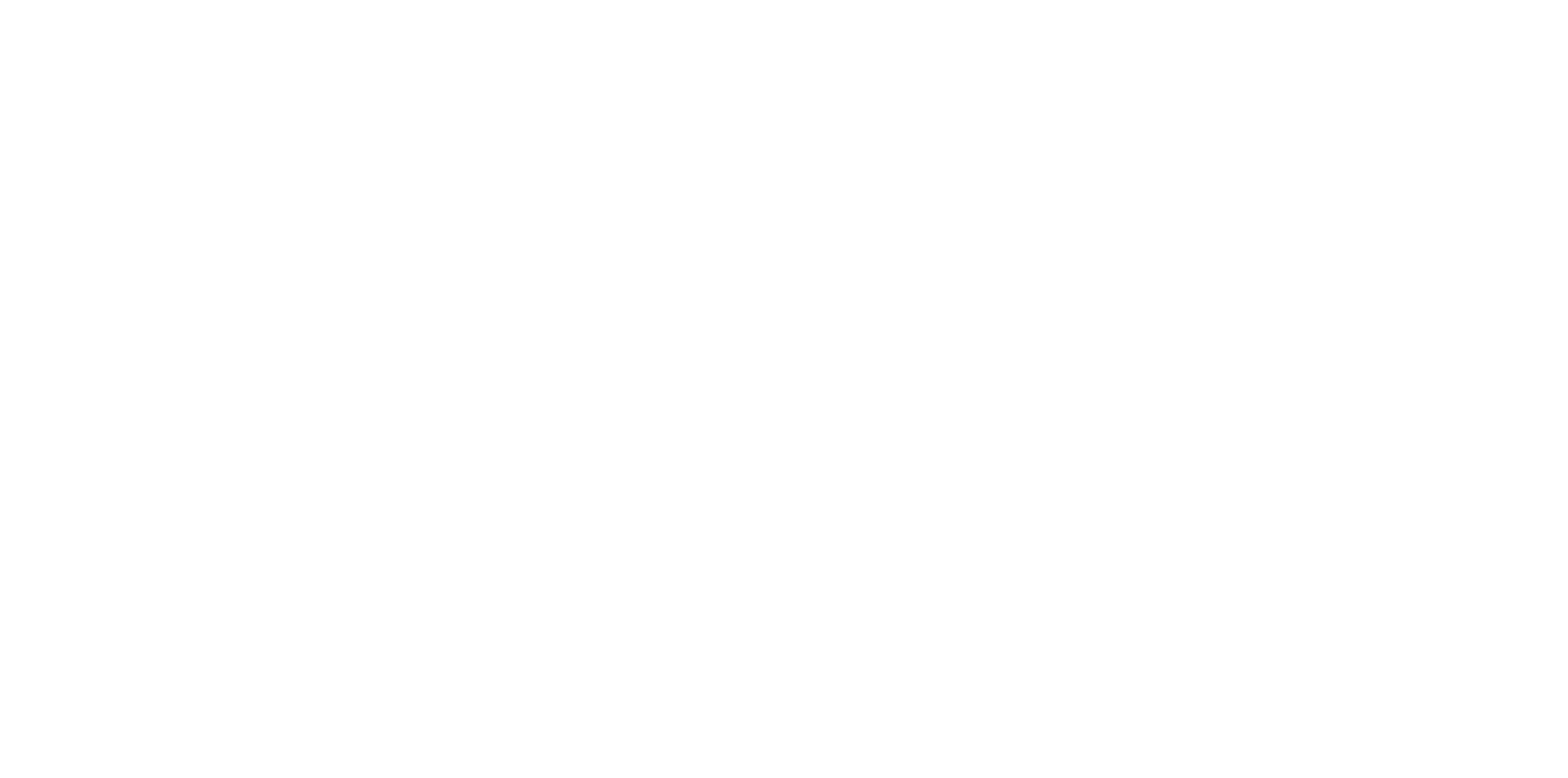
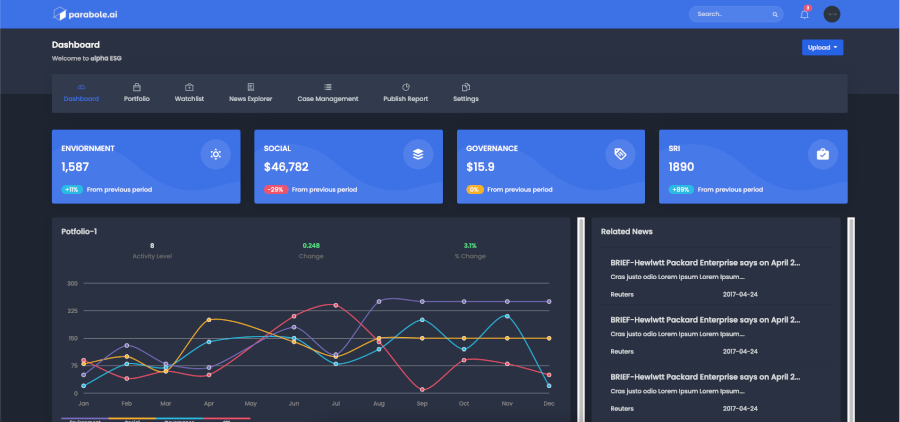
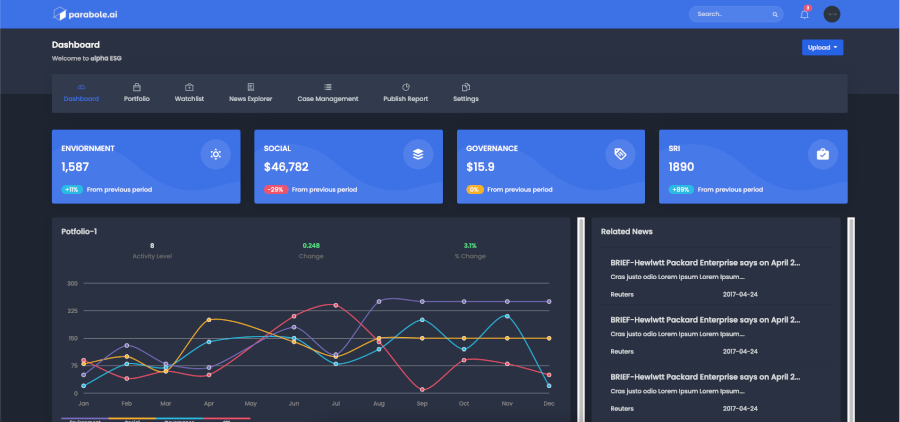
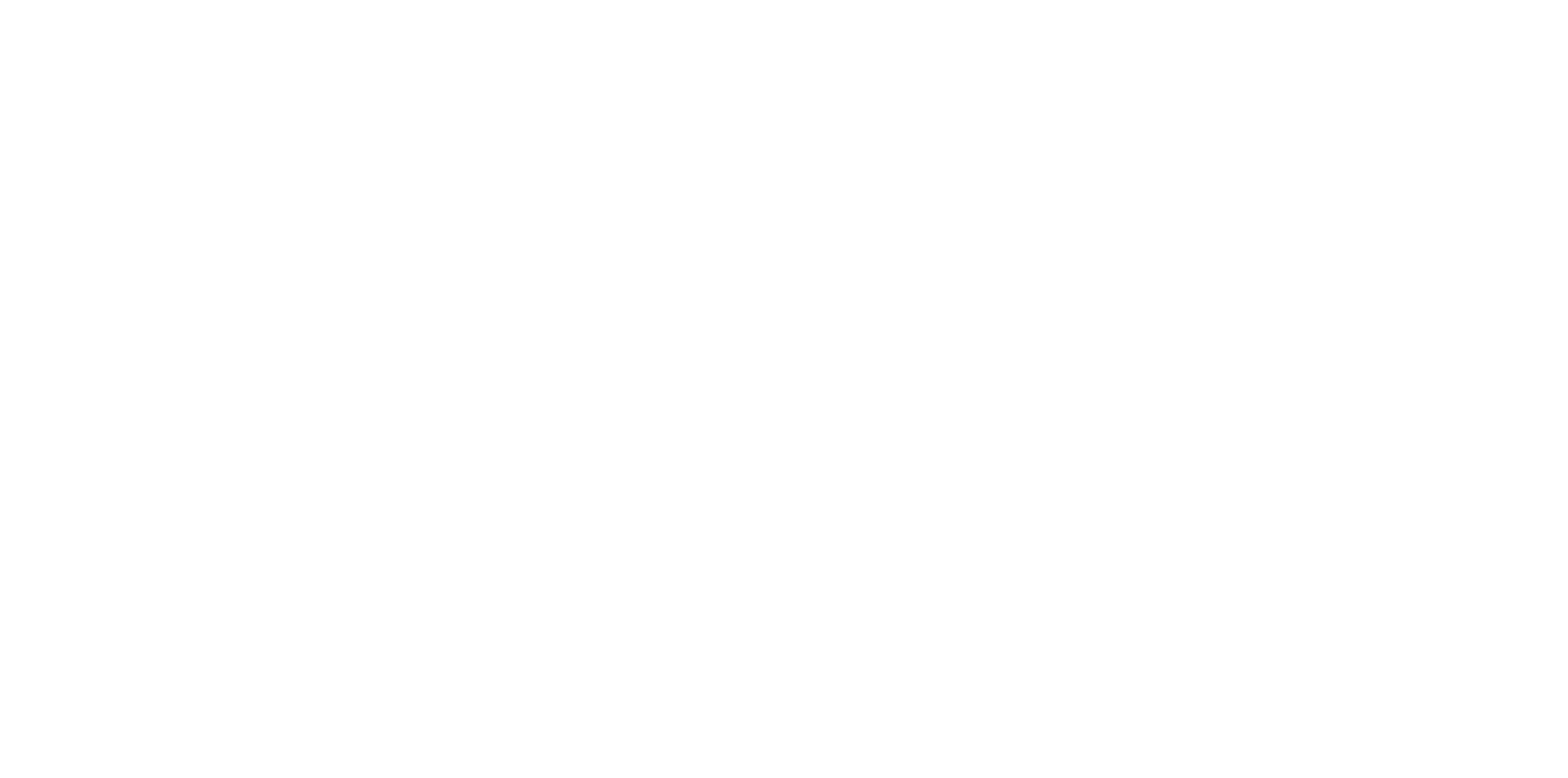
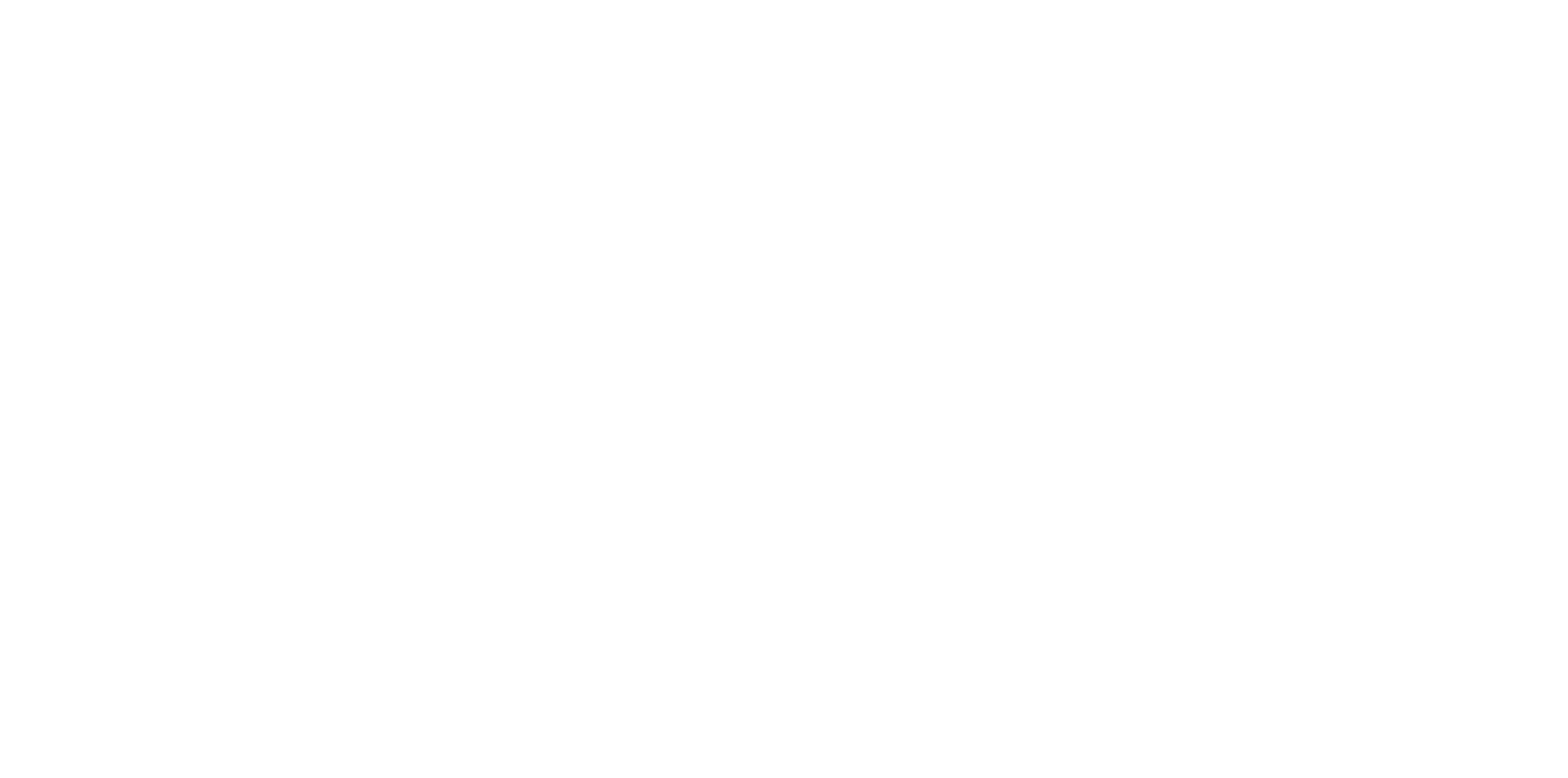
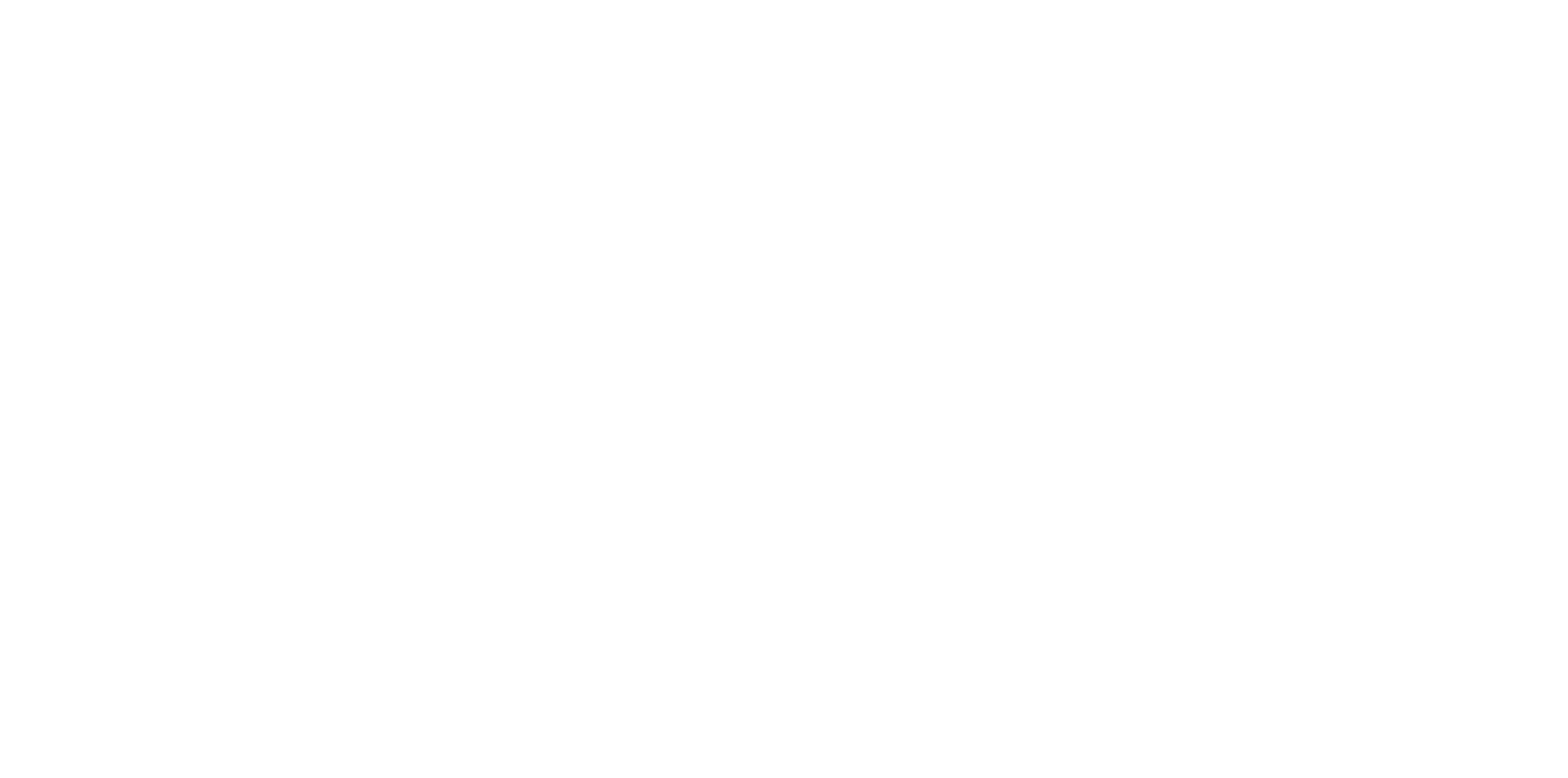
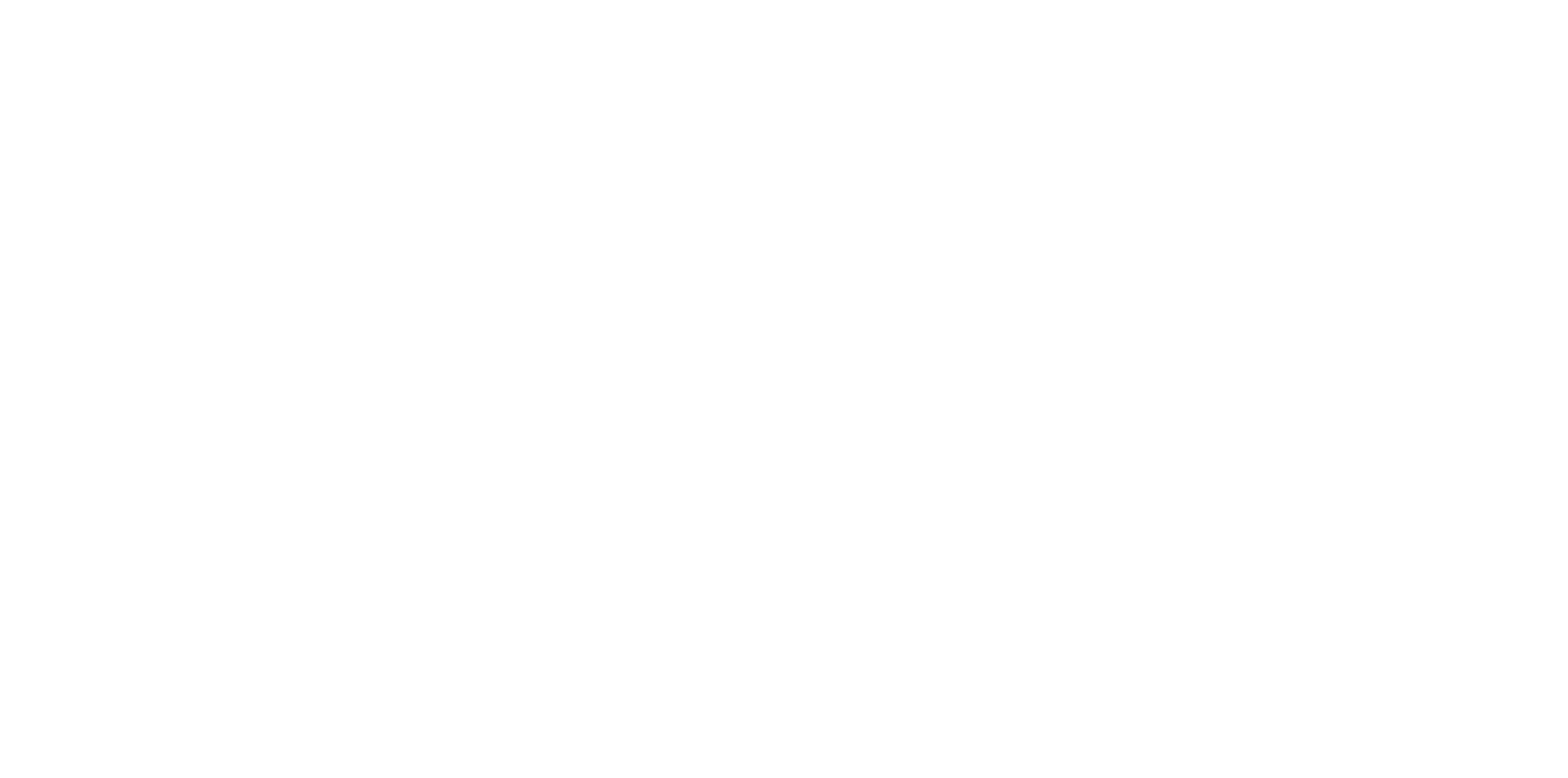
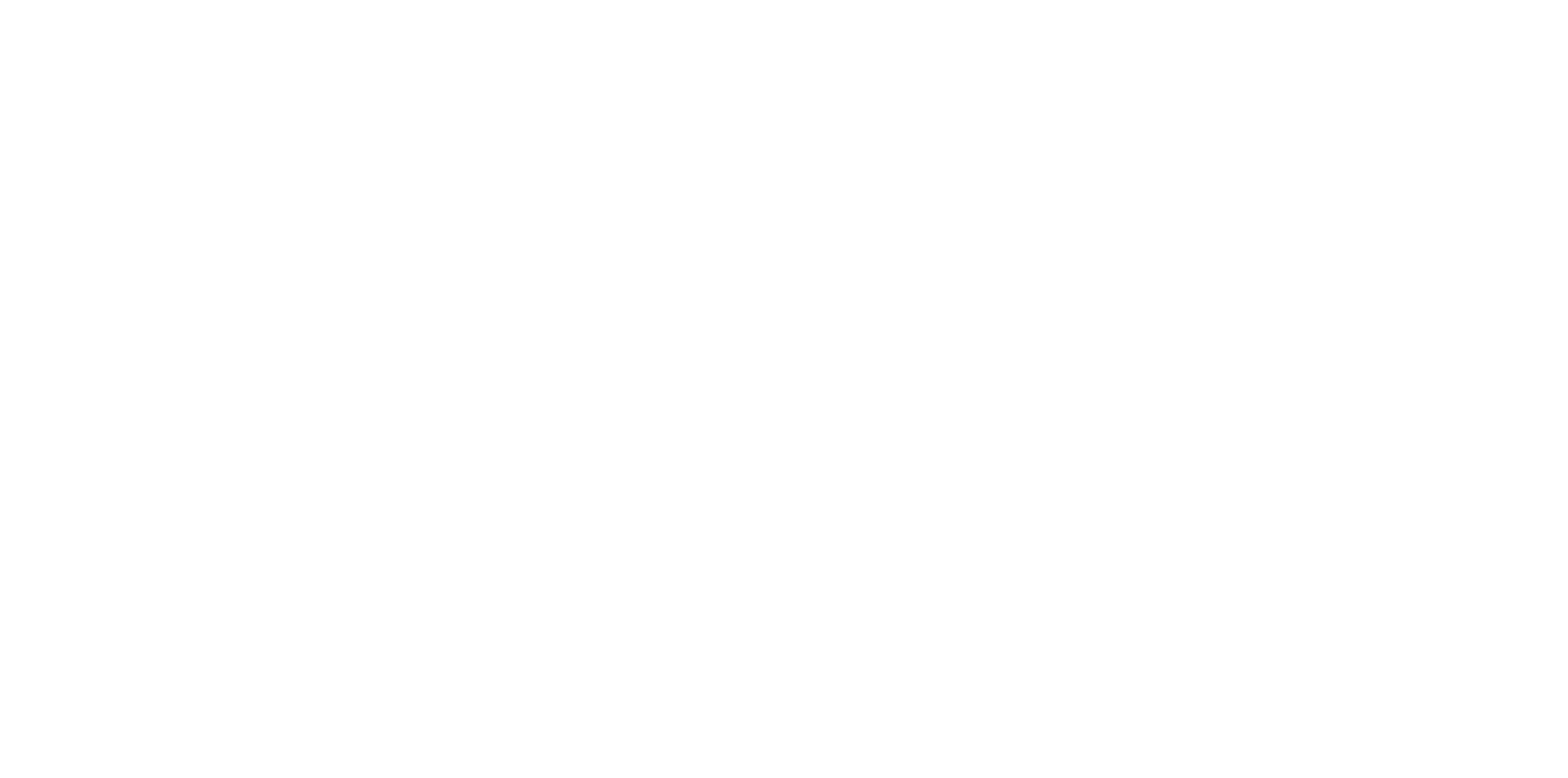
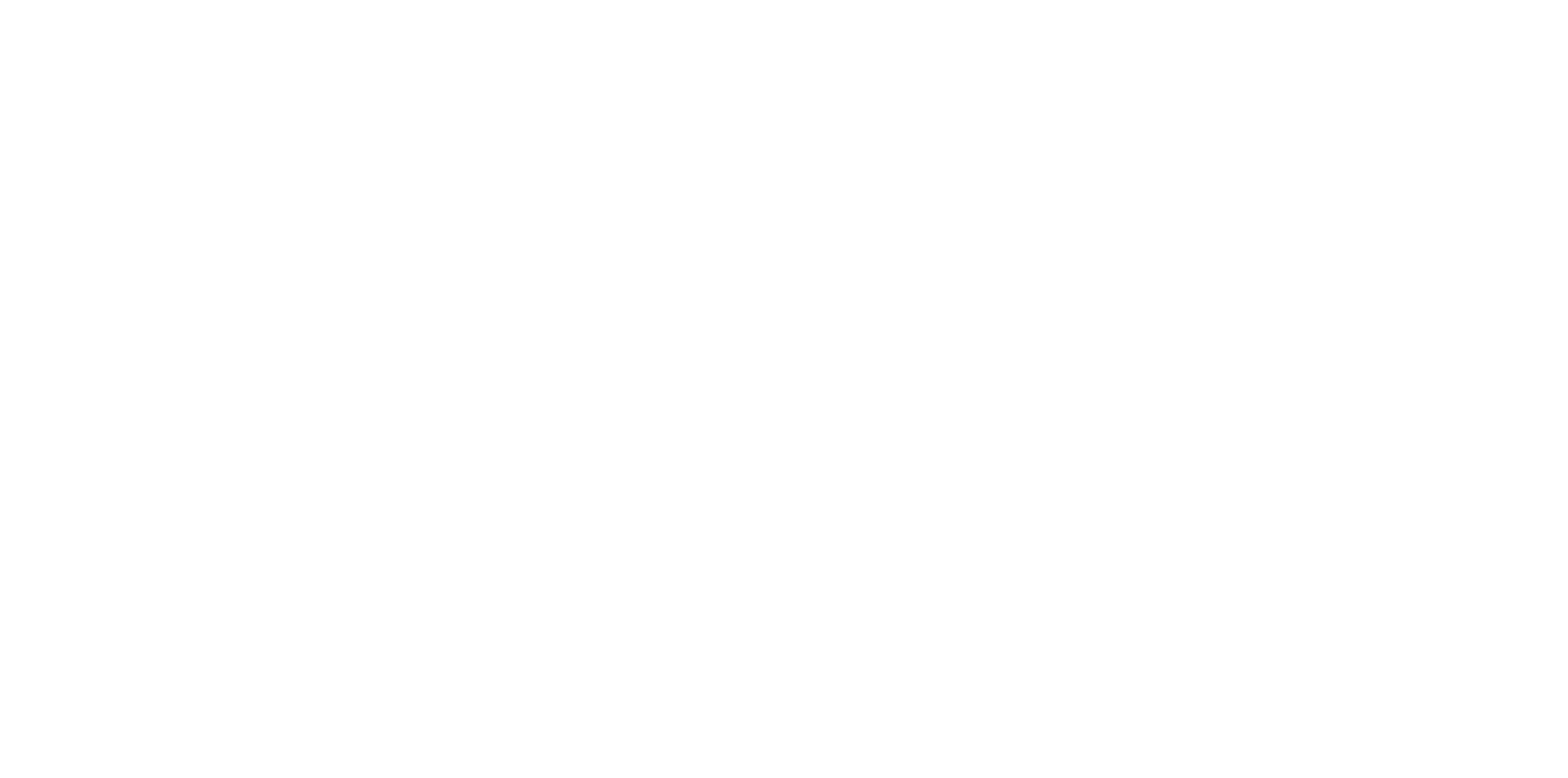
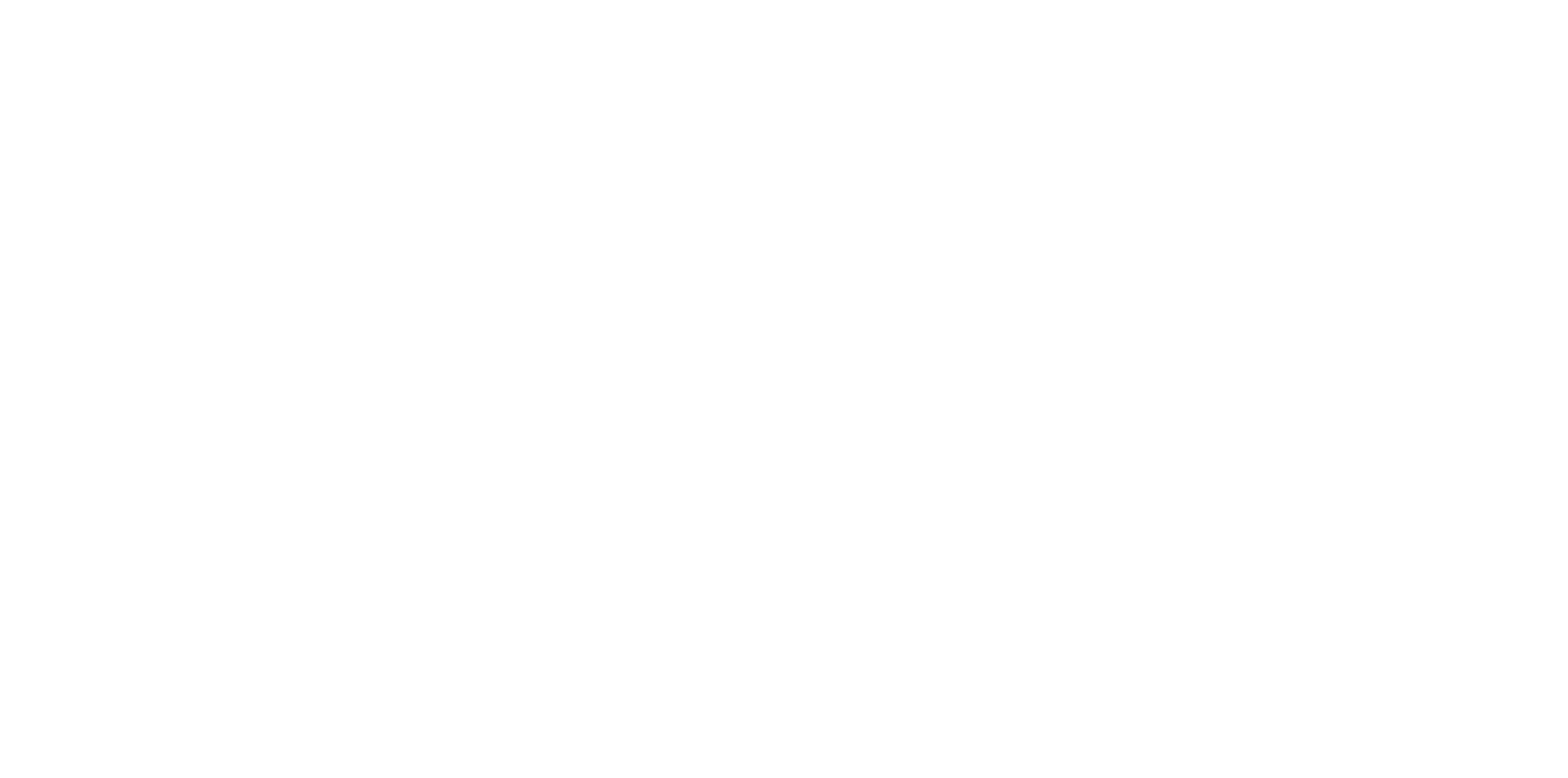
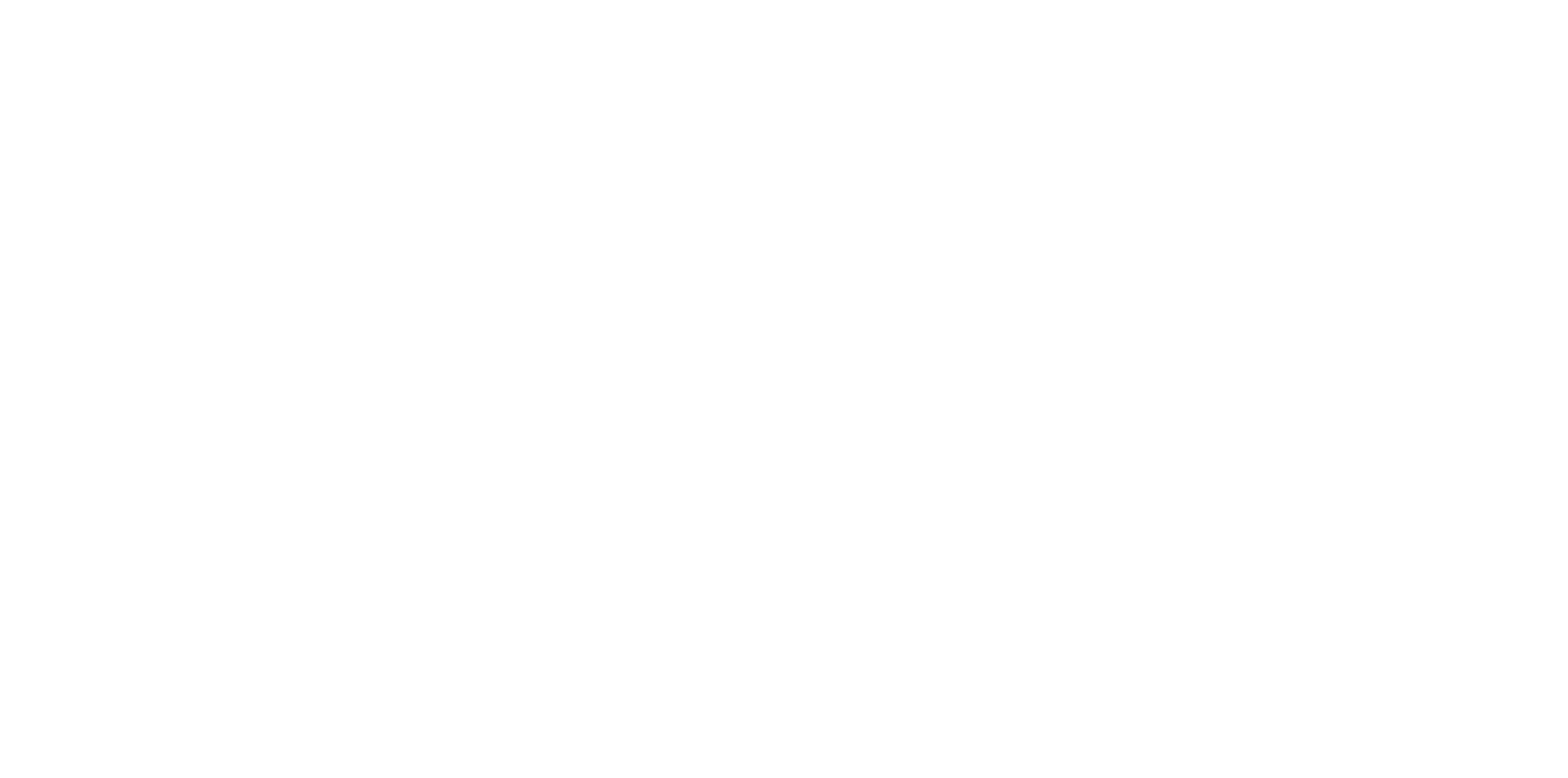
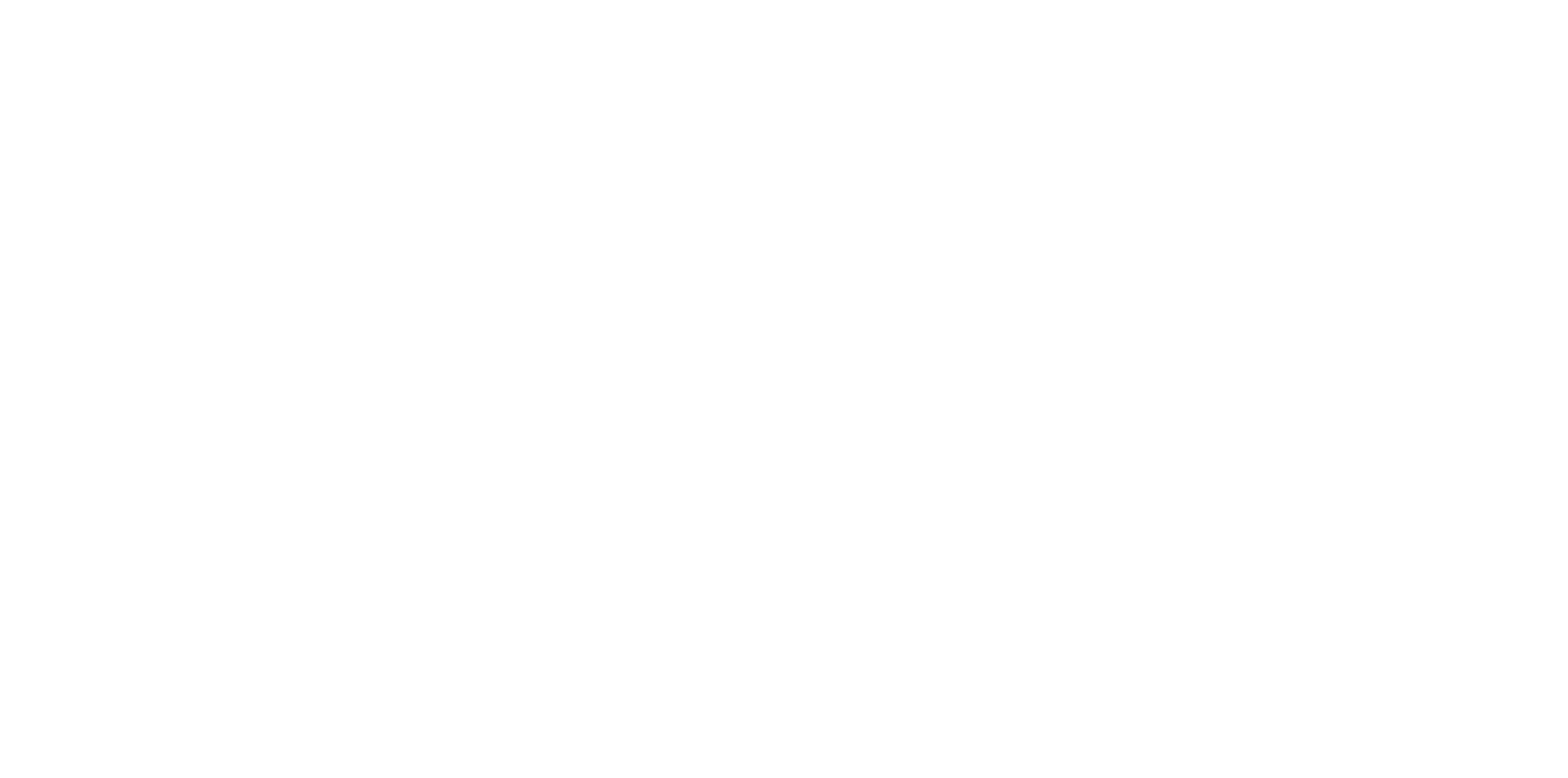
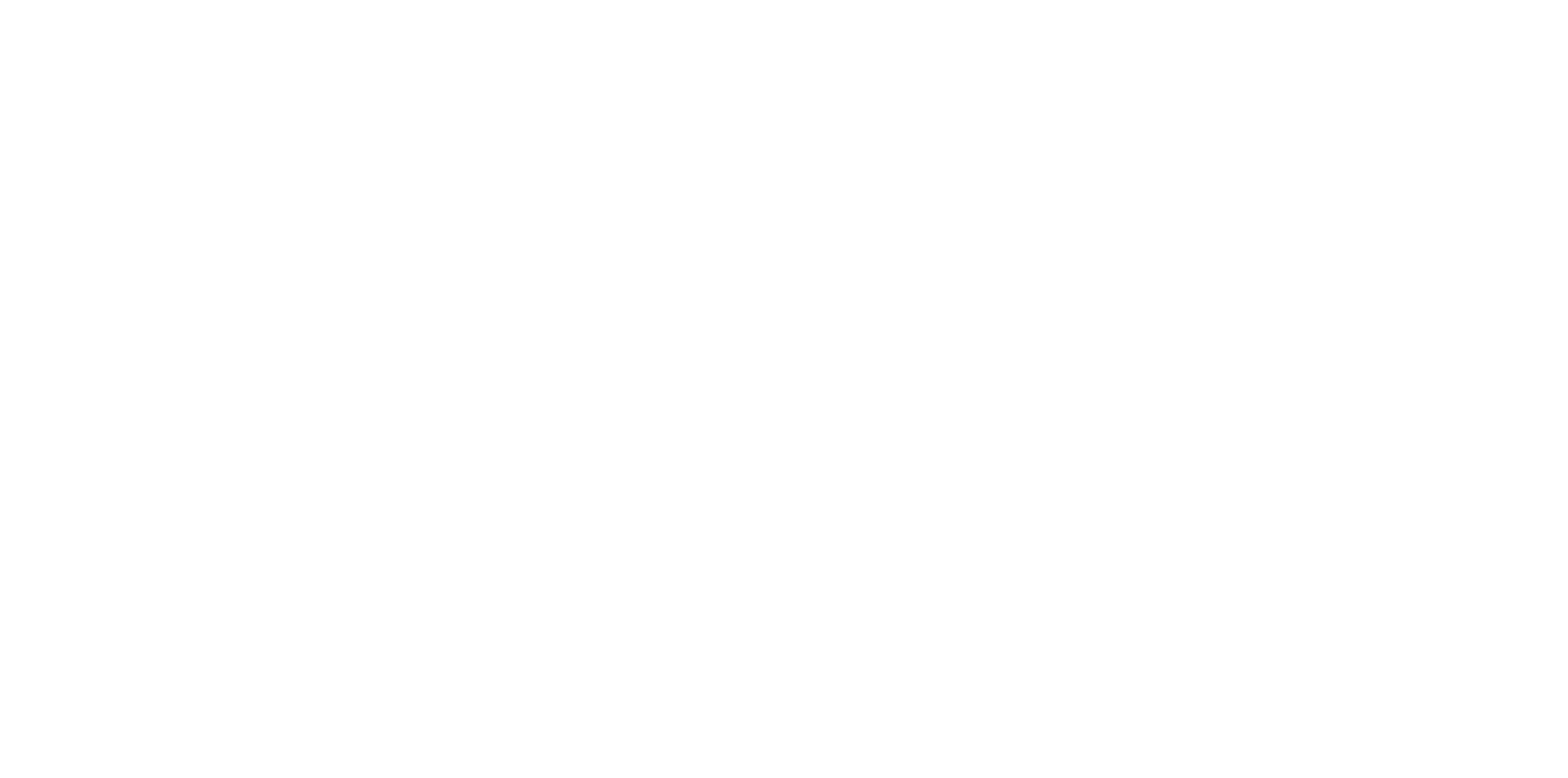


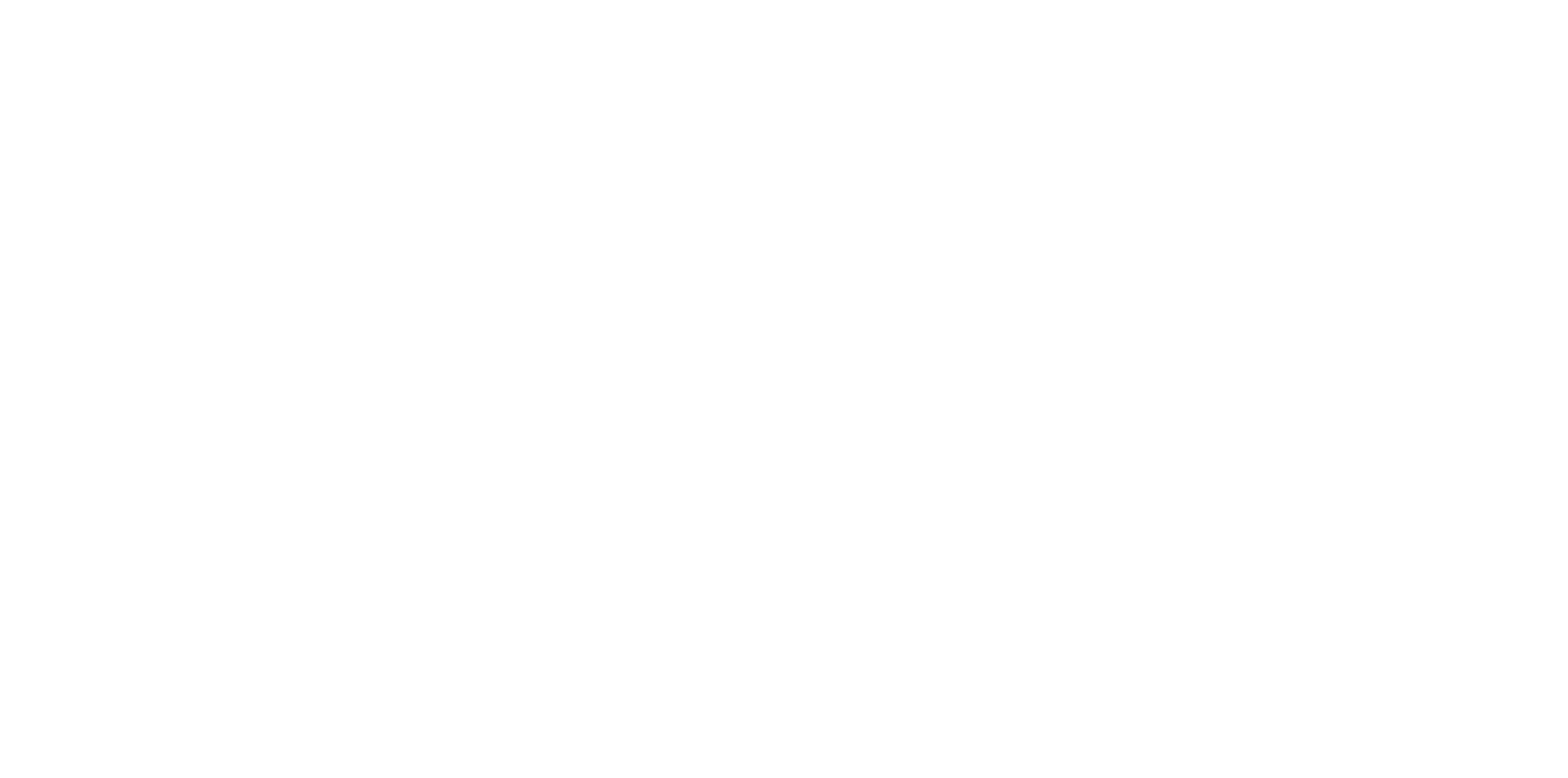
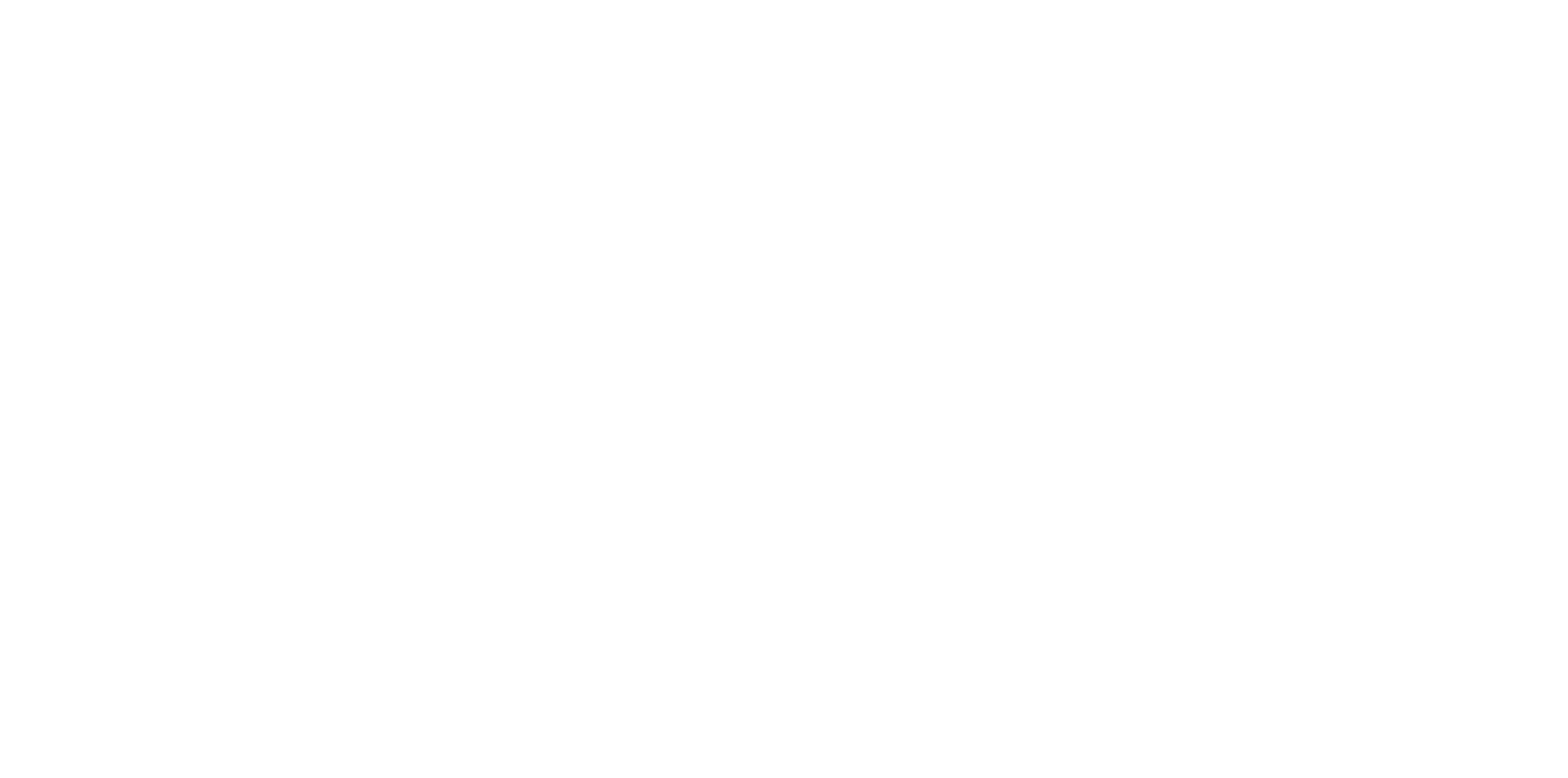
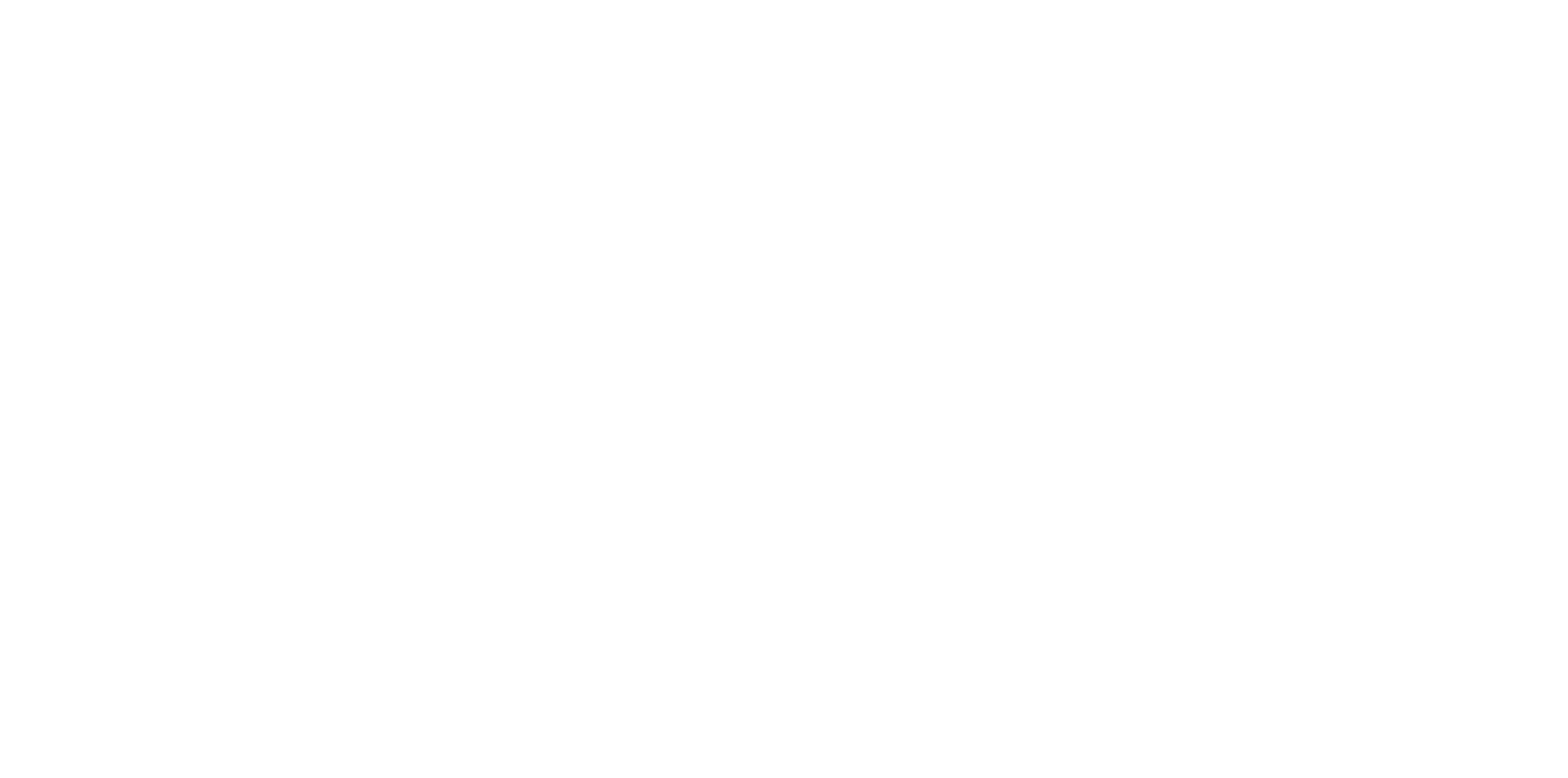
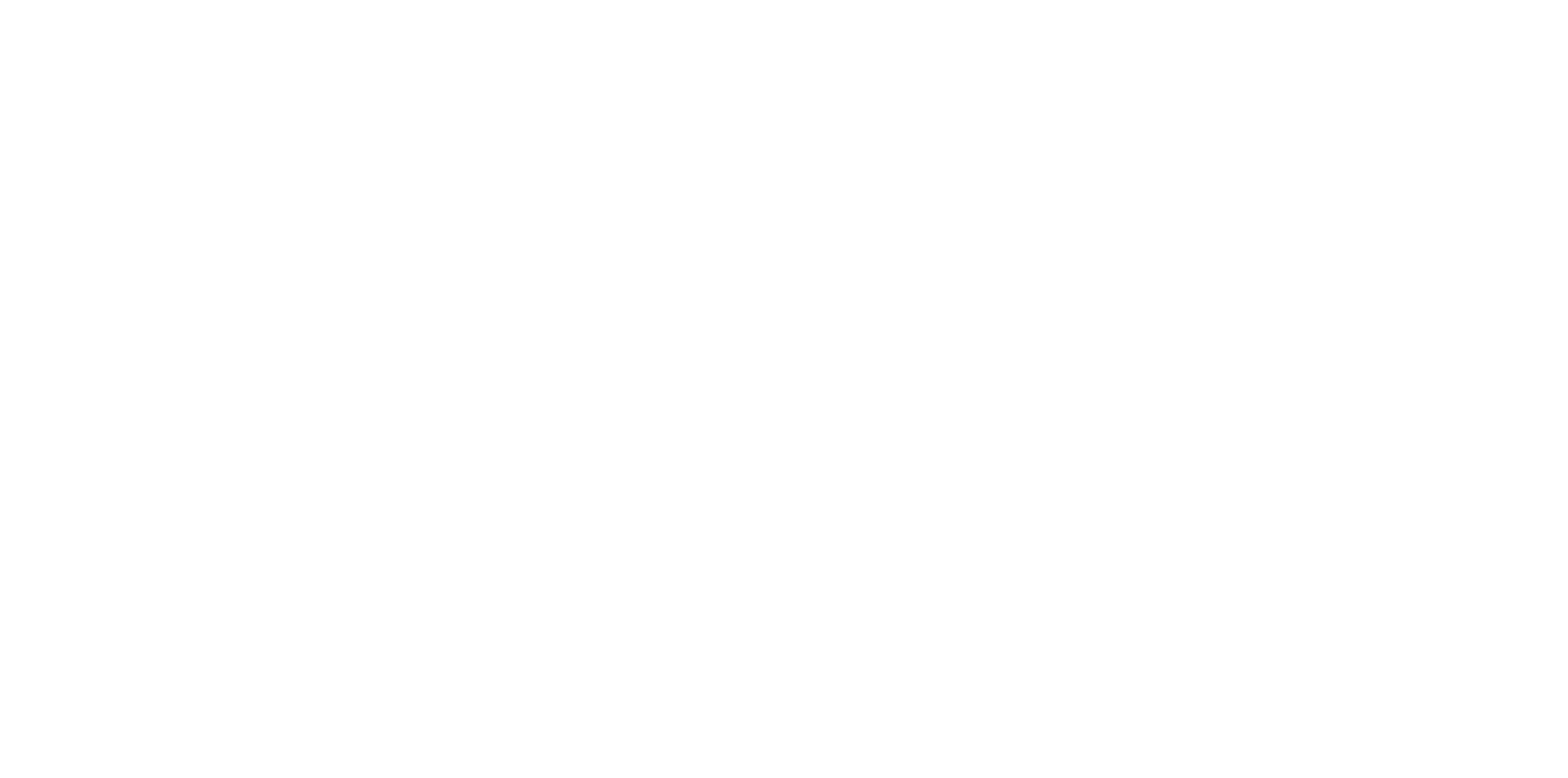
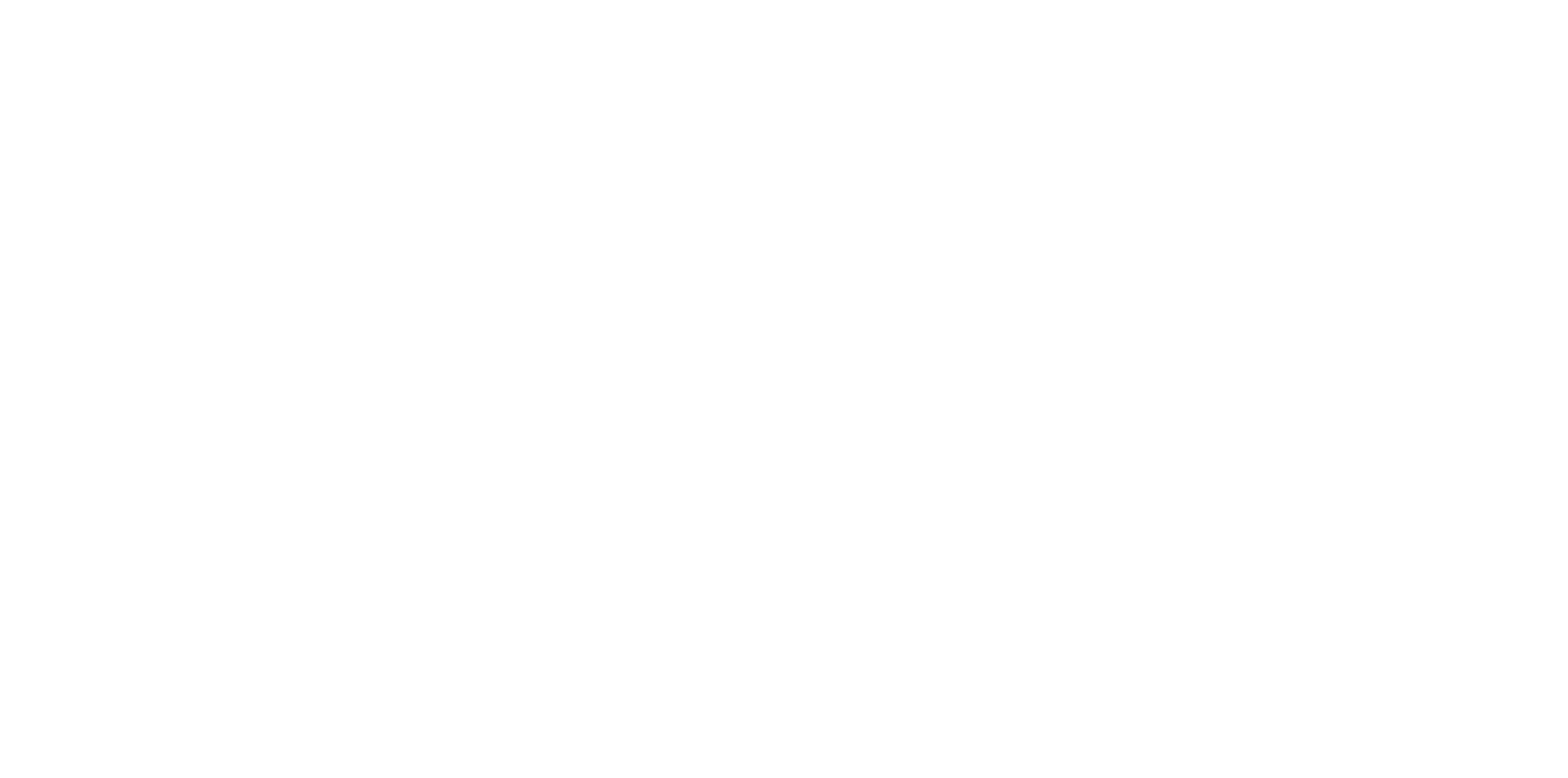
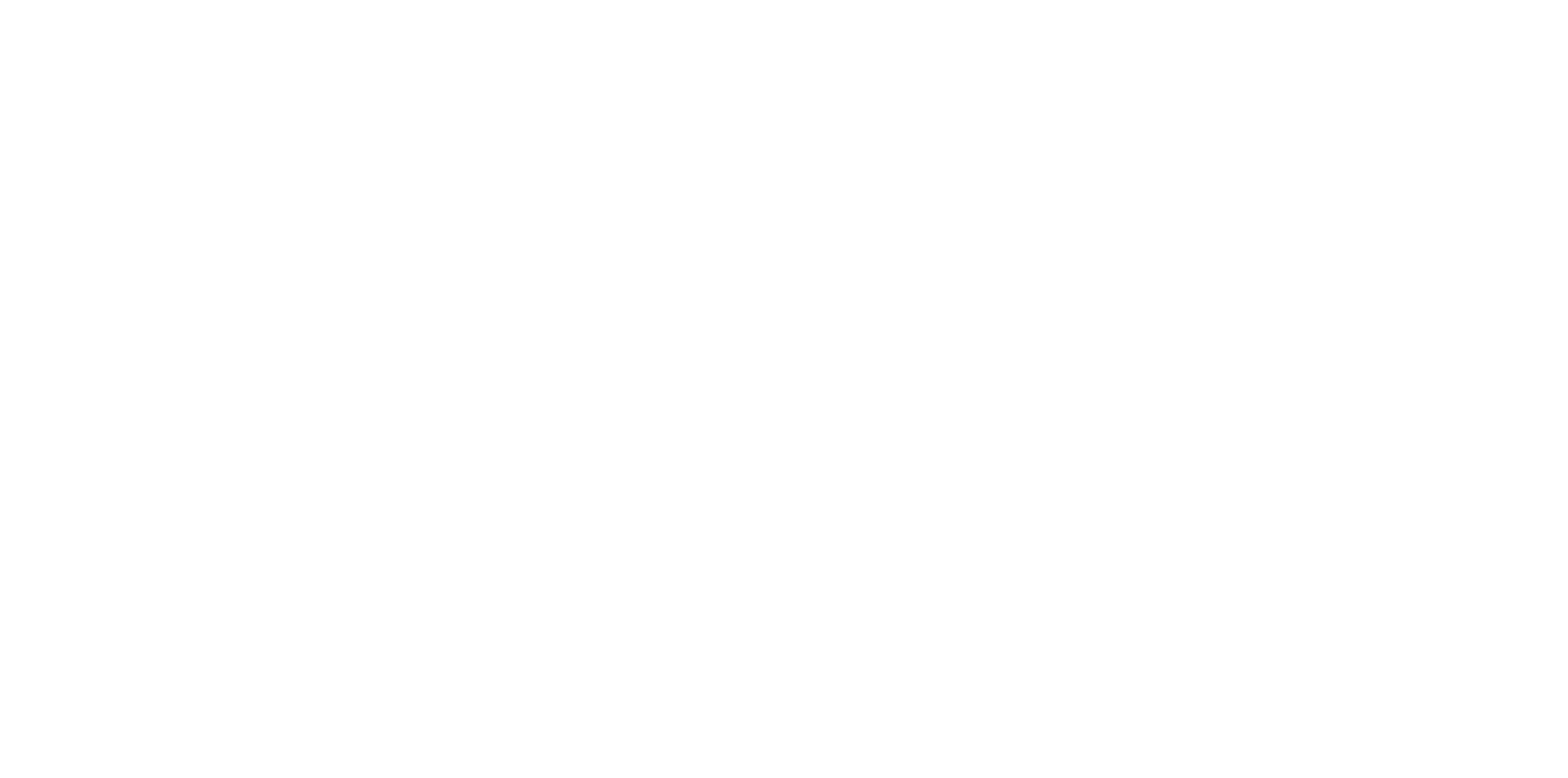
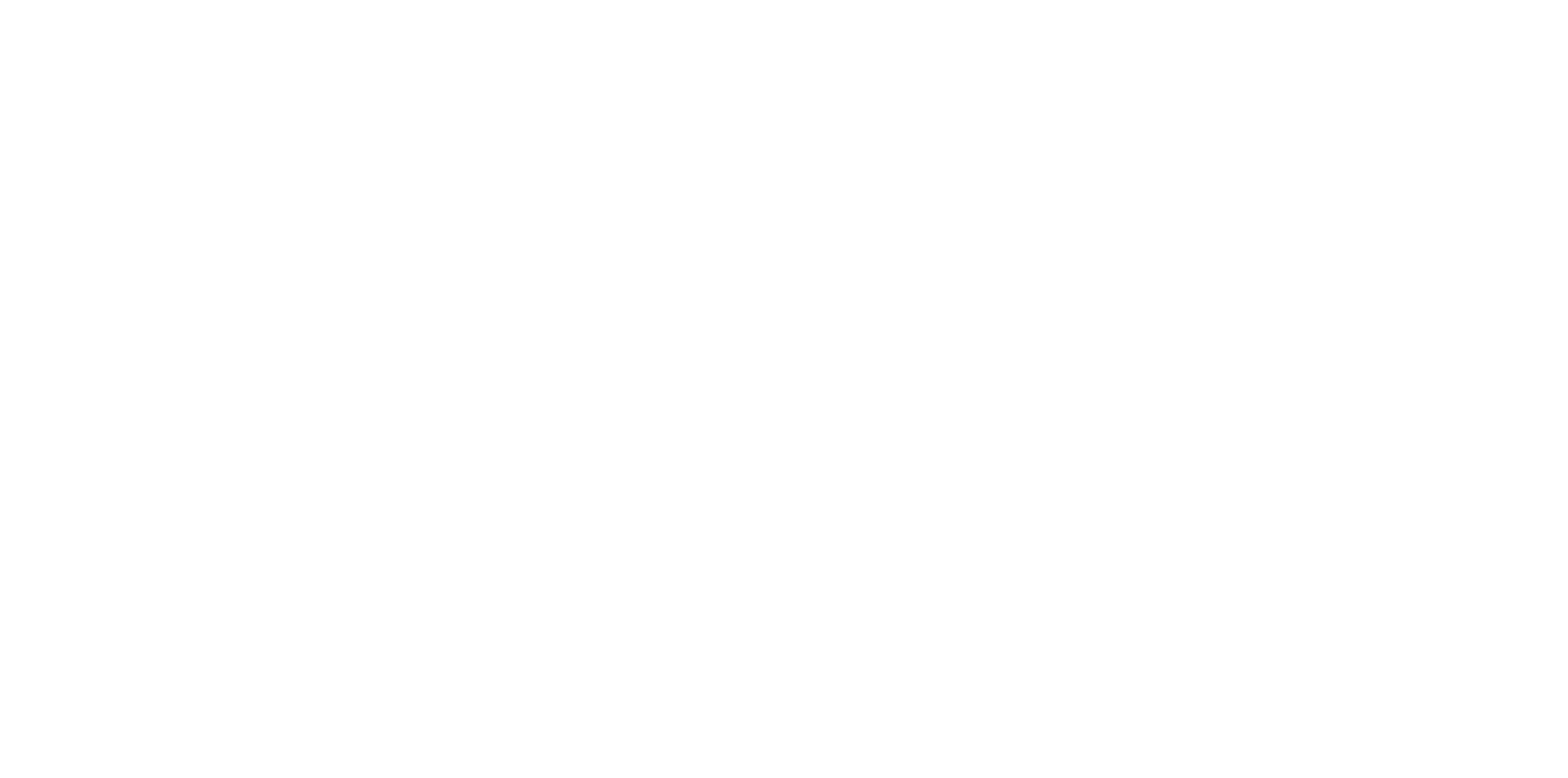
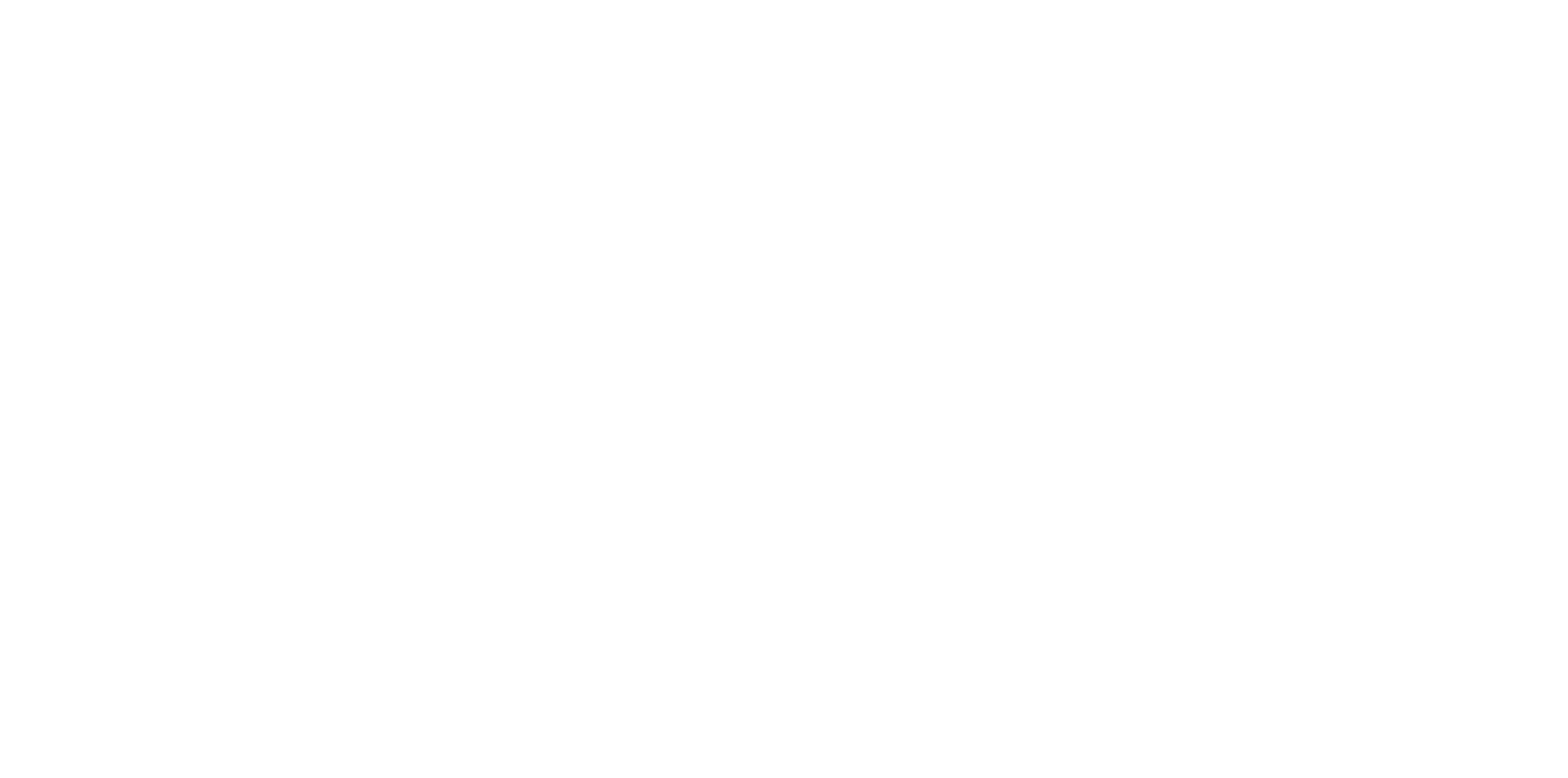
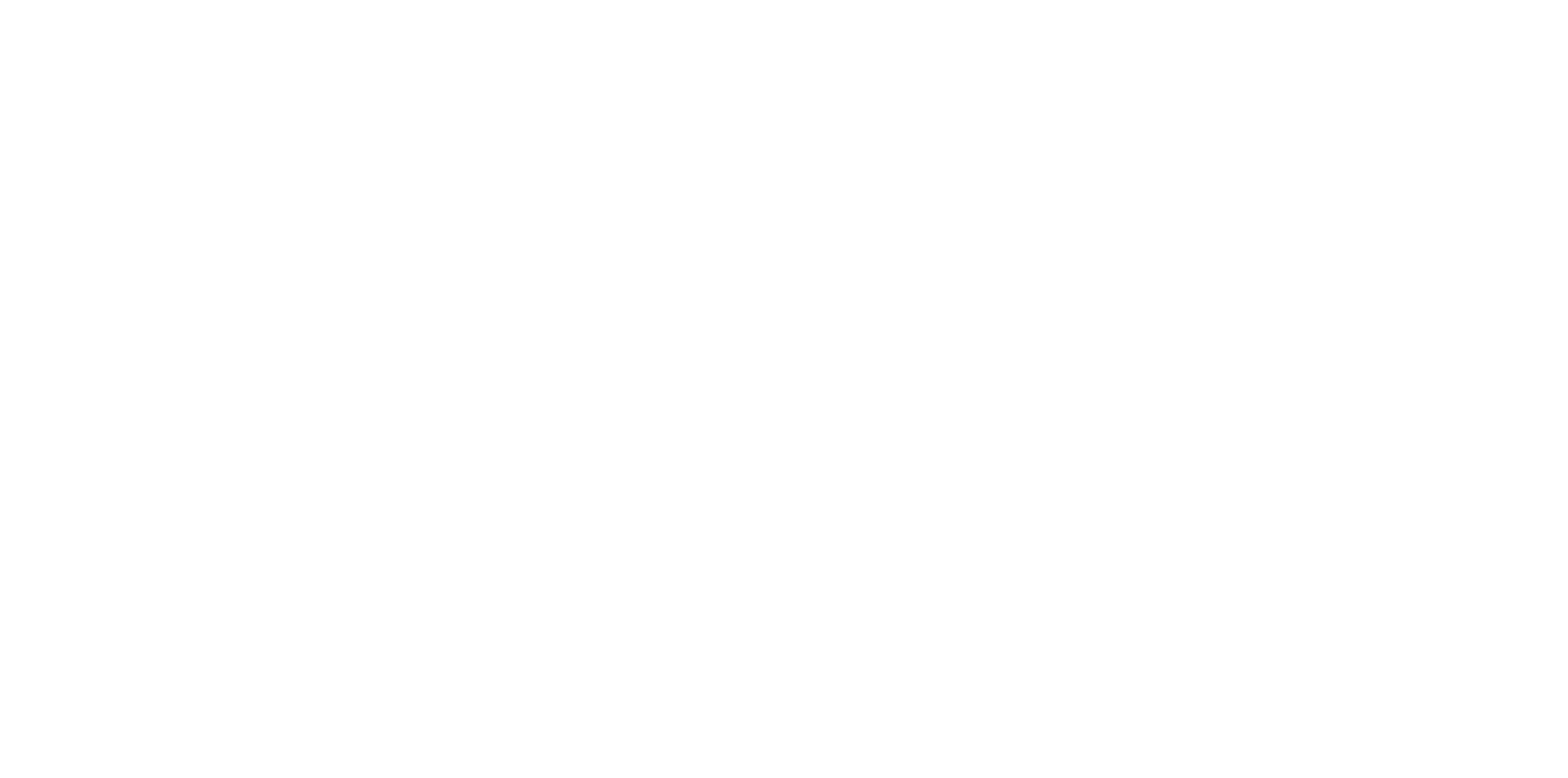
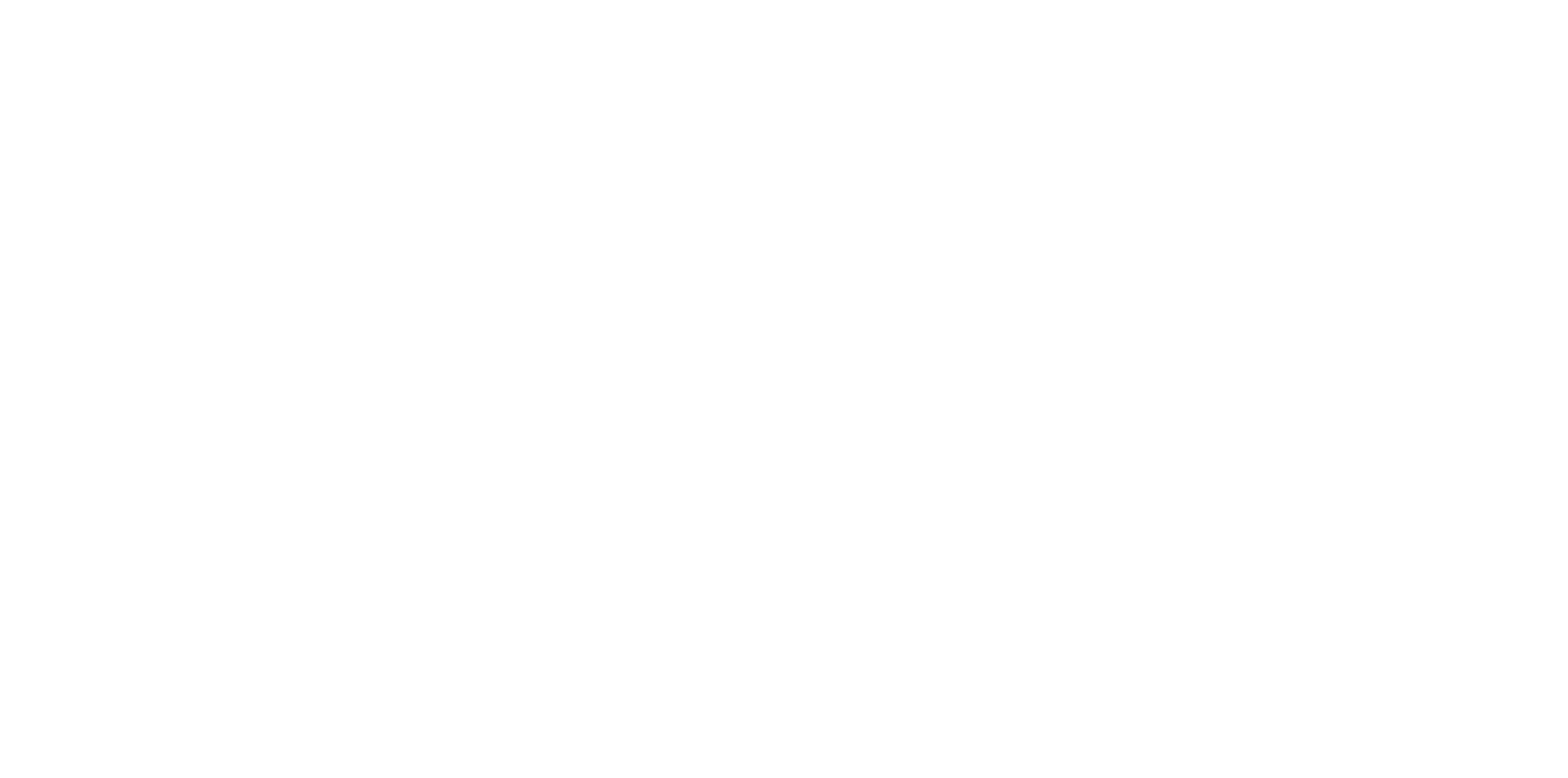







